Introduction
Today, our world may be described as VUCA (volatile, uncertain, complex, and ambiguous; see Bennett & Lemoine, 2014). The current tumultuous market today is the product of highly dynamic processes in the organizations, all in the context of technological development and advancement, globalization, and increasing competition (Corrales-Herrero & Rodríguez-Prado, 2018; van Tulder et al., 2019). It is clear that working in such conditions will have an impact on the organization and its members alike. Whether it is to meet customers' expectations and demands, reach an organizational goal, or applying new and innovative working methods/techniques, the modern market inherently demands a heavier investment at work (Corrales-Herrero & Rodríguez-Prado, 2018; van Tulder et al., 2019). Professionals and managers are faced with especially high work requirements and experience an imbalance between them and their other obligations/commitments in life (e.g., leisure and family; see, e.g., Rabenu, in press; Skinner et al., 2012).
In addition, increasing attention had been given to dispositional heavy work investors who may exhibit work devotion, work passion, immersion, and involvement at work (e.g., job engagement; Bailey et al., 2017; Lebron et al., 2018; Shkoler & Kimura, 2020; Shkoler & Tziner, 2020; Tziner, Buzea et al., 2019; passion to work; Snir et al., 2014), addiction to work (e.g., workaholism; Baruch, 2011; Snir & Harpaz, 2015; Shkoler, Rabenu, & Tziner, 2017; Shkoler, Rabenu, Vasiliu, et al., 2017) and more. Of course, time investment, solely, at work does not necessarily represent an employee's favourable (or, even, truthful) attitude towards it (van Beek et al., 2011). Golden (2015) described this: “... at least some people are driven to work longer hours beyond their initially preferred time commitment, defined as 'overemployment', and perhaps beyond their own capacity that is sustainable in terms of physical or mental health or well-being, referred to as 'overwork' (p. 140). For example, workers' appearance of being very immersed and/or involved at their work (“hard working employee”) might be nothing short of presenteeism, for reasons such as impression management motives or lack of autonomy to decide regarding working hours (Rabenu & Aharoni-Goldenberg, 2017). As such, recognizing the ambiguous nature of “time”, this study also scrutinizes the investment of efforts, not only time, at work. Hence, the purpose of this paper is to propose and validate a relatively new re-conceptualized construct named heavy-work investment (HWI; Snir & Harpaz, 2012, 2015).
Heavy-Work Investment (HWI)
Employees usually invest time and energy at their workplace in many different ways, such as staying longer hours at work, working excessively, thinking how to solve work challenges even while at home, and connecting to work (remotely) after the formal working hours. All of these “investment at work” constructs ultimately boil down to the concept of heavy-work investment (HWI). This is an umbrella term and has been reconceptualized to encompass two major core components: (1) investment of time (i.e., working long hours) and (2) investment of effort and energy (i.e., devoting substantial efforts, both physical and mental, at work) (Snir & Harpaz, 2012, 2015). These dimensions are respectively called time commitment (HWI-TC) and work intensity (HWI-WI). Although many studies have dealt with the implications of working overtime (e.g., Caruso, 2014; Rabenu & Aharoni-Goldenberg, 2017; Snir, 2018; Stimpfel et al., 2012), to the best of our knowledge, empirical studies regarding the investment of efforts at work as an indicator of HWI are scarce (e.g., Rabenu et al., 2019; Shkoler & Kimura, 2020; Tziner, Shkoler, et al., 2019). It is, therefore, one of the aims of the current research to address both of these core dimensions of HWI (i.e., “time”, HWI-TC, and “effort”, HWI-WI). In terms of “measurement”, Snir and Harpaz (2012) resurfaced and cleaned some dust off of an existing scale for HWI (see Brown & Leigh, 1996) and used it in their research (they also added two short and open-ended items). This scale was also used in other research involving HWI in several countries as it was also impacted by culture (Rabenu et al., 2019; Shkoler & Kimura, 2020; Shkoler, Rabenu, Vasiliu, et al., 2017; Tziner, Shkoler, et al., 2019). For further reading about the concept of HWI, kindly refer to Snir and Harpaz's (2015) book.
HWI is a higher order construct and is conceptually different from workaholism, presenteeism, and work engagement. For example, the workaholism concept implies addiction to work behaviors, such as working beyond expectations defined in one's role as an employee (e.g., Clark et al., 2016). While workaholism embodies heavy time investment, the concept does not go far enough as to include either the nature of these behaviors or the level of performance. Workaholism also has a negative connotation implying addictive attraction to one's work to the point of neglecting other life dimensions. Work engagement, on the other hand, is a generally positive concept defined as a “positive, fulfilling, work-related state of mind, characterized by vigor, dedication, and absorption” (Langelaan et al., 2006, p. 522). Even though work engagement takes into account higher levels of energy and commitment to work, it does not specify the intensity with which one conducts the work. Finally, HWI is different from presenteeism because presenteeism refers to someone being physically on the job but not fully functioning due to an illness or medical condition (Hemp, 2004). It has negative implications, such as potential productivity loss.
VUCA vs. COVID-19, in the International Context
Coronavirus and its related disease (COVID-19) had a break-out in China around the twilight of 2019, and has been spreading out to most of the world ever since (e.g., Li et al., 2020; Wu et al., 2020). At the time of composing this article the number of infected people worldwide has crossed the 108 million mark and is rising still to this day (https://www.worldometers.info/coronavirus/). This pandemic has impacted most life domains, and as such may also have affected the domain of heavy-work investment, as it changed the employee count and their working characteristics, such as: (1) working from home vs. from office (i.e., on-site traditional office work), using electronic virtual communication (EVC) like Zoom or Skype; (2) working parents taking care of children at home (including home-schooling) since the education system is closed or operating in a limited format; (3) increasing in home chores (e.g., cleaning and cooking) for those working from home; (4) prioritizing local markets over global markets since the lock of national borders; (5) increased job insecurity and unemployment rates since the COVID-19 pandemic infected business domains, such as transportation, aviation and tourism, entertainment, and fashion; (6) increased overwork in “essential” sectors like healthcare, pharma, and groceries (Rabenu, in press, ch. 1, 9; Rudolph et al., 2020; Sinclair et al., 2020).
Our research had actually begun prior to the coronavirus' pandemic all over the globe. We did manage to collect data from a few countries, but this has reached a significant halt due to the pandemic and the ensued chaos. However, although difficult logistically, very recently we were still able to collect more data from even more countries – all are widely different in their national cultural context (Hofstede, 1980, 2020). Data from (1) Israel, Japan, Romania, and the USA were collected before COVID-19's spread, and data from (2) Pakistan, Italy, Turkey, Brazil, and Germany were collected recently in midst of this pandemic. The opportunity to collect pre-pandemic and post-pandemic data and across a variety of countries will allow us to investigate differences across cultures before and during a crisis situation.
A cross-cultural point-of-view may yield interesting and vital results (Barrett & Bass, 1976) as culture, in this sense, may be defined as “common patterns of beliefs, assumptions, values, and norms of behaviour of human groups (represented by societies, institutions, and organisations)” (Aycan et al., 2000, p. 194). Thus, countries differ from one another in many aspects, and the most prominent example is cultural/national dimensions devised by Hofstede (1980, 1991). In essence, different countries display different cultural codes, norms, and behaviours, which may affect their market, work values, behaviours, and work investment. Evidently, investment at work is distinct for every country. For example, in Israel 14.7% of employees work very long hours (50 hours a week or more), higher than OECD's average, while the USA's average is below it (OECD, 2015). Accordingly, the annual hours worked in Israel are consistently higher than in the USA (OECD, 2013, 2017). For further reading on the specific data and other countries, see OECD (2013, 2015, 2017), Eurofound (2016), and Eurostat (2016).
Current Research and Contribution
The current study is comparative in nature. The comparison is two-fold, as both different cultural contexts and COVID-19's timing will be juxtaposed and scrutinized in the view of HWI. As such, we embark on a humble quest to:
Examine the psychometric properties of the current measure for HWI, its dimensionality and invariance across different cultures, and explore how demographic variables can affect the measurement properties of the construct.
Compare these properties across different countries (see Hofstede, 1980, 2020; Shkoler & Kimura, 2020).
Explore how demographics relate to HWI.
Investigate if and how the COVID-19 situation is associated with investment at work. However, this point should be taken with a grain of salt – as will be elaborated in the Discussion section.
The current paper is unique in its international ambition, and the world-wide context (investment at work in COVID-19's context), while focusing on statistics and comparisons of HWI across all these 9 different countries. We wanted to have a wide spectrum of countries that will represent countries below and above OECD's own average annual working hours. The choice of these countries (i.e., Israel, Romania, Japan, USA, Pakistan, Italy, Turkey, Brazil, Germany) was based on OECD's rankings of their working hours (i.e., average annual hours that employees actually worked). Specifically, considering OECD's “average” annual working hours is 1,726, Japan is ranked at 1,644 working hours, Italy at 1,718, Israel at 1,898, Germany at 1,386, the USA at 1,779, Brazil (which is not in OECD's data) is estimated at 1,433 (Insider, 2019; OECD, 2021), Turkey at 1,832 (OECD, 2013), Romania at 1,791 (Tempo Online, 2020), and Pakistani data (which is not in OECD's data) indicates that nearly 39% of the total employees worked excessive hours (i.e., more than 48 hours a week) (International Labour Organization, 2017).
Additionally, to the best of our knowledge, this is also one of the very few pioneering studies doing research on HWI in the context of COVID-19.
This research is mostly exploratory in nature and the “main hypothesis” of the current study focuses on differences and variance among different (1) countries, (2) demographics, and (3) “times” or “context” (i.e., “before” and “during” COVID-19's pandemic). All will have an effect on HWI.
It is of paramount methodological importance that we have written the Method and Results sections meticulously and rigorously, in order to ensure replicability of the research. However, we are also aware that the current research is filled with a great deal of data and statistical analyses. Thus, to facilitate readability, focus, and interest, we have summarized the main and important findings in the Discussion section.
Method
Participants
In order to reduce the impact of organization-specific culture, we collected data from various companies rather than from a specific company, in all countries. So, the research encompasses a total of 12 samples from 9 countries (total sample size, NT = 3,418). Seven samples were collected before COVID-19's initial spread: (1 + 2 + 3) Israel (three samples; n1 = 166, n2 = 242, n3 = 221), (4 + 5) Romania (two samples; n1 = 1,338, n2 = 335), (6) Japan (n = 171), and (7) the USA (n = 190). In addition, “very” recently we have added six more samples to our data in the midst of the pandemic: (8) Pakistan (n = 211), (9) Italy (n = 142), (10) Turkey (n = 141), (11) Brazil (n = 224), and (12) Germany (n = 37) (it should be noted that the sample in Germany is the smallest). Striking a cooperation with the participants was very difficult, as they ultimately proved to be mostly unresponsive. However, we deemed it important to retain this sample for statistical and comparative reasons, as suggested in Shkoler et al. (2021), where it was important to scrutinize small samples in tandem with a much larger one. Demographical and descriptive statistics for each sample are presented in Tables 1 and 2. These tables also contain the result of group difference tests, pointing at some demographic differences across samples and countries.
Table 1. Descriptive Statistics for the Qualitative Demographics
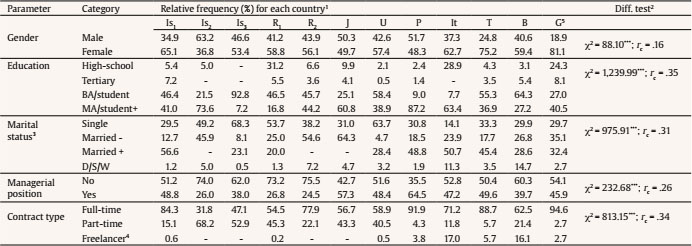
Note. 1The sequence of numbers here depicts the samples/countries as follows: Is1 + Is2 + Is3 = Israel; R1 + R2 = Romania; J = Japan; U = USA; P = Pakistan; It = Italy; T = Turkey; B = Brazil; and G = Germany; 2diff. test = chi-square difference tests to compare the demographical data based on the different countries. 3Married - = married/informal union “without” children; married + = married/informal union with children; D/S/W = divorced / separated / widowed; 4freelancer or self-employed; 5Germany has N = 37.*p < .05, **p < .01, ***p < .001.
Table 2. Descriptive Statistics for the Quantitative Demographics

Note. 1The sequence of numbers here depicts the samples/countries as follows: Is1 + Is2 + Is3 = Israel; R1 + R2 = Romania; J = Japan; U = USA; P = Pakistan; It = Italy; T = Turkey; B = Brazil; and G = Germany; 2diff. test = one-way ANOVA difference tests to compare the demographical data based on the different countries.*p < .05, **p < .01, ***p < .001.
The current study was correlational, based on a survey, without any manipulation on human subjects. At the beginning of each questionnaire, the general goal of the research was explained. Informed consent was obtained individually from all participants included in the study. We ensured maximum anonymity and discretion of the results, and also ensured the subjects knew they could leave and stop their participation at any time they choose to do so.
However, In Japan and in the USA the procedure of this study was approved by the IRB/Ethics Committee. The committee approved that this study does not contain ethical flaws like leaking of private information and inhumane questions in the questionnaire. All subjects gave written informed consent regarding the purpose of research, that of data collection, and the privacy protection method.
Measures
The items of the questionnaire were initially written in English and then translated into other languages (in Turkey, Pakistan, and Germany measures were administered in English), utilizing the back-translation procedure (Brislin, 1980).
Demographic items were asked to draw basic information about the participants and, hence, the different countries: (1) gender, (2) age (in years), (3) education, (4) marital status, (5) job tenure (in years), (6) managerial position (no/yes), (7) contract type, and (8) country of residence. Kindly refer to Appendix A for full demographical items from the survey.
Heavy-Work Investment (HWI; based on Brown & Leigh, 1996) was tapped by 10 Likert-type items ranging from 1 (strongly disagree) to 6 (strongly agree), 5 items for each dimension, namely, “time commitment” (HWI-TC; e.g., “Few of my peers/colleagues put in more weekly hours to work than I do”; items 1-5) and “work intensity” (HWI-WI; e.g., “When I work, I really exert myself to the fullest”; items 6-10), respectively. Descriptive statistics, including reliability coefficients, will be presented in the Results section. The full item list in different languages can be viewed in Appendix B (it should be noted that Urdu and Turkish versions were “not” back-translated, but nevertheless we decided to include these translated versions for scholars and practitioners who wish to use it in their native language).
Procedure
For the first Israeli sample, a pencil-and-paper research survey was distributed. However, all other samples were collected by using the online survey system of Google Forms. The samples that were gathered during the COVID-19 pandemic had their data collected between 12th and 25th of June, 2019. We assured anonymity and discretion of participants and data derived from the research and included a conscious consent question at the beginning of the survey asking for their agreement to participate. No incentives were given whatsoever to the participants for their cooperation. The link to the survey was administered via SNS (social networking service) method, such as LinkedIn, emails, etc. Data was analyzed using SPSS (v. 23) software package and AMOS (v. 23) software package.
Control Variables
As per Tables 1 and 2, we can see significant differences across all countries, and as such we included them as covariates in further analyses. This is in order to control their potential influence on further analyses so that we may reduce the statistical artefacts that could potentially rise from said differences. In other words, in these analyses we controlled for the effects of gender, age, education, marital status, tenure, managerial position, and contract type. This is relevant only for Tables 6-8 in the Results section.
Common-Method Bias
The full explanation and analyses of common-method bias are presented in Appendix C.
Confirmatory Factor Analyses (CFA)
Table 3a displays the 10 items of HWI measure, “divided” to its dimensions (as was mentioned, HWI-TC and HWI-TC), CFA factor loadings, and other statistics. For future references and researchers who wish to view the full scale (in English, Portuguese, Hebrew, Japanese, Italian, Romanian, Urdu, and Turkish), kindly refer to Appendix B at the end of the paper.
Table 3a. CFA Factor Loadings of HWI's two Dimensions, for Each Country
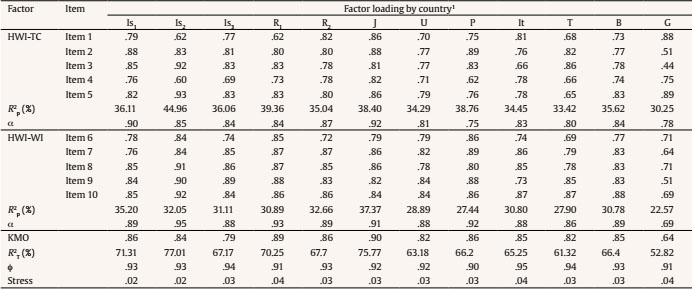
Note. The CFA was employed in the principle component analysis method with Varimax rotation (eigenvalue condition > 1, factor loadings must be > .45). HWI-TC = Time Commitment dimension of HWI. HWI-WI = Work Intensity dimension of HWI. KMO = Kaiser-Meyer-Olkin measure of sampling adequacy. 1The sequence of numbers here depicts the samples/countries as follows: Is1 + Is2 + Is3 = Israel; R1 + R2 = Romania; J = Japan; U = USA; P = Pakistan; It = Italy; T = Turkey; B = Brazil; and G = Germany; α = Cronbach's alpha reliability coefficient. p = partial extracted variance; T = total extracted variance; Φ = Burt-Tucker's congruence coefficient; stress = normalized raw stress.
Table 3a shows outstanding CFA results, indicating strong construct structure. The congruence coefficients also indicate fair similarity, but “not” equality, between HWI's dimensions (because “... a value in the range [0.85-0.94] corresponds to a fair similarity, while a value higher than 0.95 implies that the two factors or components compared can be considered equal”; Lorenzo-Seva & ten Berge, 2006, p. 57). In addition, using AMOS we also concluded that the general hypothesis for structural invariance across groups (i.e., countries) is rejected, assuming that the structural covariances model is correct: Δdf = 143, Δχ2 = 1,164.84, p = .000. This result indicates that HWI-TC and HWI-WI are structurally variant across countries.
Furthermore, we also employed full convergent and discriminant validity analyses. To this end, we used a statistical tool developed by Gaskin and Jim (2016). Table 3b portrays the findings, whereas: (1) CR = composite reliability, (2) AVE = average variance extracted, (3) MSV = maximum shared squared variance, (4) MaxR(H) = maximum reliability, (5) statistics “below” the diagonal on the right side of the table = zero-order Pearson correlation coefficients, (6) bolded statistics “on” the diagonal at the right side of the table = square-root of the AVE.
As seen in Table 3b, there are no validity concerns. Convergent and discriminant validities were achieved and supported (for further reading, see Hu & Bentler, 1999).
Results
First, we explored descriptive statistics and zero-order associations between variables per country. These results are displayed in Tables 4 and 5.
Table 4. Basic Descriptive Statistics for HWI's Dimensions, for Each Country

Note. 1The sequence of numbers here depicts the samples/countries as follows: Is1 + Is2 + Is3 = Israel; R1 + R2 = Romania; J = Japan; U = USA; P = Pakistan; It = Italy; T = Turkey; B = Brazil; and G = Germany.
Table 5. Zero-order Pearson Correlation Coefficients for HWI's Dimensions, per Country

Note. 1The sequence of numbers here depicts the samples/countries as follows: Is1 + Is2 + Is3 = Israel; R1 + R2 = Romania; J = Japan; U = USA; P = Pakistan; It = Italy; T = Turkey; B = Brazil; and G = Germany. HWI-TC = Time Commitment dimension of HWI; HWI-WI = Work Intensity dimension of HWI; HWI-T = Total HWI construct; 2Germany has N = 37; 3All correlations are statistically significant at p < .001.*p < .05, **p < .01, ***p < .001.
Table 5 indicates that: (1) the two dimensions (HWI-TC and HWI-WI, respectively) are mostly correlated with one another, but (2) they do reflect their total construct (HWI) to a greater extent. In conjunction with the results of the CFA and validity analyses (Tables 3a and 3b), we can see these composites are quite stable across samples and “time” (as there were samples before and “during” the COVID-19 pandemic, also see Further Analyses section)
Further, a one-way MANCOVA test for the differences across countries on the two dimensions of HWI was employed (as the two HWI dimensions are theoretically and statistically related), with the control variables mentioned in the Method section (i.e., gender, age, education, marital status, tenure, managerial position, and contract type). The “multivariate” F-test proved to be statistically significant: MF(2, 6818) = 35.82, p = .000, Wilk's λ = .80, indicating a generic difference across samples. The specific (univariate) F-tests are presented in Table 6. The specific-group differences are displayed in Tables 7 and 8.
Table 6. MANCOVA Univariate Analyses and Descriptive Statistics for HWI's Dimensions

Note. 1The sequence of numbers here depicts the samples/countries as follows: Is1 + Is2 + Is3 = Israel; R1 + R2 = Romania; J = Japan; U = USA; P = Pakistan; It = Italy; T = Turkey; B = Brazil; and G = Germany; 2diff. test = specific F-tests from one-way MANCOVA.*p < .05, **p < .01, ***p < .001.
Table 7. Specific-group Differences for HWI-TC Based on MANCOVA Analysis
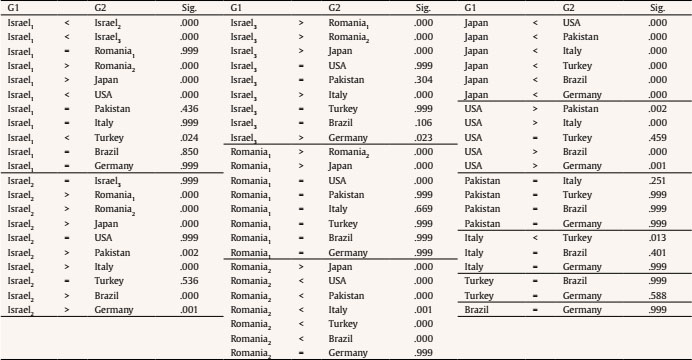
Note. G1 = group 1/country 1. G2 = group 2/country 2; specific-group differences are based on Bonferroni's adjustment for multiple comparisons.
Table 8. Specific-group Differences for HWI-WI Based on MANCOVA Analysis
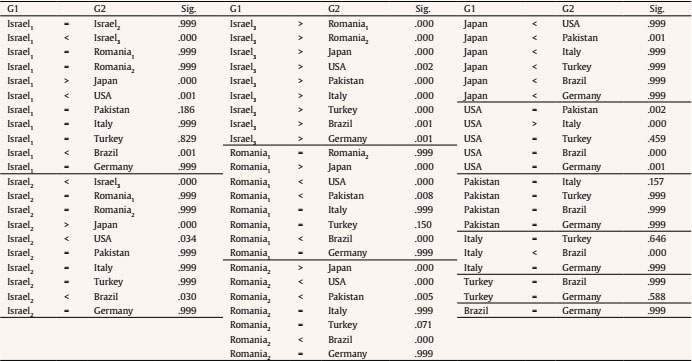
Note. G1 = group 1/country 1; G2 = group 2/country 2; specific-group differences are based on Bonferroni's adjustment for multiple comparisons.
Tables 6-8 teach us that these 9 countries exhibit different levels of heavy-work investment of time and effort, even after controlling for the effects of mentioned covariates.
Further Analyses and Demographical Differences
The results of prior analyses indicate that there are indeed various differences across the several countries examined in this research, almost regardless of sample size (the largest is Romania1 with n = 1,338, and the smallest is Germany with n = 37). However, reading results from 12 difference samples may prove to be quite an ordeal. Ergo, in this section we will repeat a few cardinal analyses and add some more, while the prime concern here is distinguishing between “only two distinct groups” of samples: (1) “before” COVID-19 pandemic (consisting of 7 samples: Israel (1 + 2 + 3), Romania (1 + 2), Japan and the USA; n = 2,666), and (2) “during” COVID-19 pandemic (consisting of 6 samples: Pakistan, Italy, Turkey, Brazil, and Germany; n = 766). The analyses in this section are mainly focused on: (a) zero-order correlations and, more importantly, (b) group differences with demographics via “two-way” MANCOVAs.
As for the correlations, the analyses produced the following: (1) the association between HWI-TC « HWI-WI is r = .42, p < .001 (for the “before” COVID-19 group) and r = .36, p < .001 (for the “during” COVID-19 group), and by using Fisher's Z we can conclude that there is a barely significant difference between these two relationships (Z = 1.72, p = .043); (2) the association between HWI-TC « HWI-T (i.e., “total” HWI construct) is r = .87, p < .001 (for the “before” COVID-19 group) and r = .88, p < .001 (for the “during” COVID-19 group); (3) the association between HWI-WI « HWI-T (i.e., “total” HWI construct) is r = .79, p < .001 (for the “before” COVID-19 group) and r = .75, p < .001 (for the “during” COVID-19 group).
Furthermore, as for comparative analyses, the aforementioned MANCOVA tests are performed similarly to previous analyses – also controlling for demographics – but here in each analysis one demographic is picked from the list, which will be included as a fixed factor/predictor in tandem with the group factor (before/during), while the other demographic parameters remain as covariates. The results are shown in Tables 9-13.
Firstly, in order to observe the generic difference between the two groups (before COVID-19 and during COVID-19) with no interaction between them and other demographics, a one-way MANCOVA was calculated. The analysis yielded is a significant “multivariate” effect of the groups, MF = 17.56, p = .000, Wilk's λ = .99. There were also two significant “univariate” effects (1) on HWI-TC, F(1, 3420) = 7.16, p = .008, η2p = .01, and (2) on HWI-WI, F(1, 3420) = 35.04, p = .000, η2p = .02. Further, specific-group differences (based on Bonferroni's adjustment for multiple comparisons) show that: (a) the “during” COVID-19 (M = 3.67, SE = 0.05) group exhibits “higher” HWI-TC than the “before” COVID-19 group (M = 3.53, SE = 0.02), p = .008, and (b) the “during” COVID-19 (M = 5.07, SE = 0.04) group also exhibits “higher” HWI-WI than the “before” COVID-19 group (M = 4.82, SE = 0.02), p = .000.
Secondly, regarding the two-way MANCOVA analyses, Table 9 displays the adjusted-for-covariates means and standard errors for each analysis, groups, and sub-groups. Table 10 portrays multivariate F-tests and specific F-tests for each dependent variable (one for HWI-TC and one for HWI-WI). Specifically, there is one multivariate F-test and three univariate F-tests (one for the main effect of the sample groups, before vs. during, one for the main effect of the demographical parameter, and one for the interaction effect of both of these factors). Tables 11-13 depict specific-group differences (based on Bonferroni's adjustment for multiple comparisons), for each analysis. In total, there are five MANCOVA analyses, one for each sample group (before/after) × demographic parameter, based on gender, education, marital status, managerial position, and contract type. Interaction univariate F-tests were performed “only” when the multivariate F-test has proved to be statistically significant.
Table 9. MANCOVA Univariate Analyses and Descriptive Statistics for HWI's Dimensions
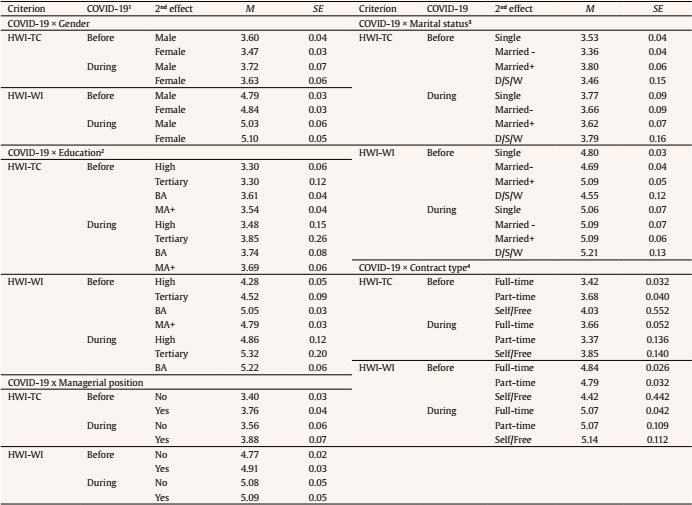
Note. 1COVID-19 groups = (a) “before” COVID-19, (b) “during” COVID-19. 2Education (high = high-school; BA = have BA/BA student; MA+ = have MA/MA student and above). 3Marital status (married- = married/informal union “without” children; married+ = married/informal union “with” children; D/S/W = divorced/separated/widowed). 4Contract type (full = full-time employee; part = part-time employee; self/free = self-employed/freelancer).
Table 10. MANCOVA Multivariate and Univariate Statistical Tests

Note. Interaction = interaction effect between the two main effects (for example: COVID-19 × Gender). 1COVID-19 groups = (a) “before” COVID-19, (b) “during” COVID-19. 2Gender (1 = male, 2 = female). 3Education (1 = high-school, 2 = tertiary/technical, 3 = have BA/BA student, 4 = have MA/MA student and above). 4Marital status (1 = single, 2 = married/informal union without children, 3 = married/informal union with children, 4 = divorced/separated/widowed). 5Managerial position (1 = No, 2 = Yes). 6Contract type (1 = full-time employee, 2 = part-time employee, 3 = self-employed/freelancer).
To facilitate readability, focus and understanding of the results, here we summarize the results from Table 9 and the group-specific analyses (for main effects and interactions, when applicable) based on Bonferroni's adjustment for multiple comparisons. It should be noted that if there are no statistically significant differences, they will not be written as to minimize the reading load:
Results from analyses of COVID-19 × gender: the “during” COVID-19 group exhibits “higher” HWI-TC than the “before” COVID-19 group (p = .010). The “during” COVID-19 group exhibits “higher” HWI-WI than the “before” COVID-19 group (p = .000). Males exhibit “higher” HWI-TC than females (p = .037).
Results from analyses of COVID-19 × education: the “during” COVID-19 group exhibits “higher” HWI-TC than the “before” COVID-19 group (p = .004). The “during” COVID-19 group exhibits “higher” HWI-WI than the “before” COVID-19 group (p = .000). Those with BA (or BA students) exhibit “higher” HWI-TC than those with full high-school education (p = .011). Those with full high-school education exhibit “lower” HWI-WI than those with tertiary/technical education (p = .036), those with BA (or BA students) (p = .000), and those with MA (or MA students) (p = .000). Those with BA (or BA students) exhibit “higher” HWI-WI than those with MA (or MA students) (p = .000). The interaction effect's results (COVID-19 × education) are displayed on Table 11.
Table 11. COVID-19 × Education Group Differences for HWI-WI

Note. INT = interaction (combination of the two main effects' groups); 1before = before COVID-19 group; 2during = during COVID-19 group. Tertiary = tertiary/technical education; BA = have BA or a BA student; MA = have MA or a MA student.
As can be seen in Table 10, there are clear differences in most “before” groups, though there are none in the “during” group which hints at the effects of COVID-19 pandemic. Also, we can see that, in general, the “during” group exhibit “higher” HWI-WI than the “before” group, regardless of education (apart from BA).
Results from analyses of COVID-19 × marital status: the “during” COVID-19 group exhibits “higher” HWI-TC than the “before” COVID-19 group (p = .012). The “during” COVID-19 group exhibits “higher” HWI-WI than the “before” COVID-19 group (p = .000). Participants who are married/informal union “with” children exhibit “higher” HWI-TC than those who are married/informal union “without” children (p = .028). Participates who are married/informal union “with” children exhibit “higher” HWI-WI than those who are single (p = .030), and those who are married/informal union “without” children (p = .002). The interaction effect's results (COVID-19 × marital status) are displayed on Table 12.
Table 12. COVID-19 × Marital Status Group Differences for HWI-WI

Note. INT = interaction (combination of the two main effects' groups); 1before = before COVID-19 group; 2during = during COVID-19 group; married- = married/informal union “without” children; married+ = married/informal union “with” children; D/S/W = divorced/separated/widowed.
As can be seen in Table 11, there are clear differences in half of the “before” groups, though there are none in the “during” group which hints at the effects of the COVID-19 pandemic. Also, we can see that, in general, the “during” group exhibit “higher” HWI-WI than the “before” group, regardless of marital status (apart from married/informal union “with” children).
Results from analyses of COVID-19 × managerial position: the “durin”g COVID-19 group exhibits “higher” HWI-TC than the “before” COVID-19 group (p = .008). The “during” COVID-19 group exhibits “higher” HWI-WI than the “before” COVID-19 group (p = .000). Managers exhibits “higher” HWI-TC than the non-managerials (p = .000).
Results from analyses of COVID-19 × contract type: the “during “COVID-19 group exhibits “higher” HWI-WI than the “before” COVID-19 group (p = .009). The interaction effect's results (COVID-19 × marital status) are displayed in Table 13.
Table 13. COVID-19 × Contract Type Group Differences for HWI-TC

Note. INT = interaction (combination of the two main effects' groups); 1before = before COVID-19 group; 2during = during COVID-19 group; full-time = full-time employee; part-time = part-time employee; self-free = self-employed/freelancer.
As can be seen in Table 12, there is only one significant difference of the “before” groups, though there are none in the “during” group which hints at the effects of the COVID-19 pandemic. Also, we can see that, in general, the “during” group exhibit “higher” HWI-TC than the “before” group, regardless of contract type (apart from self-employed/freelancers).
Discussion
The aims of the current statistical and comparative paper were to (1) shed light on the psychometric properties of the relatively “new” construct of Heavy-Work Investment (Snir & Harpaz, 2012, 2015), and its two dimensions – Time (HWI-TC) and Effort (HWI-WI), (2) assess differences across 9 countries in relation to HWI, (3) gauge the effect that demographical parameters may have on HWI, and (4) investigate the interaction between said demographics and the sample groups (i.e., “before” the COVID-19 pandemic, and “during” the COVID-19 pandemic). Our current research adheres to the very few, and extant, studies which tested and validated Brown and Leigh's (1996) HWI measure (e.g., Rabenu et al., 2019; Shkoler & Kimura, 2020; Shkoler, Rabenu, Vasiliu et al., 2017; Tziner, Buzea et al., 2019). As was mentioned, to the best of our knowledge, this is also one of the very few pioneering studies that research HWI in the context of COVID-19. In addition, our research hypotheses were supported to a great extent, with interesting results. However, as the paper has some long and complex analyses, here are the main findings:
(1) HWI is a stable construct across different countries with different backgrounds; (2) HWI is best interpreted by its “dimensions” (HWI-TC, Time Commitment and HWI-WI, Work Intensity) and not as a standalone construct; (3) the total extracted variance by the two dimensions is remarkably high but not perfect, hinting at something “missing” from the total construct (see Future Research section for more details); (4) HWI-TC and HWI-WI correlate with one another, but they do “not” exhibit a very strong association, indicating fair convergent validity, yet they are discriminated from measuring the same construct; (5) even after “controlling” for the effects of all the demographical parameters (i.e., gender, age, education, marital status, tenure, managerial position, and contract type), we found significant effects on HWI-TC and HWI-WI, by country affiliation, time-group affiliation (“before” and “during” the COVID-19 pandemic), and all the demographics (as was explained under the Further Analyses section); (6) in general, employees “during” the COVID-19 pandemic report “higher” HWI-TC and HWI-WI than workers “before” the pandemic; (7) demographics interact with the group of samples “before” the COVID-19 pandemic, but they do “not” interact with the “during” the pandemic group. In other words, demographics do “not” differentiate between employees in the “during” the pandemic group, but they mostly distinguish between workers “before” the pandemic (this finding has been proven to be stable/invariant across most of the demographical interactions).
Of note, the initial impact of the COVID-19 crisis on OECD labor markets, where data are available, has been ten times larger than that observed in the first months of the 2008 global financial crisis (taking into account both the drop in employment and the reduction in hours worked among those who remained in work, total hours worked fell by 12.2% in the initial three months compared to 1.2% in 2008). This reflects the special nature of the COVID-19 crisis with many countries having put entire sectors of their economy “on hold” to contain the spread of the virus (e.g., OECD, 2020, 2021). Nonetheless, our findings show that we still face heavier work investment in both time and efforts that ostensibly contradict OECD's deduction. However, it is not a complex issue. Although unemployment rates rose to unprecedented extremes very sharply (e.g., OECD, 2020), those who “remained employed” during the pandemic had to work harder and put extra efforts in their jobs. In other words, generally, on the macro-level, working hours may have been diminished, but surgical observation portrays a different picture on the micro-level. Hence, it is reasonable to assume that HWI maybe be dependent on the industry or job types (e.g., medical teams, food deliveries, managers, the third sector and others were able to continue working during the pandemic, either from office or from home) and hence our findings do not reflect and represent the entire population (see Limitations and Future Research Recommendations section).
A plausible explanation to the finding that people invested more at their work “during” the pandemic, as opposed to “before” it, is their need to “prove” themselves at work from fear of being fired or of otherwise losing their job (of which, the financial consequences could be dire).
However, there is another alternative explanation. We capitalize on the fact that people might deliberately engage in excessive work in order to assuage some negative feelings, like guilt (e.g., Ng et al., 2007). We, therefore, postulate that the same notion is also relevant in the current research's situation. People might exert themselves more at work as an element of distraction from adversities, difficulties, and stress which permeate our lives in COVID-19 pandemic.
Other explanations may also apply, such as: (1) a situation when only one person (in a relationship with a partner) gets fired from his/her job, while the other has to work extra hard to sustain their financial state, (2) in mid-pandemic, healthcare systems and organizations occupied their employees (e.g., medical doctors and nurses) around the clock due to the virus.
All these lead us to understand that the effects that COVID-19 has on HWI may vary, depending on other parameters. We elaborate further in the next section.
Limitations and Future Research Recommendations
While our study has strength in the newness of findings, the use of several different international samples, and the COVID-19's research aspect, we must also count its limitations. First, our data is cross-sectional and single-sourced, despite the many samples and the “time” notion (before COVID-19 vs. during COVID-19). This limits the generalizability of the research and does not let us see if the findings are stable across time. Although it may not be a major limitation, our research was not focused on a specific industry, sector, or types of workers (e.g., high-tech, low-tech, services, marketing, and sales). While this bolsters the external validity of the research, it limits the construct validity of the results.
Due to logistical difficulties, the current research only included the HWI measure and did not test this with other constructs, such as job engagement and workaholism (for convergent validity) or ethical climate and work-family conflict (for discriminant validity). As such, we suggest a replication (even on a smaller scale of countries/samples) with more measures to compare.
To expand our understanding of cultural difference, we recommend replicating our study in other countries with cultural similarities (or differences) to the those used in this research, to broaden the generalizability, credibility, and validity of our findings. Although this study includes 9 countries, this might still limit the generalizability of the results to a certain extent. We, therefore, suggest scholars worldwide to replicate our research in other countries to generalize and expand our findings. Furthermore, in future international comparative studies, researchers can explore why and how each country's cultural and institutional components influence the differences that would exist between countries.
Nevertheless, although the HWI is invariant across countries, contextual conditions may not be, meaning that the samples were collected in different countries in “pre-COVID-19” and “during-COVID-19”, and we could neither account for the potential differences, across countries, in response to the pandemic, nor could we gauge it.
A general suggestion to researchers worldwide is to conduct longitudinal studies, and this is highly relevant in the face of adversities or big changes (such as the COVID-19 pandemic). The current research would have benefited from “pre, mid, post” research design (i.e., before, during, and after COVID-19 pandemic). However, because of logistical and time constraints, we were unable to proceed with this research design.
It is also safe to assume that the findings discovered in the research would be dependent on which industry we focus on (e.g., high-tech, low-tech, marketing, service), and as such we would also recommend incorporating this element in future research.
As was mentioned at the beginning of the Discussion section, the total extracted variance by the two dimensions of HWI is remarkably high but not perfect, hinting at something “missing” from the total construct. HWI is divided to the investment of (1) time, (2) cognitive efforts, and (3) physical efforts at work. Based on Shkoler, Rabenu, Vasiliu, et al.'s (2017) work and suggestions, we can conclude and, therefore, suggest adding a new element, a new dimension to HWI – the “emotional” aspect (see also, Krasulja et al., 2017; Toubiana et al., 2017; Voronov & Weber, 2017). This will necessitate constructing a new sub-scale for HWI and passing it through standardized validation processes. We believe that this emotional dimension will enrich the current HWI construct theoretically, methodologically and statistically. This notion is even more pertinent at times of uncertainty, such as a worldwide pandemic.
Furthermore, we highly recommend approaching this research through a “qualitative” point of view. A quantitative scale may not reveal intimate and personal narratives or experiences, which can enhance our findings and augment our understanding of this phenomenon (e.g., Shkoler, 2019).
Moreover, we propose investigating two other important and interesting venues. Firstly, whether the work itself is done while sitting (sedentary) or not. There has been an increase in physical inactivity and sedentary behaviors with the advent of the COVID-19 pandemic (e.g., Cheval et al., 2020; Hall et al., 2020). Part of the reasons is the increased use of EVC for work (e.g., Zoom) that has decreased workers' ability to engage in physical activities during a regular work day (for example, teachers and lecturers working remotely, using EVC, rather than walking around the classroom). As per our findings, employees worked more hours during the pandemic as opposed to before it, in pre-pandemic situation. We suggest further research in this regard to further explore not only if a worker is a heavy work investor, but also if his/her job is sedentary – all in order to understand various health implication for the employees (see also Ozemek et al., 2019; Rabenu, in press; Sanchez-Villegas et al., 2008; Thorp et al., 2011).
Secondly, whether the employee is a parent to adolescent children or not (regardless of marital status). As mentioned before, because children were present at home, the load/burden that workers, who are also parents, had to bear was greater than those without children to take care of and worry about. This fact almost invariably affects these parenting employees' abilities and availability to work beyond formal working hours and to exert more efforts at work, in tandem with taking care of and occupy their children.
Last but not least, we discussed the effects of the COVID-19 pandemic and the cultural contexts of our research. We can see that the context might be more impactful than the pandemic situation itself. As such, it is highly interesting to explore and further research whether this pandemic “left a mark” on HWI culture in the countries scrutinized in the current paper and in the world in general. In other words, future research (OECD included) may benefit from investigating HWI after the pandemic is over, in order to see whether the heavy-work investment culture (like in Israel and Japan) changed, and in what direction and strength.
Finally, we wish to emphasize that this research was “half”-exploratory and not theory-focused, but methodologically- and statistically-driven. Baseline a-priori differences between cultures and between times (before vs. during COVID-19) were indeed proposed. However, there were no in-depth and specific formulation of confirmatory hypotheses. Nevertheless, the findings of the research proved to be very interesting and highly relevant to the current challenging times we live in (i.e., COVID-19). As such, we call out to the academic community and scholars worldwide to proceed with confirmatory studies based on the ideas generated and conclusions raised from the current paper.