Introduction
Patient safety is an essential objective in healthcare, and voluntary reporting systems for medication errors can help to avoid their recurrence. But regardless of the system advantages, it is necessary to conduct a data refining task.
This article analyzes the errors reported in a database (DB) and the differences between the raw and refined databases, suggesting improvements that can be useful for the scientific community, and particularly for the quality of healthcare offered by health centres.
The National ENEAS Study for healthcare-related adverse events (AEs)1 confirmed that 37.4% of the events found were associated with medications, and 42.8% of them were avoidable.
Reporting systems represent a key strategy to learn from errors and prevent their recurrence2. Those focused to the improvement of safety are voluntary3, mostly focused on incidents or errors causing minimal damage, that allow to conduct a Root Cause Analysis and build up a real caseload of quasi-errors or incidents, in order to identify the system weaknesses and train professionals on the knowledge collected from multiple cases4, thus helping to improve the most critical points in the structures process.
The limitations attributed to these systems 5,6 are essentially an underestimation of prevalence (in fact, they are not useful for its determination), and varied reporting biases; but limitations or reservations regarding the quality of report data are not typically included. A comprehensive report on the systems for reporting adverse events throughout the world (“Sistemas de registro y notificación de incidentes y eventos adversos”) by the Spanish Ministry of Health7 makes no reference to this specific matter within its 149 pages. In 2009, our hospital set up the SINEA system8,9 for voluntary AE report in healthcare; this system was subsequently adopted by all the health departments in the Conselleria de Sanitat of the Valencian Community. SINEA is an electronic system that can be accessed by all the health department professionals through the intranet. It has received institutional support, by the inclusion of the report in SINEA within the 2014 management agreements for all departments10.
SINEA has been designed for direct on-line capture. This eliminates a previous data record, and prevents the lack of correspondence between the paper format, which will cease to exist, and the electronic record. There is plenty of research about the design of applications to improve results in terms of record errors and to facilitate data processing11,12, though these rarely deal with healthcare databases, and particularly databases for recording drug-related problems (DRPs)13. Despite its advantages vs. deferred capture, information consistency cannot be ensured, and therefore this does not guarantee record quality per se. Refining14 is defined as the process to be conducted after data capture, in order to detect and correct any errors contained; it is considered essential if data have been entered with software that has no process implemented for protection, or this is incomplete15.
In this scenario, we raise the matter of the validity of data contained in SINEA regarding medication errors (MEs) and adverse reactions to drugs (ADRs).
The objective of this study is to determine the number and type of errors contained in the SINEA database, with the aim to suggest potential improvements in the form of protections in the capture process in order to reduce them, to confirm the differences in the results obtained based on the raw and the refined databases, because if these were not significant, the refining process, which is long and complex, could be avoided; and finally, to consider the need for extending the training for reporters, based on the analysis of the results.
Methods
An EXCEL file was prepared with the AEs reported in the department between January, 1st and August, 30th, 2014. The SINEA database has 212 fields; 121 associated with drug-related AEs were analyzed, and 49 were refined, as they were considered relevant for this study: those regarding hospital unit, event classification, date, coding for the description/s of the event, impact on patient and healthcare, molecule, and medication involved. Ten of these fields were considered key elements for joint analysis: who discovered the error, professional category of the person involved and the reporter, what happened and where, brand name, molecule, hospital unit, description of the error, and causes.
All records were manually reviewed in order to determine the consistency between what appeared in the free-text field “describe_what_happened”, which was considered the gold standard, and the classification as adverse reaction or medication error. Once correctly coded, the entries for ADRs and MEs were analyzed separately. The study variables were refined, detecting any incidences caused by inconsistencies and unknown values through logical analysis. Thus, it was confirmed that the entry date was not previous to the event or incident date, and that these two were not previous to December, 2013 or after August, 2014. It was confirmed that errors classified as potential were associated with “no impact on healthcare” and “no harm for patient”, and the opposite in the case of ADRs. The majority of variables required manual refining, because valuable information was included in free-text fields. A Pharmacist specialized in DRPs and reporting systems was responsible for correcting any incidences detected by entering the correct value in all cases where possible, and assigning an unknown value when this was not possible; and finally, a descriptive statistics was prepared for the errors detected after refining and the unknown values in the final data. No probabilistic controls were applied.
A comparison of the values obtained from the analysis of the raw and the refined databases was made, for the following variables: percentage of MEs, percentage of potential and real MEs, and percentage of ADRs, distribution of impact on patients, distribution of impact on healthcare and drugs more frequently involved.
The Cohen’s kappa agreement index was calculated between the reported and refined16, data for the classification of AEs (ADR, ME, or event not associated with medication), and for the type of ME (potential ot real).
Results
During the eight months of the study, 364 AEs were reported, with a 31.3% index of non-completion in some of the 10 fields considered essential. During the refining process, 552 changes were made in the 275 records of drug-related events (the mean errors per record was 2.01 SD 2.11). The 21 adverse reaction records contained more errors (mean 4.57 SD 2.82) than the 254 records for medication errors (mean 1.80 SD 1.90).
241 AEs were classified as MEs (1 was re-classified as ADR), 8 as ADRs, and 2 as both, which were re-classified as MEs because they were allergic reactions caused by the administration of medications with documented allergy.
Among those 113 AEs not identified as DRPs, 12 ADRs and 12 MEs were found during the refining process.
After refining the 364 AEs, 254 MEs were found (69.8%), as well as 21 ADRs (5.8%) and 89 events not associated with medications (24.4%). The raw database would have provided the following percentages: 66.7%, 2.7% and 31.0% respectively. Given that two events had been simultaneously and wrongly classified into two categories, the total percentage exceeds 100%.
All reporting dates are correct, because they are automatically entered by the system. The hour when the event occurred cannot be analyzed, because 92.5% were recorded as occurred at 9 a.m., and there are no clues in order to rectify this piece of data.
The calculation of the Kappa coefficient for classification into type of AE is shown in Table 1.
Table 1 Calculation of Cohen’s Kappa Coefficient for total AE classification.

DB: database; ADR: adverse reaction to drug; ME: medication error; OTHERS: adverse events not associated with medications. Number of coincidences observed: 337 (92.58% of the observations). Number of coincidences expected by hazard: 196.4 (53.95% of the observations). Kappa = 0.839 SD for kappa = 0.028 95% Confidence Interval: 0.784-0.894 The agreement obtained can be considered VERY GOOD.
Medication errors
73.6% of errors were potential, 82.3% in the raw database due to poor coding in 32 cases (12.6%). The calculation of the Kappa coefficient appears in Table 2.
Table 2 Calculation of Cohen’s Kappa coefficient for the classification of potential and real medication errors.
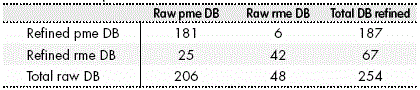
DB. Database; pme: potential medication errors; rme: real medication errors. Number of coincidences observed: 223 (87.80% of the observations). Number of coincidences expected by hazard: 164.3 (64.69% of the observations). Kappa = 0.654 SD for kappa = 0.056 95% Confidence Interval: 0.544-0.764 The agreement obtained can be considered GOOD.
In the 254 MEs reported, 76 records (30.0%) were found to contain errors in the fields Date of the Event or Incident, 41 errors due to entering non-valid dates, and another 35 errors due to discrepancies between the date of the event and the incident.
The distribution of the effect on the patient and impact on healthcare are shown in Table 3, according to the categories pre-defined in SINEA. There are no elements that will allow to refine them.
In 133 ME cases, the molecule was not reported, or the brand name was recorded instead, which made impossible a direct analysis of those drugs involved with higher frequency. Likewise, it was not possible to conduct a direct analysis of MEs according to the member of staff who discovered them, or the category of the professional involved, because these were free-text fields, which provided non-homogeneous data. In the refined database, trastuzumab was the drug most frequently involved in errors (20.9%), which were always potential. Once re-coded, the Pharmacist discovered 67.3% of all errors reported, followed by the nurse (19.3%).
The frequency of the different types of error identified appear in Table 4, for Total Errors and Real Errors. Refinement reduced the percentage of errors classified as “others”, due to re-classification into types of error already defined. Said table shows the total number of errors detected, which is superior to the number of entries classified as ME, because each record can contain more than one error.
Table 4 Types of total and real errors reported from the raw and refined database.
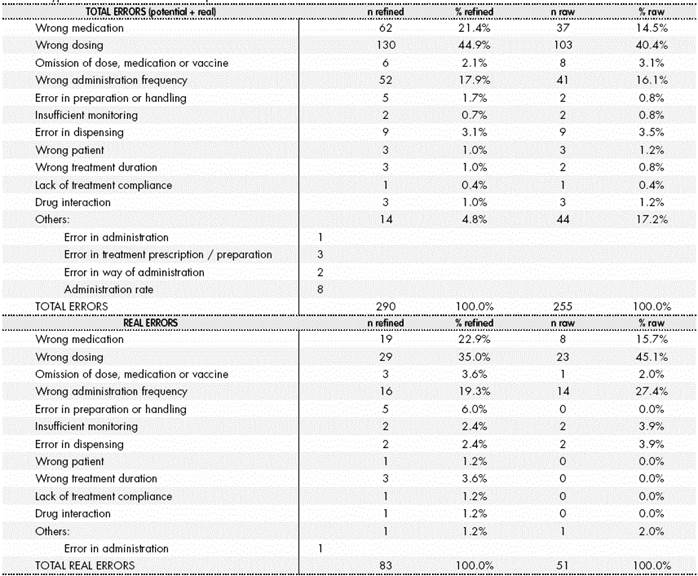
The total number of errors is higher than the number of entries, because each entry can contain more than one error.
ADR
The dates for ADR reports were correct in 100% of cases. These were discovered in 61.9% of cases by the Primary Care Physician, followed by the staff at the Home Hospitalization Unit, with 9.5% of cases. In the unrefined database, 11 inconsistencies (52.4%) were found in terms of impact on healthcare, and 13 (61.9%) in the reported effect of the ADRs. All of them were re-codified according to the damage described in the field “describe_what_happened”. From this same field, 16 of the 21 medications causing the ADR were retrieved. The other 5 did not appear in any field in the original database.
Types of ADR were completely codified in 14 cases (66.7%), but were incomplete in the rest (Table 5).
Discussion
The number of reports in SINEA was limited until 2014. The inclusion of a minimum objective of reports per hospital unit in management agreements has allowed to have a significant number (364 in the first 8 months, object of this study). The AEs associated with the use of a medication can be approached from an individual perspective17, or from a collective point of view allowing the identification of the risk factors and characteristics associated with them in a group of patients. This study was aligned in the second group1, though SINEA also allows a team of reviewers, who receive an alert e-mail for every new report, to trace in real time the report of sentinel events individually (the only analysis that was possible in previous years, due to the low number of reports).
The proportion found in this study of blank fields among those considered key is slightly superior (31.3%) to the one described by pharmacovigilance centres18; however, a major part of the information was retrieved during the process of retrieval for free-text fields. Pharmacovigilance centres have wide experience in the validation of data obtained through spontaneous report of adverse events. Between 20 and 25%18 of all Yellow Cards require some additional information, which is generally collected by phone. This refinement, however, is conducted before entering data on the database.
In a voluntary Adverse Event Reporting System that can be assessed locally, without any specific provision for refinement, it is important to be aware of the quality of raw data, and to implement measures to minimize the need for manual refinement. In this case, data quality was apparently poor, with deviations of almost 114% in some variables, such as the percentage of adverse reactions identified before and after refinement. Given the reduced number of ADRs, these deviations might not be showing the real quality of data.
Kappa Coefficient was originally proposed by Cohen (1960) for cases with two evaluators or two methods. This agreement measure has been designed for nominal classifications, where there is no ranking order between the different categories; therefore, it is adequate for this study19. Landis and Koch20 proposed some margins to assess the level of agreement based on the Kappa Coefficient; in this way, values between 0.4 and 0.6 indicate a Moderate Level of Agreement, between 0.6 and 0.8 represent a Good Level of Agreement, and between 0.8 and 1, a Very Good Level of Agreement.
According to these margins, the agreement obtained in the classification of the event as ADR, ME, or event not associated with medications, was Very Good (kappa = 0.85 CI 95% 0.79-0.90), regardless of the striking underestimation of ADRs reported (2.7%) vs. real ones (5.8%). It was evident that there was a difficulty to differentiate ADRs from other events. The simultaneous classification of two events as ADR and ME allowed to draw two conclusions: the database should be modified so that these two categories were mutually exclusive, and it is necessary to train professionals in terms of event classification: an anaphylactic reaction to a medication can be considered an adverse reaction, if there are no data available to prevent it, but it will become a medication error if there is previous information available about a declared allergy, and this has been overlooked during the process of prescription, validation, and/or administration. In the case of ADRs, by their own definition, all of them should affect the patient, and all patients will suffer a damage to a higher or lower extent, which should have an impact on healthcare. This issue also requires some training reinforcement, because there was a >50% of inconsistencies in both items. The classification of an event as ADR is relevant, because only in these cases, SINEA will offer at the end of the reporting process the option to complete the Yellow Card to be sent to Pharmacovigilance Centres. Better training is also required in order to differentiate between real and potential MEs. In this case, there was a Moderate-Good agreement (kappa 0.65 CI 95% 0.54-0.76).
Refinement is a costly process, performed essentially through the free-text field “describe_what_happened”, which however allows to have plenty of quality data in order to make improvements in the pharmacotherapeutical process. Regardless of the agreement values obtained in the primary variables (type of adverse event and type of error), the tedious process of refinement cannot be skipped. If this was not conducted, for example, the ability to analyze the molecules most frequently involved would be lost, because this field was blank or contained errors in 133 cases (48.5%). Refinement allows to learn very important data, such as the fact that the highest number of errors reported involves the prescription of trastuzumab, and therefore to investigate the causes for this. This error is due to the fact that electronic prescription does not allow to program automatically different doses in different cycles, which requires manual modification. Structured mechanisms of prescription have been recommended for trastuzumab and other drugs that also require a loading dose in the first cycle (cetuximab), in order to minimize the risk of error, and alerts have been implemented in the second cycle, warning about the need for dose reduction. The emergence of new errors due to the implementation of new technologies has been described21, and those associated with electronic prescription22,23 are the cause for around 60% of these24. There are many incidents and alerts in literature about the likelihood of error due to the confusion between trastuzumab and adotrastuzumab emtansine25, but no errors have been found regarding dosing errors due to the persistence of loading doses. It has been demonstrated26 that the variables with the highest impact on the acceptance of a voluntary reporting system are ease of use and the ability to draw conclusions that allow to learn; and this case is a good example of the latter. On the other hand, the great number of errors associated with cytostatics is due to the fact that, during the process of prescription validation, the Pharmacist in charge of this process will report all errors found, while reporting is less comprehensive in other settings. As already mentioned5, voluntary reporting systems are not useful to determine prevalence, precisely due to the occurrence of these reporting biases.
The errors detected in date entries can be rectified through re-coding, following a logical sequence; but it would be preferable to change free-text fields by data fields with an incorporated logical control (the date of the event cannot be posterior to the date of the report, for example). There was a statement of the importance of training on the convenience on reporting the exact hour of the event, in order to analyze the density of events per work shifts. Likewise, the use of a drop-down list to classify the member of staff involved and who discovered the error appeared as a measure easy to implement, with high impact on the ease of analysis.
As a conclusion, it could be noted that for the time being, database refinement before its analysis seems to be unavoidable, and it would be convenient to set up some training practice sessions for reporters, as well as to introduce logical controls in the fields for event classification, date, and impact_on_patient in the case of ADRs, and to convert into drop-down fields with closed options the fields for who_discovered, professional_category and molecule; however, this latter process would have its difficulties, and its feasibility should be assessed.