Introduction
The expansion of higher education in most western countries resulted in higher levels of ethnic and socioeconomic diversity in the student population. This heterogeneity, also observed in students' scientific and academic preparation and ability, study habits, and motivations, is related to variability in first-year outcomes, such as academic performance and persistence. Although universities are nowadays welcoming a wider public, not all students successfully cope with the academic and psychosocial challenges related to the transition to higher education. Research has shown that the difficulties students experience in adapting to life at university are a result of an interaction between the students' characteristics and his/her academic environment (Crosnoe, Mistry, & Elder, 2002; Gilard & Guglielmetti, 2011; Nightingale et al., 2013; Páramo-Fernández, Araújo, Tinajero-Vacas, Almeida, & Rodríguez-González, 2017; Pascarella, Pierson, Wolniak, & Terenzini, 2004; Pritchard, Wilson, & Yamnitz, 2007). In addition, the decision to persist with or withdraw from college may not be necessarily influenced by actual experiences lived by the student: many of students' behavioral and emotional problems may already be present prior or at the moment of entry into college.
One of the pre-entry variables that influence students' adaptation to higher education is academic expectations. These expectations include students' beliefs of self-worth and efficacy, as well as aspirations and motivations for their future in higher education (Howard, 2005). Academic expectations are shaped by students' prior academic expe riences and have a positive influence on students' academic and social integration and engagement, as well as their academic performance (Jackson, Pancer, Pratt, & Hunsberger, 2000; Kuh, Gonyea, & Williams, 2005; Lent, Brown, & Hackett, 2002; Pike, Hansen, & Childress, 2014; Smith & Wertlieb, 2005).
Despite the positive relation found between expectations and educational attainment, prior research has shown that first-year students' expectations for college tend to be unrealistic. While students expect to have the best teachers and colleagues, as well as interesting and successful learning experiences, they also underestimate the amount of hours needed to prepare for classes, turn in written assignments, and study for exams (Howard, 2005; Kuh et al., 2005; Smith & Wertlieb, 2005). Such misadjusted expectations are likely to result in feelings of frustration for a significant number of students, as well as doubts about the ability and motivation to pursue the initial educational plans they had set for themselves. The frustration of initial expectations and dissatisfaction with the first-year experience is positively related to academic failure and attrition (Howard, 2005; Moneta & Kuh, 2005; Pleitz, MacDougall, Terry, Buckley, & Campbell, 2015). Such frustrations are even more important now, at a moment when higher education institutions and tertiary learning goals are changing, while students (and society) are paying a high price for their education and not always maximizing its' benefits. Therefore, it is important that higher education institutions learn more about students' profiles of expectations, in order to tailor their services to what students aspire to when they matriculate.
A multidimensional description of academic expectations is defensible, considering the multifaceted nature of young-adult psychosocial development, adaptation to higher education, and academic environments. Students formulate expectations for future, regarding learning and academic success, personal development and autonomy, career plans, and development of interpersonal relationships with colleagues or the wider community (Pascarella & Terenzini, 2005; Sánchez-Sandoval & Verdugo, 2016). In recent years, a research project involving first-year students from Brazil, Portugal, and Spain developed a multidimensional measure for the assessment of academic expectations in seven dimensions: (i) training for employment and career development (e.g., to attain better qualifications to access the job market and therefore get better jobs), (ii) personal and social development (e.g., to increase levels of self-knowledge and autonomy), (iii) student mobility (e.g., to take part of student exchange programs or training experiences in another country), (iv) political engagement and citizenship (e.g., to engage in the discussion of social problems), (v) attending to social pressures (e.g., to match significant others' expectations or to reciprocate the investment of society in students' education), (vi) quality of education (e.g., to increase relevant knowledge in the scientific area of the graduation), and (vii) social interaction and leisure (e.g., to make new friends and have moments of companionship). Initial results of this project indicate moderate correlations among these seven dimensions suggesting their relative differentiation (Almeida et al., 2012; Deaño et al., 2015).
Attending to student's diversity in higher education, it is also important to analyze intergroup differences in expectations. Family socioeconomic background can play an important role in shaping students' expectations. Students from lower socioeconomic groups tend to have less support and information about the academic and social environments and challenges they will face in the first year in higher education, sometimes presenting unrealistic expectations or lower self-efficacy levels regarding their competencies to overcome such challenges (Crosnoe et al., 2002; Vuong, Brown-Welty, & Tracz, 2010). In addition, first-generation students (those whose parents never enrolled in postsecondary education) have lower self-efficacy, aspirations, and expectations, are at a higher risk of dropping out of college, and have lower levels of extracurricular involvement and interaction with peers in non-course contexts, when compared to students whose parents graduated from college (Pascarella, Wolniak, Pierson, & Terenzini, 2003; Pascarella et al., 2004; Vuong et al., 2010).
A second variable that is significantly related to students' academic expectations and adaptation in higher education is gender, as female students tend to present higher levels of academic achievement and college completion than men (Buchmann & DiPrete, 2006). A major impact of gender socialization in still observed women's decreased participation in science, technology, engineering, and math (STEM) fields in higher education (Fouad et al., 2010; Blickenstaff, 2005; Saavedra, Araújo, Taveira, & Vieira, 2014), when compared to male students, although this gap seems to be currently narrowing in post-graduate training (Ceci, Ginther, Kahn, & Williams, 2014). Male students also present higher levels of status and striving orientation and are more motivated by the career progression and employment opportunities related to the course (Diniz et al., 2018; Sax & Harper, 2007). Female students, on the other hand, have recently outperformed male students in mobility programs (Böttcher, Araújo, Nagler, Mendes, Helbing, & Herrmann, 2016) and are more involved in social activism, including volunteering and community support activities (Sax & Harper, 2007). In addition, female students are more likely to be responsive to others' opinion and social pressure and seem to present lower levels of academic self-efficacy (Huang, 2013; Sax & Harper, 2007).
Finally, age also seems to be a relevant variable for students' transition to higher education and adjustment. Older students are more mature in their study behavior, as they are more likely to adopt a deep learning approach than younger students (Richardson, 2013). Older students are also more motivated to study out of intrinsic interest or for their personal development (Gow & Kember, 1990), have developed a mature sense of self-esteem (Twenge & Campbell, 2001), and present higher levels of career maturity than younger students (Patton, Creed, & Spooner-Lane, 2005). However, the college experience can be more challenging for older students, in terms of their social integration and coping with the academic workload, due to the effort to balance their multiple commitments, including employment and having children (Gilard & Guglielmetti, 2011).
In light of the above, the present paper had two main purposes. First, using latent profile analysis, we sought to identify the expectations profiles that exist in first-year university students. While most research is variable-centered, using correlation or regression analysis, the person-oriented approach in this study will allow to consider personal characteristics and identify subgroups attending to these characteristics (Bergman & Andersson, 2010). Such studies concerning the identification of subgroups of students based on their expectations are scarce. An exception is the study conducted by Jackson et al. (2000), which identified four clusters of students, based on their positive and negative expectations for adaptation and social and academic experiences: optimistic, prepared, fearful, and complacent. However, an analysis of the patterns of students' expectations should also include other information regarding how students expect to enrich their experience (e.g., through student mobility experiences) and achieve personal growth and development. In the current study, the use of latent profile analysis will allow to describe such heterogeneity in students' initial expectations, which will potentially allow higher education institutions to acknowledge diversity in their public's motivations to enroll. We expect to identify distinct subgroups of students, according both to their levels (high, moderate, or low) of expectations and to the salience of specific types of expectations for higher education (e.g., career development, personal and social development, student mobility, political engagement and citizenship, attending to social pressures, quality of education, and social interaction and leisure). A second purpose was to relate the expectations clusters to gender, parents' educational background, and age, as prior research has shown that these variables influence students' outcomes in higher education. By studying the expected influence of such demographic and background variables, we expect to provide further evidence of the validity of the identified profiles. The overarching goal of the study was to contribute to the developing literature regarding higher education students' expectations, exploring variations in expectations in the student population, which in turn could inform future educational policies and psychoeducational interventions to ease the transition of incoming first-year students.
Method
Participants
Participants are 2,478 first-year students (55.8% females, Mage = 18.65, SD = 3.34) at Minho University, a public higher education institution in the north of Portugal. Students attended undergraduate programs in different academic fields: humanities (archaeology, geography, law, history, languages, literatures, philosophy; cultural studies; 16.4 %), social sciences (communication sciences, economics, management, marketing, accounting, political sciences, international business, international relations, public administration, psychology, sociology; 23.3%), education (elementary education, education; 5.3%), natural sciences (biology, geology, biochemistry, chemistry, physics, environmental sciences; 8.7%), formal sciences (mathematics, statistics, computer science; 3.5%), engineering (biological, biomedical, chemical, civil, computer, informatics, telecommunications, information systems, electronic, industrial, physics, materials, polymers, textile; 27.6%), health care (medicine, nursing, optometry and visual sciences; 9.8%), and arts (architecture, music, theatre, fashion design, product design; 5.3%). The participant fathers' educational level was mostly elementary schooling (50.2%), followed by high school (29.2%), and higher education (20.6%). A similar pattern was found in mothers' educational level, although mothers seem to be more educated than fathers are: most mothers are educated at the elementary level (42.8%), followed by high school (30.3%), and higher education (26.9%). The combination of parents' education and occupations allowed the identification of four groups: low socioeconomic status (SES; 24%), medium-low SES (35.4%), medium-high SES (32.9%), and high SES (7.6%).
Instrument
The Academic Perceptions Questionnaire - Expectations (APQ-E; Almeida et al., 2012) assesses the students' beliefs and aspirations in the transition to higher education, including what they expect to find and to develop as a benefit of enrolling in higher education. The items combine cognitive and motivational aspects of the academic experience. A total of 42 items measure seven expectation dimensions (six items per dimension): (i) Employment and career development (professional preparation to have a good job prospect), (ii) Personal and social development (developing maturity and autonomy), (iii) Student mobility (participating in Erasmus or similar programs to have academic or practicum experiences in another country), (iv) Political engagement and citizenship (discussing the world's or country's socio-economic problems), (v) Social pressure (matching parents' and society's investment in students' education), (vi) Quality of education (being in an interesting and academically exciting graduation program), and (vii) Social interaction and leisure (participating in leisure activities and student parties). Students' answers are provided on a 6-point Likert-type scale, with three points regarding low or negative expectations and three points regarding high or optimistic expectations (1= completely disagree, 6 = completely agree).
Concerning evidence of reliability and validity of the test's scores, following international guidelines and standards for psychological testing (AERA, APA, & NCME, 2014; Messick, 1995; Prieto & Delgado, 2010), prior analyses conducted with a sample of first-year students after six months of academic experience (during the second semester) resulted in evidence of adequate psychometric coefficients for each dimension and for internal validity (Deaño et al., 2015). In addition, the instrument's scores present measurement invariance across gender and countries - Portugal and Spain (Diniz et al., 2018).
Regarding the current sample for the present study, confirmatory factor analysis (CFA), using a robust weighted least squares estimator (WLSMV) to accommodate the categorical nature of the data, showed that the APQ-E data of the present sample of first-year students fit the theoretical model adequately, after allowing 11 pairs of error covariances as suggested by modification indexes: χ 2(788) = 9416.19, p < .001, RMSEA = .067 (90% CI = .065 - .068), CFI = .91, TLI = .90. Cronbach's alpha was .82 for Training for employment and career development, .84 for Personal and social development, .88 for Student mobility, .86 for Political engagement and citizenship, .82 for Social pressure, .77 for Quality of education, and .86 for Social interaction and leisure. Therefore, evidence of reliability and internal validity for the test's scores were once more confirmed.
Procedures
The study goals and procedures were presented to the students and confidentiality was assured, after which students provided their formal consent to participate in the study. The questionnaires were administered at the moment students were matriculating in the first year, in the classroom environment. After the 12th grades examinations in June and July, students apply for college providing their preferences in a rank of six pairs of graduation course and institution. The Education Ministry then distributes students based on prior GPA and access exams according to the numerus clausus system. Results of this national competition are announced in early September, after which students have a week to matriculate in their respective institution and undergraduate program. Therefore, the questionnaires were answered when students confirmed their interest in the course and institution where they were placed.
Data Analysis
Subgroups of students were identified using latent profile analysis, a variant of latent class analysis for continuous variables and a sub-group of finite mixture models, which aim to discover relationships between dependent variables through categorical latent variables. Unlike traditional cluster analysis, latent profile analysis is a model-based method that fits a statistical model to the data, classifying each case (person) in the most probable group (i.e., latent class) based on responses to a set of observed variables. Despite being somewhat similar to confirmatory factor analysis, because both techniques estimate latent variables from a set of observed indicator variables (Wang & Wang, 2012), latent profile analysis provides classification of people instead of variables. Therefore, it can be considered “a person-centered analytic tool that focuses on similarities and differences among people instead of relations among variables” (Berlin, Williams, & Parra, 2014, p. 174).
Latent profile analysis assumes that the dependent variables are continuous and normally distributed within each latent class. Therefore, the distribution of each person's scores on the dependent variables (xi ) given the latent classes (𝜃) is a function of the probability of these persons being a member of a class (πκ)through the normal density function (
), where 𝜃κ composes the estimation of a mean and the variances/covariances for each latent class (Tein, Coxe, & Cham, 2013).
Given a categorical latent variable 𝜃, which includes a number of classes, the dependent variables x i are explained by a function (
). This function is the probability (𝜋) of any person being a member of a class (𝜅) through the normal density function (
).
Latent profile analysis is capable of answering about how many classes exist in the data and the meaning of these classes. Because the number of latent classes is unobservable and cannot be estimated directly from a given data set, model fit statistics and indices are applied to assess the goodness-of-fit of the mixture model (Wang & Wang, 2012). Mplus (Muthén & Muthén, 2012), which was used in the current study, provides such statistics. To determine the optimal number of classes in the model, a series of latent profile analyses models with increasing number of latent classes are fit and the number of classes is determined by comparing the k-class model with the (k-1)-class model iteratively. The Akaike Information Criterion (AIC) and the Bayesian Information Criterion (BIC) are usually applied as goodness-of-fit measures. The best model is the one that presents the lowest AIC and BIC values. Other two types of model data fit indices and statistics for model comparison are the Vuong-Lo-Mendell-Rubin likelihood-ratio test and the bootstrap likelihood ratio test. The first compares the estimated k-class model with the (k - 1)-class model. The p-value informs the hypothesis that the model with (k - 1)-classes has an equal or a better data fit than the k-class estimated model. Therefore, a p-value below .05 indicates that the estimated k-class model is better than the (k - 1)-class model, which is therefore rejected in favor of a model with at least k classes. The bootstrap likelihood ratio test follows the same rationale of the Vuong-Lo-Mendell-Rubin likelihood-ratio test, where parametric bootstrapping is used to generate a set of bootstrap samples using the estimates from the (k - 1)-class model, and each of the bootstrap samples is analyzed for k-class and (k - 1)-class models (Wang & Wang, 2012). This statistic allows to compare the estimated k-class model with the (k - 1)-class model and also presents a p-value for the null hypothesis.
In addition to these statistics and indices, entropy should be used as a criterion of the quality of class membership classification, informing about the accuracy of the model to classify the persons in the classes. Mplus (Muthén & Muthén, 2012) reports the relative entropy of a k-class model, ranging from 0 to 1, where a value closer to 1 indicates better classification. For example, a value of .95 means that the model has a probability of 95% in accurately classifying the person in the classes of the model.
Beyond these indices, an aspect to consider when choosing the best model is the size of the classes. In order to have a meaningful class classification, classes should not be too small. Any given class should include at least 1% of the sample or 25 cases (Tein et al., 2013). Small classes should not be considered because of low statistical power (Type 2 error) and low generalization power. The decision to maintain certain classes which do not fit these cut-off values needs to be theoretically supported by the researcher. All latent classes need to be meaningful and interpretable.
In the current study, fifteen models, specifying one latent class to 15 latent classes, were estimated using Mplus 7.0 (Muthén & Muthén, 2012), through robust full information maximum likelihood (MLR) estimation. Latent classes were explored using the seven factor scores of students' expectations, which were standardized with a mean of zero and a standard deviation of one. All the 15 models constrained the class-specific covariance matrix as a diagonal matrix, with the covariances among the dependent variables equal to zero, and the variances across the latent classes of a model also equal to zero. Therefore, all the tested models assumed local independence and variance homogeneity, only allowing for variation in the classes means.
The selection of the best model was chosen by the combination of the Akaike Information Criterion (AIC), the Bayesian Information Criterion (BIC), the Vuong-Lo-Mendell-Rubin likelihood-ratio test, the bootstrap likelihood ratio test, the entropy information, and the size of each class of the model. After choosing the best model, we ran pseudo-class draws for a posterior probability-based multinomial logistic regression of the latent class variables on gender, SES, age, and mothers' and fathers' education levels, in order to understand which of these variables would explain the latent classes. This was accomplished by performing a path analysis through maximum likelihood robust estimator via Mplus 7.0 statistical software (Muthén & Muthén, 2012). SES, age, and education level were transformed in dummy variables. Low SES served as the baseline, while the categories of middle-low SES, high-middle SES, and high SES were transformed in dummy variables. Age was dichotomized in values below 20 years (= 0) and equal to or above 20 years (= 1). The education level of the father and the mother were dichotomized in categories below higher education (= 0) and higher education (value 1).
Results
The best loglikelihood value was obtained and replicated at least twice in all the analyzed models. This is a strategy that aims to avoid maxima local, which leads to estimation problems. Table 1 presents the fit indices of the 15 models, including the Akaike Information Criterion (AIC), the Bayesian Information Criterion (BIC), the entropy information, the Vuong-Lo-Mendell-Rubin likelihood-ratio test, the bootstrap likelihood ratio test, and the number of classes that do not achieve at least 1% of the sample.
Table 1. Data Fit of All Models.
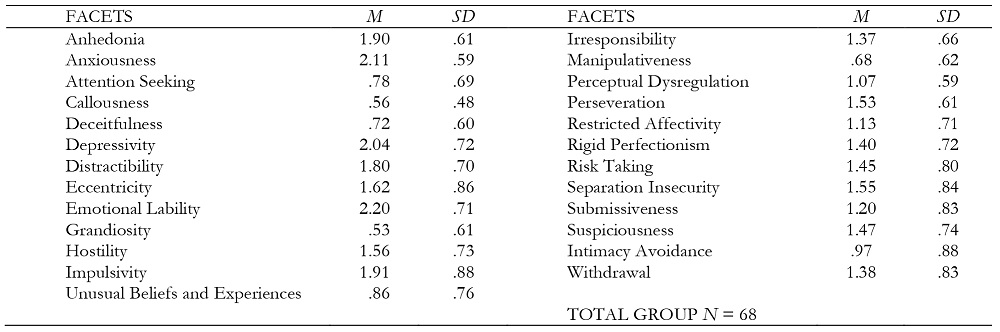
Note. LRT = Vuong-Lo-Mendell-Rubin likelihood-ratio test; BLRT = bootstrap likelihood ratio test.
The Akaike Information Criterion (AIC) and the Bayesian Information Criterion (BIC) had lower values for each model that increased one class. Model 2 presented lower AIC and BIC values than model 1, model 3 showed lower values than model 2, and so on. Six models - model 9, model 10, model 12, model 13, model 14, and model 15 - presented classes that showed less than 1% of the sample and were therefore rejected by the established criteria. However, the more restrictive criterion was the Vuong-Lo-Mendell-Rubin likelihood-ratio test, because it showed that only models 2 to 6 presented a p-value < .05, allowing to reject the null-hypothesis that the (k - 1)-class model is equal to or better than the estimated model. Combining all the criteria, the selected model was the sixth model, which presents an entropy of 80.4%, indicating that the six classes are capable of accurately classifying all the sample in 80%.
Table 2 informs about the absolute and relative percentage of students in each class of the best model, as well as the accuracy of classification in each class. Three classes include the majority of the sample: class 5 includes 36% of the students, class 4 includes 27% of the students, and class 3 includes 22% of the students. Taken together, these classes account for 84-85% of the participants of the study. The classes with fewer students are class 6 (8%), class 1 (4%), and class 2 (4%). Regarding the average latent class probabilities for the most likely latent class membership, table 2 shows that the most accurate is class 1, which has an accuracy of 90.0% (see the diagonals in table 2 for the accuracy of the classes). Class 2 has the lowest accuracy amongst all the six classes, with an accuracy of 81.7%. On the other hand, class 5 has the highest probabilities that its members are classified as members of another class, with a probability of 12.3% of being classified as a member of class 2, a probability of 10.8% of being classified as a member of class 3, and 8.0% of being classified as a member of class 4. This indicates that class 5 has the closest proximity to the other classes.
Table 2. Frequency of Students in the Classes and Accuracy of Classification in Each Class of the Best Model.
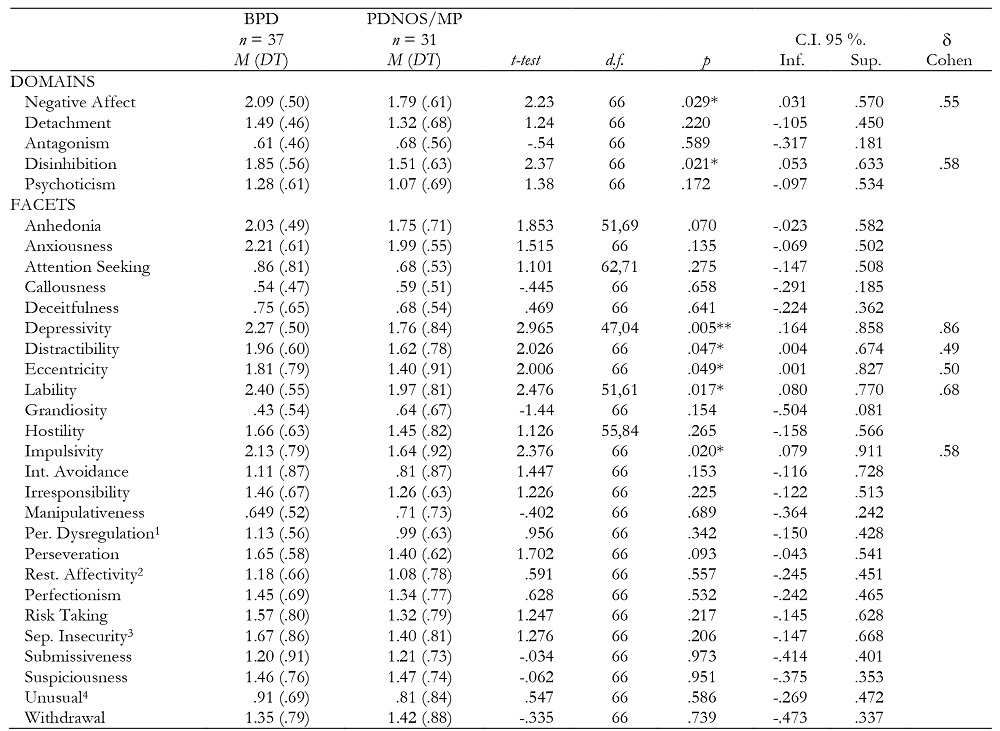
Note: af = absolute frequency; rf = relative frequency.
The six classes of the chosen six-class model present a variance of .47 in training for employment and career development expectations and a variance of .24 in personal and social development expectations, as presented in table 3. Regarding these values, as informed in the data analysis subsection, the dependent variables are factor scores with mean zero and standard deviation of one. Therefore, the variance of .47 in the training for employment and career development expectations, for example, represents a deviance of 0.47 standard deviation points from the mean.
Interpreting the classes, five of the six classes have similar means among the dependent variables, meaning that there are not significant qualitative variations in the seven dependent variables to define these classes. For example, in class 1 students show expectations means below one standard deviation in all of the seven dimensions of academic expectations. The lowest mean in class 1 is observed for social interaction and leisure expectations (-1.79) and the highest mean is presented for training for employment and career development expectations (-1.15). The mean of the means in class 1 is -1.49 with a standard deviation of 0.20. The same pattern occurs in class 3, class 4, class 5, and class 6. Class 3 presents a mean of the means of -0.70 with a standard deviation of 0.11, with the lowest mean in personal and social development expectations (-0.84) and the highest mean in attending to social pressure expectations (-0.55). Class 5 shows a mean of the means of -0.12 and a standard deviation of 0.07. Its lowest mean is in personal and social development expectations (-0.19) and its highest mean is in student mobility expectations (0.00). Class 4 has a mean of the means of 0.54 and a standard deviation of 0.08, with the lowest mean in student mobility expectations (0.40) and the highest mean in personal and social development (0.66). Finally, class 6 shows a mean of the means of 1.24 and a standard deviation of 0.21, and the lowest mean in training for employment and career development expectations (0.85) and the highest mean in personal and social development, political engagement and citizenship, and social interaction and leisure expectations (1.38 for all of them).
Therefore, these five classes (classes 1, 3, 4, 5, and 6) should be interpreted as general academic expectations, which vary in magnitude. Class 1 represents the group with the lowest general academic expectations. Class 3 represents the group with moderate-low general academic expectations, in relation to the sample distribution. Class 5 includes the group which presents average general academic expectations. Class 4, on the other hand, includes a group which has moderate-high general academic expectations and class 6 represents a group with the highest general academic expectations. It is important to say that the terms lowest, low, average, high, or highest are adequate and pertinent for the groups in comparison with the total sample of participants. The norm of distribution of the sample is the reference for the interpretation regarding these terms (what is the lowest, what means low, high, and so on). In sum, these five classes represent low academic expectations, moderately low, average, moderately high, and high academic expectations, but, as commented, all in relation to the sample distribution.
Class 2 presents a different pattern of academic expectations. It shows a mean of means of -0.05, similar to class 5, but has a considerable standard deviation of 0.68. This class includes students with low social interaction and leisure expectations and attending to social pressures expectations (-1.08 and -0.85, respectively) and presents moderately high academic expectations in political engagement and citizenship expectations and quality of education expectations (0.62 and 0.65, respectively). In this class, students do not show considerably high expectations in attending to social pressures and social interaction and leisure expectations but show moderately high expectations in quality of education and political engagement and citizenship expectations. The other academic expectations are close to the average values for the sample.
The path analysis which explained the classes based on gender, age, SES, and parents' educational level had 18 free parameters, a loglikelihood of -5225.805, a Bayesian index criterion of 11392.057 (11334.867 sample size adjusted), and an Akaike index criterion of 11287.610.
Table 4 presents the odds ratio for the independent variables in each class. Class 1 is explained by age and gender. Females were 3.99 times more likely to be included in this class than male students, and students with ages below 20 years were 4.02 more likely to be included in this class than students with ages above 20 years. Class 2 is explained by age, gender, and low-middle SES and high SES. The most important variables in this class are high SES and age. High SES students are 7.60 times more likely to be included in this class than low SES students and younger students are 3.75 times more likely to be included in this class than students with ages above 20 years. Class 3 is explained by gender, with female students being 2.16 times more likely to be classified in this class in comparison to male students. Class 4 is explained by age, gender, and fathers' educational level. Males, students with ages above 20 years, and students whose fathers are graduated are more likely to be included in this class. Class 5 is explained by age, with older students (ages above 20 years) being 1.42 times more likely to be included in this class. Class 6 is explained by gender, with males 1.98 times more likely to be included in class 6 in comparison to females.
Discussion
The main purposes of this study were to identify profiles of first-year students' expectations at the moment they are matriculating in university and then assess the relationship between these profiles and sociodemographic variables, including gender, SES, age, and parents' educational background. Confirming heterogeneity in students' characteristics in their transition to higher education, we identified different subgroups of students according to their expectations in the studied sample of first-year students. In addition, as expected, these profiles were influenced by background characteristics.
In our study, we identified six statistically significant and interpretable profiles, although only one presents qualitative variations in the assessed dimensions. Five of the identified classes present similar means in the assessed seven expectation dimensions, and therefore comparisons between these clusters are solely significant considering the average level of expectations, overall. Only one of the identified classes is characterized by some differentiation of the type of expectations students present in their transition to higher education: these students presented lower expectations for social integration and leisure and attending to social pressures, moderate expectations for political engagement and citizenship, as well as for the quality of their education at university, and close to the average expectations for training for employment and career development, personal and social development, and student mobility. Students in this profile seem to be more interested in personal, academic, and career development experiences, rather than conforming to social pressures or developing their social networks.
A further examination of the identified profiles of student expectations shows that more than differences in the dimensions of expectations, what contributes to the identification of the classes of students is the variation in the level of expectations. Classes 1, 2, and 3 are composed of students with lower average scores in the various dimensions of expectations, while classes 5 and 6 include students with higher average expectations scores. Therefore, although literature has described first-year students' expectations as being generally high (Diniz et al., 2018; Jackson et al., 2000; Smith & Wertlieb, 2005), our study showed that students indeed vary in their visions of the benefits of higher education: while some are unrealistically positive about the university experience, others seem to have moderate expectations, and others present a more negative perspective for their academic future. In addition, the observed lack of differentiation in students' expectations in most identified clusters is congruent with prior research which has shown that, in general, students' scores on the different dimensions of expectations are highly and positively intercorrelated (Deaño et al., 2015; Diniz et al., 2018), suggesting that such self-reported expectations may be influenced by more stable dispositions, including self-efficacy and personality (Nightingale et al., 2013; Vuong et al., 2010).
Further, the present findings showed that men are more likely to be included in high-expectations profiles, while women are more likely to be included in low-expectations profiles or classes. These results are in accordance with literature in this domain: despite actually composing the majority of students at university and generally presenting higher academic engagement levels and academic success (Buchmann & DiPrete, 2006), women seem to systematically present lower career self-efficacy and outcome expectations, when compared to male students, especially for engineering and science careers (Fouad et al., 2010). According to the social cognitive career theory (Lent et al., 2002), lower self-efficacy and career expectations are due to less exposure to positive performance experiences in the past, role models, and encouragement. In the current study, female students may present lower expectations for the benefits of higher education because they globally perceive they will have fewer opportunities to engage in social, political, mobility, and research experiences. The personal (e.g., low self-efficacy and expectations) and environmental barriers (e.g., gender role stereotypes, lack of role models) perceived and experienced by these women are potential risk factors for their career advancement. Future studies should also analyze the role of students' study field on initial expectations, as such barriers are more relevant when students engage in non-traditional careers (Fouad et al., 2010).
The results also suggest that younger students (with ages under 20 years), who access higher education immediately after secondary education, have lower expectations when compared to older students. Such higher results of expectations for older students may be influenced by their career maturity (Patton et al., 2005) and development of the self (Twenge & Campbell, 2001). Due to the challenges older students face when they enroll in HE, including balancing their family and work responsibilities with time spent in class and working on assignments (Gilard & Guglielmetti, 2011), such high initial expectations may place these students at risk of struggling while adapting to higher education. Student affairs should, therefore, pay special attention to these students, providing, for example, accurate and timely information about the workload students are expected to accomplish and facilities provided for these students' life-balance and academic development (e.g., special evaluation moments for working students, online education tools, on-campus facilities for childcare).
In the current study, we identified a profile (class 4) in which students presented moderately high expectations that are more likely to favor their adaptation and academic engagement. In this case, students are potentially protected against the negative impact of unrealistic expectations, which would most likely contribute to frustration and dissatisfaction with the university experience (Moneta & Kuh, 2005; Pleitz et al., 2015). This identified profile is a group mainly composed of men and older students, whose fathers concluded higher education degrees. These findings support prior research that showed that students whose parents have higher educational statuses may be at an advantage when compared to first-generation students, who have less support and information about the academic and social challenges related to higher education, while also presenting misevaluations regarding their own competencies to meet such challenges (Crosnoe et al., 2002; Vuong et al., 2010).
Finally, class 2, which includes students with higher expectations for the quality of education and political engagement and citizenship and lower expectations for social interaction and leisure and attending to social pressures, is mainly composed of male students and students from families with higher SES. These findings support prior research that showed male students, compared to females, tend to present higher career expectations and are less likely to be influenced in career planning (Fouad et al., 2010), while first-generation students and those from families with lower SES present lower expectations and self-efficacy beliefs, compared to students from more advantaged backgrounds (Crosnoe et al., 2002).
To conclude, this study is not without limitations or suggestions for further research. The current study illustrates the adequacy of using the Academic Perceptions Questionnaire (Almeida et al., 2012) to inform about differences in first-year students' expectations and to identify different groups of students according to their entry characteristics. The validity of the identified clusters could be further evaluated by following the students and assessing their achievement and adjustment in the second academic term. Because prior research has already identified relations between expectations and adjustment to HE and academic success (Jackson et al., 2000; Smith & Wertlieb, 2005), we anticipate that students with more realistic, albeit optimistic, expectations for their experience in the first year will also present better indicators of academic and social integration and academic performance.
In the current study, clusters were not validated with external criteria, such as students' adaptation, academic performance, or satisfaction. Such validation can be pursued in future research. However, we should state that the classes found in the current study present consistent preliminary evidence of validity, since the validity of the model of six classes was scrutinized by the combination of the Akaike Information Criterion (AIC), the Bayesian Information Criterion (BIC), the Vuong-Lo-Mendell-Rubin likelihood-ratio test, the bootstrap likelihood ratio test, the entropy information, and the size of each class of the model. The evaluation of these indices showed that the model of six classes produced a highly qualified classification of the students, with a good probability of the students being included in their respective assigned class, which is an evidence that corroborates the validity of the identified model of six classes.
Because we collected students' expectations at the beginning of the year, students' have not developed yet a differentiated perspective of what their college experience will be, which may have contributed to the lack of qualitative differentiation in expectations scores in most of the identified profiles. Therefore, in future research, we suggest assessing students' expectations after three or four weeks of college experience, while acknowledging that these expectations may be contaminated by students' confrontation with their initial academic and social experiences. Future studies could also look into the influence of other background and academic variables that could influence the composition of profiles of expectations, including prior academic achievement and current academic field. In addition, self-reported measures of expectations could be complemented with other-rating measures, including peers' assessments, to support the validity of the findings.
The diversity in the configuration of students' expectations found in the current study and the relations observed between the profiles and sociodemographic variables show that first-year students are a heterogeneous group. Such a diversity is mainly the result of the democratization of the access to higher education. Institutions should take a closer look at such student diversity, as it is now evident that not only do students access higher education with different backgrounds, but these backgrounds also seem to impact what students expect from their academic experience. Attending to students' expectations should be a multi-targeted effort for institutions, as our study showed that older and younger, male and female, and first- and second-generation students have different levels and profiles of expectations.