INTRODUCTION
Although obesity has a strong association with chronic non-communicable diseases, some obese individuals did not present metabolic abnormalities, leading to a metabolic phenotype called metabolically healthy obesity (MHO). This phenotype is the result of the complex interaction between genetic, environmental, dietary, and lifestyle factors (1). The concept of MHO is not universally accepted, making difficult the interpretation of this new phenotype. One of the major challenges in MHO is its controversial definition across studies in the literature (2). While some studies consider MHO as the presence of obesity together with no more than two metabolic abnormalities, other authors consider a more conservative definition, with the absence of any major metabolic abnormality (3-6). The MHO prevalence widely varied depending on the adopted definition, ranging from 6 % to 75 % (7).
An important aspect related to the classification of metabolic phenotypes is the use of BMI for the identification of body fat excess. Although BMI is widely used in clinical practice with a reasonable correlation with body fat, it can result in individual misclassifications due to the influence of bone mass, mass muscle, and body fluids on weight. Furthermore, BMI does not adequately reflect the distribution of body fat, with waist circumference better predicting cardiovascular events than BMI in some studies (8). Despite these limitations, BMI can be easily obtained and does not require expensive equipment nor trained personnel, this being the most widely used anthropometric measurement in clinical practice with a good association with clinical outcomes and mortality (9).
Some cross-sectional studies have shown an association between intake of ultra-processed foods and outcomes such as obe- sity and metabolic syndrome (10,11). An increased risk of obesity, hypertension, and dyslipidemia among consumers of ultra-processed foods was also reported in a cohort study (12). Considering the increase in the consumption of ultra-processed foods in Brazil over the last years (13), and that MHO individuals are more likely to develop cardiometabolic abnormalities over time (14), the identification of a relationship between major food consumption by degree of processing and metabolic phenotypes could provide important information to the development of prevention programs based on eating habits. In this setting, the present study hypothesized that the metabolically unhealthy phenotypes have a higher consumption of ultra-processed food (15). Therefore, this study aimed to verify the association of food consumption according to the degree of food processing with metabolic phenotypes in workers from a quaternary hospital in Rio de Janeiro, Brazil.
MATERIALS AND METHODS
This was a cross-sectional study carried out with workers from a quaternary hospital in Rio de Janeiro, Brazil (National Institute of Cardiology [NIC]) between 2018 and 2020. The inclusion of workers from the same institution would provide a more homogeneous sample with similar access to health services, minimizing the possibility of bias. Workers aged > 18 years, of both sexes, were included. Those with underweight (BMI < 18.5 kg/m2), those on sick leave or assigned to another health unit, pregnant or lactating women, and those with incomplete information were excluded from the study. The recruitment of participants was made in the work sector, where participants were invited to participate in the study. Data collection was carried out in two different days in order to avoid spending long time during the evaluations and improve participation rates. Day 1 (D1) comprised self-reported assessment of general health status and clinical conditions, socioeconomic information, and physical activity status. On day 2 (D2), which occurred within a 30 days period from D1, a blood draw after a 12-hour fasting, anthropometric measurements, and food frequency questionnaire (FFQ) were performed.
CLASSIFICATION OF HEALTHY AND UNHEALTHY METABOLIC PHENOTYPES (OUTCOME)
The metabolic phenotypes were determined by the combination of BMI classification with the presence of any metabolic alterations considered by the International Diabetes Federation (IDF) for metabolic syndrome classification (16). Those who presented triglycerides ≥ 150mg/dL or under specific treatment for this lipid abnormality, HDL-cholesterol < 40 mg/dL (men) or < 50 mg/dL (women) or under specific treatment for this lipid abnormality, high blood pressure with systolic and/or diastolic blood pressure ≥ 130/85 mmHg or under use of antihypertensive drugs, and blood glucose ≥ 100 mg/dL or previous diagnosis of type 2 diabetes.
Thus, the groups of healthy and unhealthy metabolic phenotype were as follows: a) metabolically healthy eutrophic (MHE) -BMI 18.5 to 24.9 kg/m2 without any metabolic alteration; b) metabolically unhealthy eutrophic (MUE) -BMI 18.5 to 24.9 kg/m2 with at least one metabolic alteration; c) metabolically healthy excess weight (MHEW) -BMI ≥ 25 kg/m2 without any metabolic alteration; and d) metabolically unhealthy excess weight (MUEW) -BMI ≥ 25 kg/m2 with at least one metabolic alteration. We decided to use this more conservative definition considering the well described long-term harmful health consequences caused by any of these metabolic abnormalities. Similar definition was already used in previous manuscripts in the literature (17,18).
ANTHROPOMETRIC ASSESSMENT
The anthropometric assessment consisted of weight (kg), height (m), and waist circumference (cm). BMI was calculated dividing the weight in kilograms by the squared height in meters.
Weight (kg) was assessed using an electronic anthropometric scale (Filizola®, São Paulo, Brazil) with a maximum capacity of 180 kg and accuracy of 100 g, positioned on a flat surface. Patients were weighed barefoot and wearing light clothes (19). Height was measured in meters using a stadiometer (1-mm accuracy, Standard Sanny®, São Paulo, Brazil) coupled to a scale, with patients barefoot, head positioned in the Frankfurt position with arms extended along of the body (19).
Waist circumference (WC) was measured with an inextensible and flexible measuring tape (accuracy of 0.1 cm, Standard Sanny®, São Paulo, Brazil), with the individual in an upright position, relaxed abdomen, arms beside the body and the feet together, the measurement being taken at the midpoint between the last rib and the iliac crest (16). The cutoff points for waist circumference with the risk of metabolic complications were > 80 cm for women and > 90 cm for men (20,21).
The assessment of body composition was carried out through the octopolar multifrequency Bioelectrical Impedance (BIA) with 8 tactile electrodes from the Bioespace brand, model Inbody 720®, which operates in 6 different frequencies (1, 5, 50, 250, 500, and 1000 kHz). The measures used in the BIA were skeletal muscle mass (SMM), visceral fat area (VFA), and percentage of fat (% fat). The assessment was performed with participants fasting for at least 4 hours. To prepare for the exam, the professionals were instructed not to practice physical activity in the previous 24 hours, not to change their usual consumption of liquids, not to consume alcoholic beverages, coffee, teas, or cola-based soft drinks. Also, women of childbearing age should be out of their menstrual period. Participants were positioned on the device in an orthostatic position, with the head positioned in the Frankfurt plane, feet aligned, and positioned on the electrodes. The participants were instructed to hold the manual electrodes with arms slightly elevated at the sides of the body, maintaining the position until the end of the evaluation.
BLOOD PRESSURE
Blood pressure was measured using a digital sphygmomanometer (G-Tech®) after a 3-minute rest in the supine position with the headboard elevated at 30°. The appropriate cuff for each volunteer was selected considering the arm circumference at the midpoint between the acromion and the olecranon, and positioned 2-3 cm above the cubital fossa, on the right upper limb. Blood pressure was measured in the right upper limb, with the volunteer in the supine position and uncrossed legs (22).
BIOCHEMICAL EVALUATION
Blood samples were collected after 12 hours' overnight fasting to avoid possible biases associated with behavioral habits prior to the exam (23). Biochemical analyses were performed using the hexokinase method for blood glucose assessment and serum was analyzed by enzymatic colorimetric method for total cholesterol (TC), high-density lipoprotein (HDL-c), low-density lipoprotein (LDL-c), and triglycerides (TG), using an automated method (ARCHITECT ci8200, Abbott ARCHIECT®, Abbott Park, IL, USA) and commercial kits (Abbott ARCHITECT c8000®, Abbott Park, IL, USA).
FOOD CONSUMPTION (EXPOSURE)
Food consumption was assessed using the semiquantitative Food Frequency Questionnaire (FFQ), containing 76 items validated for the Brazilian population (24).
Participants were asked to indicate the frequency (> 3 times/day; 2-3 times/day; 1 time/day; 5-6 times/week; 2-4 times/week, 1 time/week; 1-3 times/week; 1 time/month; never or almost never) and the average amount of consumption for the last 12 months. The frequency of food consumption reported by the participants was transformed into daily frequency. The food consumed had the home measures converted to grammage using the table for assessing food consumption into home measures (25), and then the energy value was quantified using the table of the Brazilian Institute of Geography and Statistics (IBGE) (26).
To classify foods according to their degree of processing, the NOVA classification, proposed by Monteiro et al. was used (27). Foods and culinary preparations present in the FFQ were categorized according to the degree of processing into 3 groups (instead of four, according to the NOVA classification). Processed culinary ingredients were grouped to unprocessed or minimally processed foods because these ingredients are obtained directly from originally unprocessed or minimally processed foods or from nature by processes such as pressing, refining, grinding, milling, and spray drying, being rarely consumed alone. Additionally, the use of the FFQ has limitations to classify some items according to the degree of processing due to the low level of detail of the information, when compared to other methods. For this reason, we included culinary ingredients based on fresh or minimally processed foods, as well as culinary preparations with unprocessed or minimally processed foods, as previously suggested (13,28,29). Group 2 includes processed foods and group 3 included ultra-processed foods (13,28,29). Because they constitute very rare events, cases with energy consumption above 6,000 kcal or less than 500 kcal were excluded (30,31).
COVARIATES
The covariates age, sex, income, schooling (elementary school, high school, and college), smoking, and presence of comorbidities were self-reported. Physical activity (PA) level was assessed using the International Physical Activity Level Questionnaire short form (IPAQ-short) (32). The IPAQ-short measures the global physical activity level performed at work, leisure, means of transport, and household activities. In addition, the seated time spent on a typical weekday and a typical weekend day was also questioned. The IPAQ-short categorizes individuals as high, moderate, and low PA levels.
STATISTICAL METHODS
Statistical analyses were performed using the SPSS 23 statistical software. Data distribution was evaluated using the Kolmogorov-Smirnoff test. Data were presented as mean and standard deviation or median and interquartile interval. Variables were compared using the Kruskal-Wallis test followed by the Dunn post-test. The association between consumption of ultra-processed foods and metabolic phenotype were assessed using Stata version 13.0 using multinomial regression models adjusted for sex, age, education, and physical activity, and a confidence interval of 95 % was considered. Participants with missing information for exposure or outcome variables were excluded from the analysis. Values of p < 0.05 were considered significant for all analyses.
Sample size was calculated considering a 95 % confidence level (α) and 80 % statistical power (β). An effect size of 0.7 was considered for mean differences across the 4 groups of metabolic phenotypes, which determined the total sample size of 112 participants (28 in each group).
ETHICAL ASPECTS
This study was conducted in accordance with Helsinki Declaration amended in 2013 and was approved by the Scientific Committee/Research Ethics Committee — CEP of the National Institute of Cardiology under number 2.849.484 and CAAE: 96222718.7.0000.5272 approved on August 28, 2018. All participants signed an informed consent form.
RESULTS
Of the 230 eligible participants, 20 were excluded according to the predefined exclusion criteria, 9 did not return to D2 assessments, and 41 were excluded due to missing information for the outcome variable. Therefore, 160 individuals were included in the analysis, as demonstrated in figure 1.
The mean age of participants was 45.2 ± 11.1 years and 59.4 % were women. Most had higher schooling and family income was between 4 and 10 minimum wages for the majority. The categories of PA levels were almost equally distributed, with a slightly greater proportion for high PA level (35.6 %). Smokers accounted for 11.9 %. The self-reported frequency of comorbidities was 21.9 % for arterial hypertension and 4.4 % for diabetes. Most presented excess weight (74.6 %), with approximately 40 % being obese (Table I).
Figure 2 shows the frequency of metabolic phenotypes, with the majority of participants being classified as MUEW (55 %).
There are some significant differences between metabolic phenotypes for anthropometric and body composition parameters. Overall, the MUEW phenotype had a higher body fat percentage and central adiposity represented by higher WC and VFA in comparison to the other phenotypes. The lean body mass was similar between the groups (Table II).
Table II. Anthropometric parameters and body composition according to metabolic phenotype.
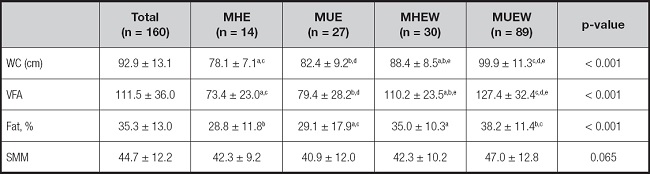
Mean (SD); WC: waist circumference; VFA: visceral fat area; % fat: percentage of body fat; SMM: skeletal muscle mass.
MHE: metabolically healthy eutrophic; MUE: metabolically unhealthy eutrophic; MHEW: metabolically healthy excess weight; MUEW: metabolically unhealthy excess weight.
Kruskal Wallis test and Dunn post-test. Equal letters represent statistically significant differences (p < 0.05) for the same variable.
Table III depicts biochemical characteristics and blood pressure according to metabolic phenotype groups. Healthy phenotypes, regardless of BMI, have higher HDL-c than the MHEW phenotype. Blood pressure was lower for MHE in comparison to MUE and MUEW.
Table III. Biochemical characteristics and blood pressure according to metabolic phenotype.
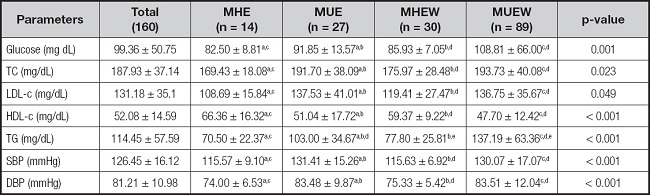
Mean (SD); TC: total cholesterol; LDL-c: low density lipoprotein cholesterol; HDL-c: high density lipoprotein cholesterol; SBP: systolic blood pressure; DBP: diastolic blood pressure.
MHE: metabolically healthy eutrophic; MUE: metabolically unhealthy eutrophic; MHEW: metabolically healthy excess weight; MUEW: metabolically unhealthy excess weight.
ANOVA test and Dunn post-test. Equal letters represent a significant difference.
There was no statistically significant difference for energy consumption according to the BMI groups, with an elevated contribution of ultra-processed foods among participants included in the study, as shown in table IV.
Table IV. Percentage of energy contribution of food consumption groups according to BMI classification.
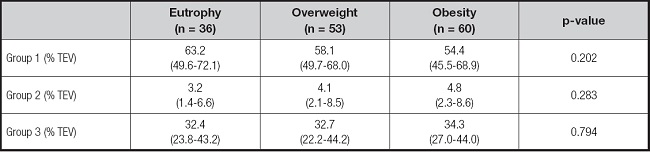
Median (interquartile interval). TEV: total energy value; Group 1: food in natura or minimally processed, or culinary preparations based on these foods; Group 2: processed foods; Group 3: ultra-processed foods. Kruskal-Wallis test.
The association between food consumption by degree of processing with metabolic phenotype is depicted in table V, with no statistically significant association being observed.
Table V. Association of food consumption groups with metabolic phenotypes (n = 160).
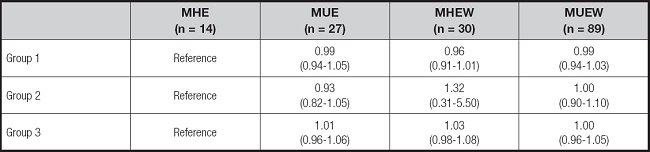
RRR (95 % CI). Multinominal regression adjusted for sex, age, education and level of physical activity; Group 1: food in natura or minimally processed, or culinary preparations based on these foods; Group 2: processed foods; Group 3: ultra-processed foods; MHE: metabolically healthy eutrophic; MUE: metabolically unhealthy eutrophic; MHEW: metabolically healthy excess weight; MUEW: metabolically unhealthy excess weight.
DISCUSSION
Ultra-processed foods and drinks are enhanced with large amounts of salt, added sugar, and fat, as well as the use of additives in an attempt to make this food category highly palatable (33). These foods are, on average, more hypercaloric and less satiating than minimally processed foods and culinary preparations based on minimally processed foods (34). In contrast, processed foods are whole foods preserved by traditional techniques such as canning, pickling, smoking, curing, alcoholic and non-alcoholic fermentation (35). The biological pathways through which ultra-processed foods influence metabolic health may involve complex mechanisms and synergies between many compounds and characteristics of ultra-processed foods, which are not yet fully understood (36). Key mechanisms include altered serum lipid concentrations, modified gut microbiota and host-microbiota interactions, obesity, inflammation, oxidative stress, dysglycemia, insulin resistance, and hypertension and hormonal imbalances (36). Contrary to our hypothesis, the present study did not find any significant association between food consumption by degree of processing with metabolic phenotype. The comparison of our results with a Brazilian population study that also included workers (the ELSA study), demonstrated a lower percentage of high blood pressure and diabetes but with a reversal frequency of metabolic phenotypes, in which less than 10 % are MHE compared to 55 % for MUEW. In the ELSA study, when obese and overweight phenotypes were combined, 39 % were MUEW and 31 % were MHE (37).
As expected, adiposity-related parameters were higher in individuals with MUEW. This characteristic is already well recognized and was also found in a study carried out in Chile, that observed a higher BMI in metabolically unhealthy individuals than in healthy individuals, reinforcing the importance of adipose-related parameters on the pathophysiology of metabolic abnormalities (38). However, comparisons between studies are difficult since the classification of metabolic phenotypes may substantially differ across studies (2).
Factors associated with a metabolically healthy phenotype should be considered as they can exert a protective effect on overweight individuals, which influences the parameters adopted to classify the phenotype. A Russian study found that MHO was associated with younger age, lower WC, higher level of physical activity, and shorter duration of obesity (39).
The groups with healthy phenotype, regardless of BMI, had a worse lipid profile than unhealthy phenotypes. Factors associated with inflammation and atherogenic dyslipidemia reinforce that the unhealthy phenotype had lower HDL-c, which corroborates the importance of visceral fat but not BMI for the development of metabolic abnormalities.
Parameters related to adiposity observed in the body composition and biochemistry of the studied group are found in the pathophysiological mechanisms presented in obesity, as well as the relationship of excess weight with components of metabolic syndrome (abdominal obesity, low HDL-c, high triglycerides, hyperglycemia, and hypertension). In obese individuals, excess adipose tissue causes low-grade chronic inflammation. Adipocytes produce adipocytokines such as TNFα and IL-6. During low-grade systemic inflammation, insulin-dependent tissues are exposed to infiltration of macrophages that promote inflammation and affect tissue performance in response to insulin (40).
Considering the fat distribution across groups, the higher central adiposity already expected in excess weight reinforces that central is more atherogenic than peripheral fat. In one Mexican study, WC was used as a criterion to classify phenotype and identified women with the metabolically unhealthy phenotype in all BMI categories. This suggests that not only the amount of body fat is an important factor in the development of metabolic complications, but also how the fat is distributed (41).
Evidence also shows that individuals with normal BMI but excessive fat percentage tend to develop various metabolic diseases as observed in the study by Yi-Chien Lu et al., in which a high-fat percentage was associated with risk of metabolic syndrome despite a normal BMI (42).
The present study did not demonstrate any significant differences in food consumption by degree of processing according to BMI, despite the fact that other studies have demonstrated that an increased consumption of ultra-processed foods is a factor that contributes to weight gain. In a large prospective cohort, participants who consumed more ultra-processed foods in France tended to have a greater increase in BMI during follow-up, and increased their risk of becoming overweight and obese, regardless of their baseline BMI. In this study, these associations remained statistically significant after adjusting for a wide range of socioeconomic and lifestyle factors, and after additional adjustments for several indicators of the nutritional quality of the diet (43).
It is important to consider that the definition of metabolic phenotypes widely varies across studies, which makes comparisons between their results difficult. The present study used the BMI classification together with the absence or presence of metabolic abnormalities according to IDF thresholds (16), considering as unhealthy phenotype the presence of a single metabolic abnormality.
Different methods of evaluating consumption are carried out to investigate associations with phenotypes. A cross-sectional study conducted in an adult population of Tehran, in comparison with metabolically healthy obese people, when there was greater adherence to the DASH diet, the results associated a 21 % less chance of having metabolically unhealthy obese people, regardless of age, sex, energy intake, physical activity, BMI, smoking and educational level (44). In an Australian study, it was identified that 20 % of adults aged ≥ 45 years were metabolically healthy obese and that higher consumption of an unhealthy dietary pattern was associated with a decreased probability of having a healthy metabolic and BMI profile, while the opposite was apparent for the healthy eating pattern (45).
Unlike the findings in this study, some studies investigating exposure to ultra-processed products and adverse health outcomes such as overweight, obesity, or cardiometabolic risks showed associations (46). However, it is observed that there is still a need for further studies to associate the consumption of ultra-processed food with negative effects on health, and that perhaps the consumption of minimally processed foods can provide more information since this association is not found. A study in a nationally representative sample in the United Kingdom showed no associations of ultra-processed foods with body weight parameters, although the consumption of minimally processed food was associated with a lower chance of being overweight and obese (47). Likewise, in a study that showed no significant association with ultra-processed food and metabolic syndrome, there was a lower chance of metabolic syndrome, low HDL-c, and hyperglycemia with a higher intake of minimally processed food. Both studies provide support for minimally processed rather than ultra-processed diets (48).
The present study has some limitations as the method of assessing metabolic phenotypes is a widely discussed issue in several studies (49,2). There is a consensus on the urgency of a single definition that characterizes studies with metabolic phenotypes so that aspects that relate to diseases and modifiable aspects of health can be more clearly determined. Moreover, the self-reported assessment of general health status and clinical conditions may have provided underestimated frequencies of major comorbidities such as hypertension and diabetes. On the other hand, blood pressure and glucose were measured to determine the metabolic profile, eliminating the potential influence of self-reported measures on the determination of the metabolic profile. Finally, we cannot exclude the possibility of reverse causation due to the cross-sectional design, in which those participants with a previously diagnosed cardiometabolic disease were previously advised to decrease their consumption of ultra-processed foods.
To conclude, the present study did not observe any association between metabolic phenotypes and the consumption of food by degree of processing according to the NOVA classification. However, an important consumption of ultra-processed foods was observed, reinforcing the need for public health strategies that improve food consumption.