Introduction
Workplace absenteeism is one of the current primary concerns at economic, social and business levels, as the absence of an employee from their post, in its different forms and causes, leads to production losses, changes the organisational environment and generates increased cost to the public Social Security system (Hassard, Teoh, Visockaite, Dewe, & Cox, 2018; López-Alonso, Ibarrondo-Dávila, & Rubio-Gámez, 2015; Mazzoni et al., 2019). In Spain, absenteeism due to temporary incapacity (work-related and non-work-related) accounts for 13.09 lost days for every active worker, one of the highest levels on an international scale (Blasco de Luna et al., 2018). When it comes to absenteeism due to common illness, the data is even more negative. According to the 7th Adecco Report on absenteeism, in 2017 86.4% of cases of temporary incapacity were related to cases of common illness, with an average duration of 17.21 days lost (see Blasco de Luna et al., 2018). This has led to an increase of 6% in comparison with 2016. The increased cost of absenteeism, added to the personal and social repercussions it can have on organisations, has meant its control is one of the primary strategic objectives of any business, region or state (Martin-Fumadó, Martí Amengual, Puig Bausili, & Arimany-Manso, 2014; Martín-Román, & Moral, 2017).
Absenteeism is regarded by several authors as a multi-dimensional process (Altunkaynak, 2018; Khashaba, El-Helaly, El-Gilany, Motawei, & Foda, 2018; Thurston, & Glendon, 2018), which has been explained as much by individual factors, such as age (Fernández-García, 2013), gender (Campos-Serna, Ronda- Pérez, Artazcoz, & Benavides, 2012), the employee’s nationality (Casey, Riseborough, & Krauss, 2015) or the consumption of alcohol (Luján, Santana, Alemán, & Sánchez, 2014), as by the consequences of the various social, economic and organisational conditions to which the employee is exposed in their work environment (Boada i Grau, De Diego Vallejo, Agulló Tomás, & Mañas Rodríguez, 2005). In respect of working conditions, a great number of studies highlight the relationship between absenteeism and different organisational variables, such as salary, job security and contract type (de Menezes, & do Monte, 2013). In particular, it is observed that people with a poorer state of health, and therefore more of a propensity to calling in sick, are those workers with temporary contacts and who are exposed to the worst working conditions (Virtanen et al., 2005).
Job insecurity, characterised by instability, uncertainty and lack of guarantees on working conditions, has been used as a valid indicator for the analysis of absenteeism, as it has already been identified as one of the risk factors (Jiang, & Lavaysse, 2018) and factors of work attendance (Bogaerts, De Witte, Verlinden, & Vermeulen, 2017). At the same time, differences in recruitment terms may also be determinants in absenteeism (García, Green, & Navarro Paniagua, 2018; Restrepo, & Salgado, 2013). The studies specifically place particular importance upon contract type, as higher rates of absenteeism have been evidenced in employees with casual contracts than in those with permanent contracts (Zaballa et al., 2016). Similarly, these studies indicate that the length of the working day stated in the employment contract shows a close link with levels of absenteeism and the health of employees (Benavides, Benach, Diez- Roux, & Roman, 2000). For example, employees working temporary partial days manifest higher rates of job dissatisfaction and symptoms of musculoskeletal disorders (Benavides, & Benach, 1999).
Objetives and Hypothesis
Under the aforementioned frame of reference, the aim of this study is to analyse the variables of a contractual nature which have an influence on workplace absenteeism. The intention is thus to provide a description of which recruitment factors (type of contract and work day, contract term, longevity and illnesses suffered in recent years and their duration) may influence the propensity to call in sick in the workplace environment.
This primary objective is detailed through different employment scenarios:
Are employees on a temporary contract most likely to experience temporary incapacities arising from non-work-related occurrences?
Does the working day have an influence on the likelihood of experiencing further temporary incapacity due to non-work-related occurrences?
Is there a link between longevity in a post and the likelihood of experiencing temporary incapacity due to non-work-related occurrences?
Are temporary incapacities experienced in recent years a potential predictor variable of present-day absenteeism?
Method
Participants
The sample is of an incidental and non-probabilistic nature and is made up of the full complement of staff hired in 2017, being 5524 people in a Spanish company in the Services sector, engaged in maintenance and cleaning tasks. Table 1 shows the characteristics of the sample with regard to its different recruitment methods.
At the same time, data was obtained for each employee relating to their absenteeism due to common illness in 2017. There was particular focus on the number of cases of common illness which had been experienced and the number of sick days taken this year.
In the development of the study, the term common illness shall equate to the concept of sickness.
Variables
On account of the above, the variables which have been used (see Table 2) correspond, on the one hand, to information about employee recruitment in 2017, (type of contract and work day, contract term in 2017 and longevity), and on the other hand, on the number of periods of sick leave taken by each employee in the last 3 years (2015, 2016 and 2017), and their duration. The ratio of absenteeism due to illness was also calculated, with the result obtained by dividing the number of sick days by the number of contracted days in 2017.
Procedure
The process for obtaining the data was completed using the ERP (Enterprise Resource Planning) software in the organisation with which the study is concerned. The statistical control of workplace absenteeism was developed using Navision and Meta4 computer applications, into which all data relating to absences due to the employees’ non-work-related and work-related illnesses was inputted. Similarly, all sociodemographic and contractual variables that have formed part of this study were included in both applications.
The introduction of the information relating to absenteeism was done by the Human Resources department of the aforementioned organisation. Likewise, the department for Occupational Risk Prevention conducted an investigation of all cases of accidents at work and work-related illness and the statistical analysis of absenteeism arising from both.
Data Analysis
Firstly, a descriptive analysis of the data (frequency and percentages) was produced by way of contingency tables. Furthermore, the Chi-squared test was used for contingency tables with two independent samples, with the aim being to determine the existence of significant differences between the groups being considered. Finally, the CHAID (Chi-squared Automatic Interaction Detection) (Kass, 1980) method was used, which, as is known, is a non-parametric technique for analysing the segmentation of variables and it is suitable for large samples and categorical dependent variables, as is the case here, which enables the grouping of elements described by a dependent variable according to the values of other independent variables (predictors) (Ortega, 2015).
The CHAID method analyses all the values of each potential predictor variable by using the Chi-squared statistical test, which reflects how similar or connected the variables are. From there, it selects the most significant predictor in order to form the main partition in the decision tree, in such a way that each node is comprised of those categories which are similar to the selected variable. The process continues successively until the tree is complete (Sanz, & Ponce de León, 2010).
In this kind of inductive model, it is essential that the way in which the tree’s structure behaves is assessed in order to generalise the available data in a sample of different data. The technique most employed for this validation involves dividing the data into a training sample and a validation or test sample. If the model generated with the training data shows a predictive capability similar to that of the validation data, the rules may be generalised. The tree is assessed with the confusion matrix which shows the proportion of cases correctly categorised by the algorithm in relation to the whole of the categorised sample and the proportion of inaccuracies.
Selecting the optimal tree is related to the problem of overfitting. Usually, the greater the complexity the greater the overfit. In the method applied to this study, ‘self-pruning’ parameters were set which prevented the overfix and generated trees with little complexity (only three levels of division and ‘child’ nodes with more than 100 individuals), which provided a stable model with few differences between the training sample and the test.
This technique was chosen due to its good results both in normal populations (Peñalva-Vélez, & López-Goñi, 2014) and clinical populations (López-Goñi, Fernández- Montalvo, & Arteaga, 2012).
All the analyses were carried out using the IBM®-SPSS Statistics® package.
Results
First of all, the interrelations between the number of cases of illness which occurred in the last three years (2015, 2016 and 2017) and the variables of contract type, work day type and contract term (see Figure 1) were examined. To analyse these types of comparative data, the size of each group was divided by the total number of individuals. The number of contracted months was also taken into consideration in order to eliminate the effect of exposure time, where possible, in such a way so as the data is relative to one working month.

Note: Group = ABC, where A is the Type of contract (0 = Permanent, 1 = Temporary), B is the Type of work day (0 = Full, 1 = Partial) and C is Months worked in the year (1 < = 2 months, 2 < = 8 months, 3 > 8 months).
Figure 1. Percentage of cases of illness in each group by month worked.
As can be seen, the number of cases of illness in 2017 holds the same distribution between groups as in the two previous years. The existing difference stands out between the high values of the first half of the chart (permanent contract) compared with the low values in the second half (temporary contract). It is also noted that the groups on contracts of a duration shorter than two months represent a greater proportion of people with a case of illness than those of a longer duration.
If these results are compared with the average ratio of illness in each group, clear variations are observed with regard to the groups on a contract shorter than two months. This means, as can be observed in Figure 2, that the groups with short term contracts represent a greater proportion of people with a case of illness in 2017, but with a ratio of sick days lower than the groups on a longer recruitment term.
Secondly, four Chi-squared tests were carried out on the Group variable in Figures 1 and 2 in comparison with each of the considered variables (17Ill01_Case, 16Ill01_Case, 15Ill01_Case and Ill01_Ratio). In all cases significant differences were obtained between the different groups being considered (Table 3).
Table 3. Chi-squared tests of the Group variable in comparison with the variables 17Ill01_Case, 16Ill01_Case, 15Ill01_Case and Ill01_Ratio.

Finally, decision trees were used to identify the types of profiles which manifest a higher probability of experiencing an illness in 2017. One decision tree was analysed for every variable considered (2017 cases of illness and illness ratio).
Initially, for the first tree, the sample was arbitrarily split into two units: training sample (60%) and test sample (40%). Hereafter, explanator variables were selected, type of contract, work day, contract term, longevity and number of instances of illness in 2016 and 2015. Some self-pruning parameters were defined for the tree: three levels of division and child nodes with more than 100 individuals, with the aim of guaranteeing the establishment of a non-overfixed tree. The results obtained are shown in Figure 3:
The tree produced accommodates a percentage of people for each node with no absenteeism and another complementary batch of people with absenteeism. The algorithm used to determine the predictive value for all people in a node is as follows: 0 is predicted if its associated probability is greater than a fixed amount on the decision boundary and where 1 is lower.
To correctly interpret the tree, the importance of the weight of every independent variable on the tree was taken into account, which is dependent upon the depth the variable on the tree appears with and the number of times it is present. The most important variable was the existence or non-existence of cases of illness in the previous year (100%). The second, and less relevant, variable was the cases of illness connected to the two previous years (20%). The rest of the variables manifested an influence of less than 15%.
The confusion matrix produced for a threshold value (decision threshold or boundary) equal to 50 produced an accuracy rate percentage of 81% in the training sample and 79.6% in the test sample, which indicates it is a stable tree (the variation between both samples was very low) with a very high accuracy rate.
The assessment of the performance of binary classifiers was conducted through the ROC (Receiver Operating Characteristic) curve and the PRC (Precision-Recall) curve (Figure 4). Whilst various indicators or measures exist for assessing a predictor for a binary classifier, the work of de Saito and Rehmsmeier (2015) was taken as a reference, as can be seen in Table 4.
Table 4. Description of predictor assessment measures for binary classifiers by Saito and Rehmsmeier (2015).
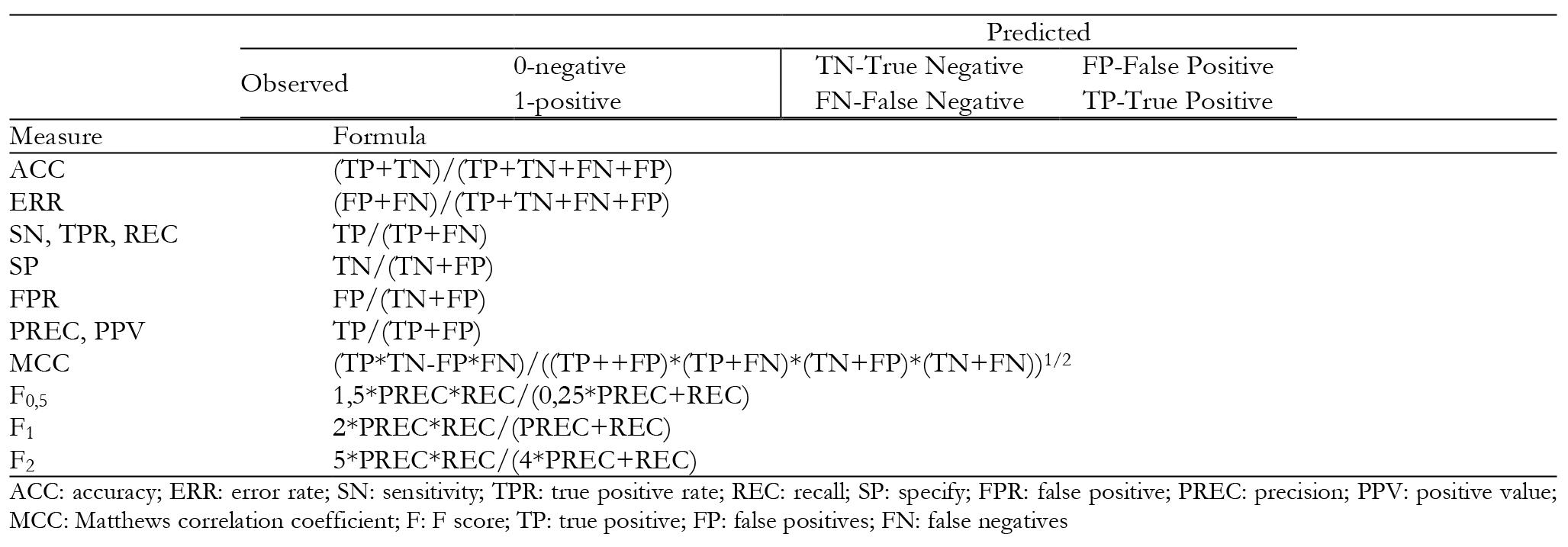
The ROC curve shows the figure obtained upon comparing the accuracy (ACC = accuracy or proportion of successes) against the sensitivity (SN = sensitivity). The curve associated with the research problem produces an area of .693.
The sample used in this study presents an unbalanced target variable (it contains just 23% of employees with an instance of illness in 2017). According to Saito and Rehmsmeier (2015), to assess a predictive value in unbalanced samples, it is more appropriate to use the PRC curve, which compares precision (PREC = precision) against sensitivity (SN = sensitivity and REC = recall).
The aim of this investigation is to identify the maximum number of employees with a case of illness, despite loss of accuracy. In other words, maximising sensitivity. Therefore, according to the PRC curve, it was decided that the threshold equal to .18 should be selected, which produces a confusion matrix with a sensitivity equal to .82 and accuracy of .34.
On the basis of the above, it was determined that nodes 5, 7, 10 and 11 have a predictive value of 1 (probability that cases of illness exist in 2017 > .18), therefore, the employees categorised into these nodes are the target of this study, as their prediction is that they experienced a case of illness in 2017.
The segmentation obtained from the group of workers is as follows:
Segment 1 (node 10): workers who experienced some sort of illness in 2016, on a permanent contract and working a full day (probability .693, number of successes = 111, number of errors = 81).
Segment 2 (node 11): workers who experienced some sort of illness in 2016, on a permanent contract and working a partial day (probability .578, number of successes = 192, number of errors = 85).
Segment 3 (node 7): workers who experienced some sort of illness in 2016, on a temporary contract (probability .486, number of successes = 72, number of errors = 76).
Segment 4 (node 5): workers who did not experience any illness in 2016, on a contract term greater than 8 months (probability .213, number of successes = 264, number of errors = 973).
On the other hand, from the second decision tree, identification of those segments of workers who represent a ratio of illness greater than 50% was attempted, taking into account the variables associated with the contract. Firstly, workers were selected who presented with a case of illness in 2017, providing a total of 1290 workers. The ratio of illness was calculated by dividing the number of sick days due to illness by the number of contracted days in 2017. Subsequently, the sample was split into two groups: a test sample (30%) and a training sample (70%). On this occasion, the target group (workers with a ratio greater than 50%) accounted for 25% of the sample, meaning that, as above, this tree is also an unbalanced sample. Explanatory variables were selected: type of contract, type of work day, contract term and longevity. The results obtained are shown in the decision tree compiled in Figure 5:
To interpret this tree, the importance indicator is made available, as before, for the weight of every independent variable in the tree, with the variable of contract term being of greatest importance (with 100%), followed by type of contract and longevity (each with 50%). Finally, the type of work day is not of importance in this tree.
The confusion matrix obtained by a threshold value equal to .50 produced an accuracy rate percentage of 74.2% in the training sample and 76.6% in the test sample. This indicates that it is a stable tree (the variation between training sample and test sample is very low), with a very high success rate.
The threshold used in this matrix is .5 and provides a sensitivity equal to .23 and accuracy equal to .52. Being an unbalanced tree, the PRC curve was used and the threshold value equal to .25 was selected, which increased sensitivity equal to .43, losing a certain level of accuracy (= .38).
Finally, the population segments predicted as ill01_ratio greater than .5 were as follows:
Segment 1 (node 6): workers on a permanent contract and a contract term greater than 8 months (probability .52, number of successes = 55, number of errors = 51).
Segment 2 (node 4): workers on a temporary contract and a contract term lower than 8 months (probability .29, number of successes = 45, number of errors = 108).
Discussion
The results obtained reveal the existence of relationships between the recruitment method and workplace absenteeism. In particular, with regard to the first hypothesis, if workers on a temporary contract are most likely to experience illness, the results obtained do not allow for this hypothesis to be tested, since it is evidenced that workers on this type of contract (temporary) show a lesser percentage of cases of common illness than workers on a permanent contract. These results contradict previous different studies, such as that of Virtanen et al. (2005). Nevertheless, they must consider the specific effects of the activity of cleaning and the ageing population, as well as the greater daily workload allocated to those on permanent contracts compared with those on temporary contracts, such as mediating variables of the results, which should be studied through future research in this field.
Furthermore, an assessment was carried out to see if the work day had an influence on absenteeism. In relation to this matter, segments 1 and 2 of the first CHAID tree highlight the fact that the partial work day provides less probability of suffering a case of common illness than the full work day, which can be explained by exposure time, which is one of the most relevant factors in physical health problems. Taking into account the fact that work place absenteeism in the cleaning sector is intimately related to a musculoskeletal aetiology, the result is completely understandable in line with the investigations of Estryn-Béhar and Van Der Heijden (2012), Gallis (2013), Moreno, Moncada, Llorens and Carrasquer (2010), and Vercruyssen and Van de Putte (2013).
Thirdly, the relationships between longevity and the possibility of experiencing illness were examined, evidencing the fact that longevity is one of the variables of greatest importance in the case of illness ratio. These conclusions must be taken with caution, given the peculiarity and specificity of the sample, and the relationship between the type of contract and the median age of the employees.
Finally, the findings made enable the last hypothesis to be verified, because in the analyses carried out, the variable ‘cases of common illness in the three reference years’ presents the same behaviour. Likewise, in the first CHAID tree, in which the variables cases of common illness in 2015 and 2016 featured, both are of great importance. In our opinion, the aforementioned history of absenteeism provides clear indicators of future absenteeism. The influence on the health of past cases may be one of the justifications, even though the inclusion of individual variables, such as personality and self-reported health, is recommended for use in future studies.
Among the limitations of this study is the lack of control over moonlighting, which is very common in a sector affected by cases of subrogation (such is the cleaning sector) which may affect the total work days of the population assessed. On the other hand, the non-probabilistic type of sample used (for convenience) makes the results neither generalisable nor conclusive. Likewise, the age of the workers, as previous different studies indicate (e.g. Fernández-García, 2013), also appears to influence cases of absenteeism, as is consistent with the ageing of the working population which Spain and Europe are experiencing. It is therefore recommended that this variable be included in subsequent studies.
Finally, although a great number of statistical methods exist for carrying out the task of classification (e.g. methods of logistic regression, discriminant analysis, neural networks, rule induction, Bayesian networks, etc.), decision trees offer clear advantages which allow all possible solutions to be fully proposed. Furthermore, their graphical representation facilitates interpretation (Chien, Wang, & Cheng, 2007). Likewise, Fleiss et al. (1972) put forward the benefits of this technique against others in the generation of diagnoses. This decision and classification technique has been widely used in the area of cognitive psychology, psychometry and the psychology of creativity (e.g., Batchelder, & Riefer, 1999; Chun et al., 2013). Nevertheless, at the same time, decision trees present different limitations, such as the large quantity of data required (available in this study) or the fact that, if costs and profits are attributed to each of the actions, it is possible to apply the maximised expected value as a decision criterion, which requires the decision maker to support the risk of downfall. In this investigation, the decision criteria has been that of identifying the maximum number of employees with a case of illness (with moderate error), without assessing the financial benefits which may be brought, a matter which should be the subject of a study in future investigations in this field.