Introduction
Teacher resilience can be defined as the ability to bounce back from adverse situations that occur both in the academic context and in one's personal life (Benvenuto et al., 2021). This concept encompasses the interaction of both occupational factors and socio-demographic variables. (Ainsworth & Oldfiedl, 2019). In addition, in the educational field, teachers must face a complex reality and multiple scenarios (Valentino & Sosnowski, 2019). The ability to cope with all these problems in a resilient manner appears to be a key factor in the prevention of work-related stress (Hlaďo et al., 2020). Among other factors, gender could be involved in the development of resilient behaviours. Thus, Rodríguez-Donaire et al. (2020) found higher mean scores for women than for men among teachers. In the same vein, Yada et al. (2021) showed that women were more resilient than men.
According to the demand-control model of occupational stress, the loss of a balance between what the environment demands of teachers and what they can provide and control leads to a situation of occupational stress that can have dramatic consequences (Marić et al., 2020). From the stress generated in an environment whose demands exceed resources comes emotional exhaustion, depersonalisation and low professional fulfilment that define the syndrome (Szempruch, 2018). Teachers with greater resilience resources are less vulnerable to feeling emotionally exhausted or experiencing stress or burnout (Llorca-Pellicer et al., 2021; Polat & İskender, 2018). This type of teacher has a more positive mindset and bounces back easily from adversity while teachers with lower levels of resilience show a more negative attitude, less resilience, and a higher risk of suffering from the syndrome (Vicente de Vera & Gabari, 2019).
In this sense, teaching is a stressful profession in which there is a tendency to suffer from burnout syndrome, with teachers being a vulnerable group (Altuntaş & Genç, 2020; Candeias et al., 2021; Carmona & Muñoz, 2021; Roohani & Iravani, 2020). Burnout syndrome in teachers arises after being subjected to a chronic situation of stress at work in which the person does not have sufficient resources to be able to manage the high and prolonged emotional and psychosocial demands (Carlotto & Câmara, 2017). Thus, a previous study found that 22.5% had high levels of emotional exhaustion, 22.5% had high levels of depersonalisation and 23.8% had low levels of personal self-fulfilment (Carmona & Muñoz, 2021). Other research found a relatively similar trend, with approximately 25% of teachers reporting high levels of emotional exhaustion, 18% depersonalisation and 17% low professional fulfilment (Seibt & Kreuzfeld, 2021). In Roohani and Iravani's (2020) research, the mean for depersonalisation was in the top third (≥ 13) and the same was true for lack of personal fulfilment (≥ 31). This trifactorial structure is supported by a number of researchers (Diat Prasojo et al., 2020). With regard to the genesis, emotional exhaustion may arise even before the start of the job itself, i.e. during teacher training (Väisänen et al., 2018), hence the importance of early detection.
With regard to the relationship between the syndrome and the gender perspective, the results are contradictory, which justifies the need for further research. Thus, on the one hand, Guan (2020) found that emotional exhaustion was higher in female teachers, in people aged between 31 and 60 years and in those who had 12 or fewer teaching hours per week. In another study also conducted with teachers, female teachers were found to have higher levels of emotional exhaustion, while male teachers showed higher depersonalisation and lower professional fulfilment, although the effects of gender were low and therefore the data need to be interpreted with caution (Yorulmaz & Altinkurt, 2018). This contrasts with another study which found no significant differences between women and men in burnout (Kroupis et al., 2017); were also not found by Feld (2021) and Seibt & Kreuz (2021), and further research is needed. With respect to other socio-demographic variables, marital status also seems to influence levels of stress and burnout, with married teachers scoring higher on professional fulfilment than divorced, widowed and single teachers (Arias et al., 2019). It seems that, while socio-demographic variables may shed light on the issue, their contribution to explaining how stress is managed in teachers is less than that of the burnout dimensions and working conditions themselves (Marić et al., 2020). After COVID-19, teachers continued to report high levels of stress and burnout, with women being the group with the highest scores and older and more experienced teachers having the lowest levels (Sánchez-Pujalte et al., 2021).
As can be inferred, resilience helps teachers to keep their stress levels in check and to be able to manage complex problems (Platsidou & Daniilidou, 2021). In other words, it seems that the relationship between burnout syndrome and resilience is inverse (Polat & İskender, 2018). Burnout syndrome can be explained, in part, by the absence of resilience, which implies emotional intelligence and the ability to develop intrinsic motivation (Abdul et al., 2020). In particular, an inverse relationship was found between resilience and emotional exhaustion such that those teachers who were more resilient showed lower levels of this dimension of burnout (Valosek et al., 2021).
Resilience building has been shown to be effective in preventing stress (Szempruch, 2018). Thus, the implementation of functional, adaptive and proactive strategies by teachers to cope with burnout has been shown to be very useful, especially in the early stages of teacher training to reduce levels of stress and burnout (Väisänen et al., 2018). Instruction and implementation of strategies based on positive beliefs and affectivity has also been associated with lower stress levels (Karakus et al., 2021).
With respect to resilience and COVID-19, the pandemic does not appear to have significantly reduced resilience levels in secondary school teachers (Liu et al., 2021). In the same study, it was also found that low levels of resilience were predictive of high levels of emotional exhaustion, so COVID-19 has not changed the relationship between the two concepts.
Predicting resilience levels in the educational community can be done through technological and methodological advances in the field of artificial intelligence (AI). AI in education has been shown to be effective in emulating the human decision-making process and reducing the resources needed to do so (Colchester et al., 2016). AI makes it possible to mine the information provided by the questionnaires by analyzing the relationships of their variables with each other (Guo et al., 2021). Despite this, AI remains an unfamiliar technology for teachers, although it is progressing very fast (Luan et al., 2020). In fact, its impact on society is growing all the time (Dignum, 2021; Paek & Kim, 2021). For progress to be made, it is necessary to change methodological aspects, issues related to research and, of course, education policy (Hwang et al., 2020). In this sense, there is a growing interest in AIEd studies which can be easily inferred from the number of scientific contributions in this field (Chen et al., 2020a, 2020b). Artificial intelligence in education (AIEd) allows the automatization of various processes in a way that frees up time, cognitive resources, etc. for teachers who can use this to perform other functions (Schiff, 2021). IAEd and associated algorithms must be diversified in order to be able to study as many educational phenomena as possible (Paek & Kim, 2021). The application of methodologies based on this technology makes it possible to simulate educational conditions and predict their results (Bañeres et al., 2020; Chen et al., 2020a, 2020b; Guo et al., 2021; Pokrivcakova, 2019). The combined use of descriptive analytics and artificial intelligence-based predictive algorithms has proven effective in the field of education to draw relevant conclusions (Bozkurt et al., 2021). Furthermore, the backpropagation algorithm used in the artificial neural network architecture has yielded very positive results (Buddhtha et al., 2019). The conclusions drawn through the use of this methodology allow for adaptation to educational agents to a greater extent, taking into account their preferences and needs in the teaching-learning process in a multidimensional way (Goksel & Bozkurt, 2019), as it allows for the development of the principle of individualisation and personalisation of teaching (Reiss, 2021).
That said, the overall aim of this research was to predict current levels of resilience in secondary school teachers as a function of gender using artificial neural networks. The specific objectives that were established were as follows: (1) to find out the level of teacher resilience in general terms and as a function of gender; (2) to examine the levels of emotional exhaustion, cynicism and professional efficacy (dimensions of burnout) in male and female teachers; (3) to study the level of stress associated with COVID-19 in teachers as a function of gender; (4) to develop artificial neural networks (ANN) that predict resilience based on scores on the dimensions of burnout, stress associated with COVID-19 and socio-demographic variables.
Method
Participants
The research involved 401 teachers who taught in secondary schools in south-eastern Spain. 29.4% (N = 118) were male teachers and 70.6% (N = 283) were female teachers. Regarding the total sample: the mean age of the total sample was 44.36 years (SD = 9.38); the years of experience was M = 15.38 (SD = 10.10); regarding marital status, 51. 1% (N = 205) were married, 20.7% (N = 83) were in a couple, 19.2% (N = 77) were single, 8% (N = 32) were divorced and 1% (N = 4) were widowed; and regarding the existence of offspring, 61.8% (N = 248) had daughters and/or sons. As for the female teachers: the mean age of the female teachers was 43.72 years (SD = 9.73); teaching experience was at M = 14.95 (SD = 9.99), with respect to marital status, 48. 8% (N = 138) were married, 20.8% (N = 59) had a stable partner, 20.1% (N = 57) were single, 8.8% (N = 25) were divorced and 1.4% (N = 4) were widowed; finally, 60.1% (N = 170) had children and/or daughters. Regarding the teachers: the mean age was 45.91 years (SD = 8.33); the mean number of years of experience was 16.41 (SD = 10.32); regarding the marital status of the teachers, 56.8% (N = 67) were married, 20.3% (N = 24) were in a couple, 16.9% (N = 20) were single and 5.9% (N = 7) were divorced; finally, 66.1% (N = 78) had daughters and/or sons. There were no significant differences in socio-demographic variables between women and men (p > .05).
Instruments
Regarding the evaluation instruments, the following were used:
Brief Resilience Coping Scale (BRCS) by Sinclair & Wallston (2004) being the Spanish adaptation of Moret-Tatay et al. (2015). It is an instrument that assesses the level of resilience through 4 items and 5 response options where 1 means that it is not a typical response and 5 means that it is a habitual response. Example item: "Regardless of what happens to me, I believe I can control my reaction to it” (item 2). The sum results in a direct score that can be categorized into three levels (low resilience < 13; medium resilience between 14 and 16; high resilience ≥ 17). This scale obtained a Cronbach's Alpha of 0.73.
Maslach Burnout Inventory - General Survey (MBI-GS) created by Maslach & Jackson (1986) and validated in Spanish by Salanova et al. (2000). It consists of 22 items rated on a Likert-type scale with seven response options ranging from 0 meaning never to 6 meaning always or every day. Its factor structure reflects the existence of three dimensions: emotional exhaustion (α = 0.9), cynicism (α = 0.79) and professional efficacy (α = 0.71). Example of an emotional exhaustion item: “Working all day is a strain for me.”. Example of a cynicism item: “I have lost enthusiasm for my work”. Example of a professional effectiveness item: “I have achieved many valuable things in this position”.
Stress Evaluation Questionnaire for COVID-19 (SEQ-COVID-19) de Martínez-Ramón et al. (2021). It is a questionnaire to assess the stress of being involved in a series of situations related to COVID-19 and collects socio-demographic variables such as age, gender, years of experience and marital status). The questionnaire has been used in the Spanish teaching population and consists of 6 items assessed through a Likert-type scale of 10 options in which 1 means that it does not generate stress and 10 means that it generates a lot of stress. The sum of all items results in a variable called "Stress about COVID-19". An example item is to assess the level of stress generated by the thought of "a member of your family becoming infected with the virus". In addition to this variable, it includes the potential stress of being infected by oneself, a friend, the student body, a co-worker, or a family member in the student community.
Procedure
The research was quantitative, cross-sectional, and ex post facto. Seventy-three secondary schools in southeastern Spain were contacted and the objective and procedure of the evaluation were explained. Once the school agreed, the questionnaires were administered to the teachers with their prior consent. Data were collected in the first quarter of 2021. Teachers were informed of the voluntary nature of their participation in the study, as well as of the confidentiality and anonymity of the data. The criteria set out in the Declaration of Helsinki and by a university ethics committee were followed.
Regarding data analysis, a descriptive analysis of the main indices of dispersion and central tendency was carried out. An inferential analysis was also carried out. This analysis consisted of the study of significant differences between two independent groups (women and men) using the Student's t-test and Chi-Square.
Within the inferential analysis, three artificial neural networks (ANN1, ANN2, and ANN3) were established based on a backward propagation model and three layers (input, hidden and output) to form a multilayer perceptron network. First, a seed with the fixed value 9191972 was set to establish a starting point in the initiation process of the active random number generator of the statistical package SPSS version 24. The cases were distributed so that the approximate proportion chosen by the network tended to be 60% for the training phase, 30% for the testing phase and the remaining 10% to perform a predictive analysis of new cases not used for the elaboration of the algorithm developed in the training phase and optimized in the testing phase.
Results
The results obtained when analysing the gender differences between the means in resilience (objective 1), with the burnout dimensions (objective 2), with the stress generated by COVID-19 (objective 3) and the main socio-demographic variables, can be found in Table 1, having applied Student's t-test. As can be seen, differences are found in the burnout dimension of efficacy (professional fulfilment) (t = .095, p = .041) and COVID-19 (t = .038, p = .000), with the means being higher in women in both variables.
Table 1. Differences between means on resilience, burnout dimensions, COVID-19 stress and the continuous sociodemographic variable experience.

Source: Own elaboration.
Note.M: Media; SD: Standard Deviation; t: Student's t-test; p: Level of significance.
With regard to the design of the artificial neural networks, three were created: one of a global nature for all the participants (RNA-Global), another for the female teachers (RNA-2), and another for the male teachers (RNA-3). The main information of the network is set out in Table 2.
Table 2. Information from the network regarding RNA-1, RNA-2, and RNA-3.
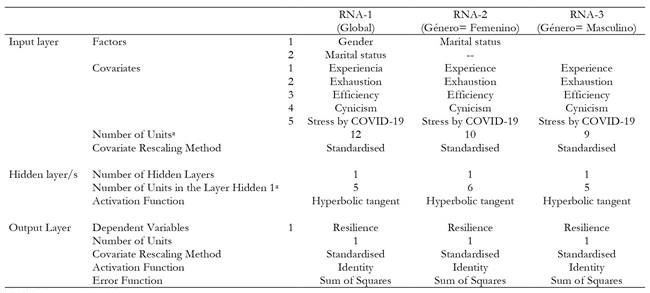
Note.a: Excluding bias units.
Figures 1, 2, and 3 show the RNA-1, RNA-2, and RNA-3 networks respectively, with resilience as the dependent variable in all three cases. Synaptic weights are represented with thicker lines are those below 0 and synaptic weights with thinner lines represent values above 0.

Source: Own elaboration.
Figure 2. Artificial neural network predictor of resilience in women ANN-2.
The summary of the three models for each of the phases involved in the design is set out in Table 3.
Table 3. Summary of RNA-1, RNA-2, and RNA-3 models.

Source: Own elaboration.
Note.ARN-1: Global Artificial Neural Network; ARN-2: Artificial Neural Network in females; ARN-3: Artificial Neural Network in males; a: Error estimates are based on the test sample; Dependent variable: Resilience.
The contribution of each of the variables to the predictive capacity of the levels of self-esteem in teachers is shown in Table 4 for each of the three artificial neural networks designed. It can be seen that professional efficacy or professional achievement is the variable that contributes most to predicting the levels of the dependent variable self-esteem, while the socio-demographic variables make a smaller contribution to the model.
Table 4. Importance and normalized importance of independent variables.
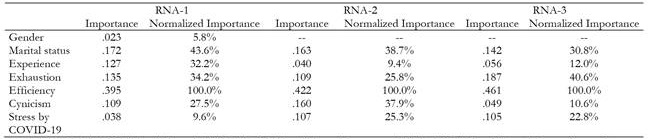
Source: Own elaboration.
Note.ANN-1: Global Artificial Neural Network; ANN-2: Artificial Neural Network in women; ANN-3: Artificial Neural Network in men.
Finally, Figures 4, 5 and 6 show the relationship between the values predicted by the model and those observed in resilience for RNA-1, RNA-2, and RNA-3 respectively. It is possible to observe in the three networks a directly proportional relationship with a pattern of points ascending and oblique to the coordinate axes.

Source: Own elaboration.
Figure 5. Relationship between the value predicted by the model and that observed in ANN-2 in female teachers.
Discussion
The general objective of the study was to predict the resilience shown by teachers as a function of gender through the ANN design. With respect to objective 1 related to knowing the levels of resilience between women and men, it was found that women and men obtained similar means in contrast to the findings of Rodríguez-Donaire et al. (2020) and Yada et al. (2021). This may be because both work in the same environment, the demands are similar and the strategies put in place to cope with the challenges are similar. This needs to be studied further.
In relation to objective 2, significant differences have been observed between women and men in burnout and efficacy. In the present study, women obtained higher mean scores on these dimensions. However, no significant differences were found in cynicism. Partly coinciding with the current results, in a previous research, higher burnout scores were obtained in women (Sánchez-Pujalte et al., 2021). It is also in line with Arias et al. (2019) who found higher levels of emotional exhaustion in female teachers, yet equal in the rest of the dimensions. In contrast, Roohani & Iravani (2020) concluded that it was male teachers who showed higher levels of the syndrome. Thus, it was found that men showed higher scores on emotional exhaustion while women scored higher on professional fulfilment. On the other hand, the research of Seibt & Kreuzfeld (2021), who found no gender differences in burnout dimensions, is noteworthy. The behaviour of the burnout dimensions seems to operate relatively autonomously. In this respect, research by Marić et al. (2020) showed that teachers can sometimes have low professional fulfilment without high levels of emotional exhaustion and depersonalisation.
About objective 3, which focused on the study of COVID stress by gender, women obtained higher means on the instrument to measure COVID stress-19. In a study of teachers at the time of COVID-19, it was found that women scored higher on stress than men (Sánchez-Pujalte et al., 2021). Based on the demand-control theory of work-related stress, it is possible that the key is to deal with work overload. To this end, it is proposed to reduce the number of classes taught per teacher, to train teachers in skills for interaction with the educational community, as well as time for subject preparation, among other measures (Seibt & Kreuzfeld, 2021).
Finally, with respect to objective 4, a different contribution of the variables under analysis to the predictive capacity of the network was found according to gender. It is recalled that the aim was to predict the levels of resilience through the dimensions of burnout, stress associated with COVID and certain socio-demographic variables. In the ANN in women, the three independent variables that contributed most to the predictive ability of the network were efficiency, marital status, and cynicism. The inclusion of variables of different natures and covering both environmental and personal factors enriches the model (Seibt & Kreuzfeld, 2021). In the case of men, these variables were efficiency, marital status and stress associated with COVID-19. The algorithm used for the neural architecture was backpropagation, which has given good results in previous studies (Buddhtha et al., 2019). Furthermore, the use of descriptive and intelligent analysis provides a great opportunity to extract valuable and mutually compatible data (Bozkurt et al., 2021; Guo et al., 2021). The AI underlying the analyses in this research is innovative (Dignum, 2021), and does not appear to be behaving as a fad but is being established in the system itself with the intention of continuity and evolution (Reiss, 2021). Furthermore, following Dignum (2021), AI is more than the sum of its parts. More specifically, AI is more than the algorithm itself that is generated, but is implicitly a reflection of the socio-cultural context.
Applicability and implications of the use of AI in education
Low resilience may be present in teachers' dropout from teaching (Valentino & Sosnowski, 2019). For this reason, designing programmes that promote resilient coping strategies could help to increase the ability to overcome adverse situations. Resilience intervention can be helpful in managing stress caused by COVID-19 (Matiz et al., 2020). Going even further, it can be inferred that fostering teacher resilience could reduce other associated problems such as emotional exhaustion, cynicism, and low professional fulfilment. In this sense, teacher training in mental health to prevent burnout syndrome should be a priority strategy (Liu et al., 2021; Yang, 2019), respecting the professional competences of each field. Teachers must learn to manage the problems of their own profession and develop strategies to cope with adversity (Altuntaş & Genç, 2020). Teaching teachers' strategies and behaviors that improve their relationships, autonomy and support will help to increase their ability to manage the demands of the environment in an adaptive way (Llorca-Pellicer et al., 2021). It is also necessary to teach emotional competences to reduce levels of stress and burnout (Sánchez-Pujalte et al., 2021). To this end, cognitive-behavioral programs for secondary school teachers have been shown to be effective in reducing the levels of the dimensions associated with burnout (Carmona & Muñoz, 2021).
The teacher capable of using artificial intelligence in education is called an "intelligent tutor". This is a teacher who can assess the main variables that influence the teaching-learning process of his or her students, carrying out intelligent data analysis and anticipating the results (Hwang et al., 2020). To achieve this, it is necessary to promote IL by training the educational community (Goksel & Bozkurt, 2019), including the different educational agents that intervene in the classroom directly and indirectly, such as the tutor, the student, co-workers, educational advisors, etc. (Hwang et al., 2020). This AIEd is also under development and its implications for factor identification and assessment are profound (Paek & Kim, 2021).
Understanding the functioning of resilience in the educational community will help to develop adaptive strategies for a multitude of stressful situations including those of environmental origin such as climate emergencies analyzed through "eco-resilience" (Gallay et al., 2022). This study makes a preliminary contribution to the field by showing the reaction of a collective in a potentially hostile environment beyond its control (in a scenario influenced by the COVID-19 pandemic), which is a vital element that can be extrapolated to future challenges.
In the coming years, it will be necessary to reflect and define what the objectives of AI are and whether they are in line with human values (Dignum, 2021). AI could be used in placing students in the most appropriate programs, for use in teacher selection processes, in making decisions about which resources are most useful, etc. Reiss (2021). It is also expected that this technology can help predict what resources the education system needs to organize and manage to meet future challenges and thus optimize time and effort. On the negative side, AI could lead to a decrease in the number of teachers required (Reiss, 2021), which is why it is necessary to use such technology in an ethical and rational way (Schiff, 2021) and it is warned about the gap between traditional and digital teaching and the collaboration of teachers is absolutely necessary to bridge the gap and improve student learning through AIEd.
Limitations and future lines of research
In terms of limitations, this is a cross-sectional study that obtains information at a given point in time and cannot obtain the same results as in a longitudinal design. Similarly, the sample size makes it necessary to take the results with caution, although intelligent analysis allows extrapolation of information given a modest database in terms of size. Also, the teaching profession is defined by a number of organisational characteristics that are different from the performance of other occupations, so extrapolation of findings from the teaching profession to other professions needs to be taken with caution (Kim, 2019).
With regard to future lines of research and with a view to expanding the number of variables that could be influencing the results, it is considered interesting to consider: the teacher-student ratio, as it seems that it may be mediating the emotional state of the teacher (Altuntaş and Genç, 2020). It would also be interesting for future studies: to analyse the differences in burnout between the public and private sectors (Kroupis et al., 2017), to study the differences between different professional profiles (specialists, ordinary teachers, etc.) (Candeias et al., 2021); how to manage teachers' emotions (Sánchez-Pujalte et al., 2021; Tsang et al., 2021); or to analyse the levels of involvement in teaching tasks (Salmela-Aro et al., 2019).
In conclusion, the design of artificial neural networks showed that variables such as cynicism contribute to different degrees to the predictive capacity of resilience, with a greater share for women. Burnout, on the other hand, contributes more to resilience scores for men. The fact that there may be differences between women and men suggests that the gender perspective should be considered when designing research in this area as well as for prevention through more tailored intervention programs (Roohani & Iravani, 2020).