My SciELO
Services on Demand
Journal
Article
Indicators
-
Cited by SciELO
-
Access statistics
Related links
-
Cited by Google
-
Similars in SciELO
-
Similars in Google
Share
Enfermería Global
On-line version ISSN 1695-6141
Enferm. glob. vol.22 n.69 Murcia Jan. 2023 Epub Mar 20, 2023
https://dx.doi.org/10.6018/eglobal.530591
Originals
Predictors of the post-stroke status in the discharge from the hospital. Importance in nursing
1Doctor in Nursing. Associate Professor. Faculty of Nursing and Physiotherapy. University of Salamanca. Salamanca. Spain
University Hospital of Salamanca (Emergency Service). Salamanca. Spain
2Doctor in Medicine. Full Professor. Faculty of Nursing and Physiotherapy. University of Salamanca. Salamanca. Spain
112 Emergency Service. Salamanca. Spain
3Graduate in Medicine. Associate Professor. Faculty of Medicine. University of Salamanca. Salamanca. Spain
Stroke Unit Coordinator. University Hospital of Salamanca. Salamanca. Spain
4Doctor in Medicine. Associate Professor. Faculty of Medicine. University of Salamanca. Spain
5Doctor in Sciences. Full Professor. Faculty of Science (Computing). University of Salamanca
Nurses are often asked to predict factors that influence post-stroke outcome by the patient and family. Many studies have been carried out in order to determine the factors that influence the neurological status of the post-stroke patient at the moment of the discharge from the hospital. However, machine learning techniques have not been used for this purpose. Therefore, with the objective of obtaining association rules of neurological prognosis, a double analysis, both clinical and with machine learning techniques of the possible associations of factors that influence the neurological status of the post-stroke patients has been carried out. The Apriori algorithm detected several association rules with high confidence (≥ 95%), from which the following pattern: In patients in the age range of 50-80 years, the association of a NIHSS between 11 and 15 points (intermediate/low NIHSS), along with thrombectomy, leads to recovery ad integrum at discharge. With the SMOTE resampling technique, the 100% confidence was reached for the association of high NIHSS (>20) and involvement of the carotid and basilar arteries, with a dire prognosis (exitus). These rules confirm, for the first time with machine learning, the importance of the association of some predictors, in the post-stroke prognosis. The knowledge by the nurses of these association rules can successfully improve stroke outcome. In addition, the role of nurses in education programs that teach knowledge of risk factors and stroke prognosis becomes essential.
Keywords: Stroke; clinical atlas; machine learning; nursing
INTRODUCTION
Stroke is the leading cause of acquired brain damage and disability, with an increasing incidence in developed countries1. Emergency care for a patient with a suspected stroke centers around the “time is brain”, principle due to the importance of quickly identifying patients with an ischemic stroke to prevent potentially irreversible damage to brain tissue. This scientific statement recognizes not only the importance of the nurse’s role in recognition of typical and atypical symptoms of stroke and prompt activation of the stroke response system, but also in all the stages of the evolution of the stroke, including the prognosis2,3.
Ischemic stroke caused by arterial occlusion is responsible for most strokes (more than 70%). Given that it is a time-dependent situation, correct management should include rapid and accurate triage and diagnosis1,4, and preventing not-so-frequent presentations from escaping it. Thus, it will be possible to immediately activate the stroke code, and achieve effective reperfusion of the ischemic brain area with definitive treatment such as intravenous fibrinolysis and/or endovascular mechanical thrombectomy. While the particular type of stroke may be suspected before arriving at the hospital, or on the triage set by the nurse, it cannot be confirmed without diagnostic imaging. Rapid processes are required in the hospital, and a team approach, to reduce the time from hospital admission to definitive treatment (diagnosis-needle time)5,6.
In some countries, the existence of specific stroke nurse has been pivotal in the early management of stroke patients, in the prognosis, and in the post-stroke neurological status at the moment of the discharge from the hospital. The “D’s of Stroke Care” are identified as significant steps in the management of stroke where nurses facilitate time-sensitive care are: Detection and education programs (nurses are key to community education programs that teach risk factor awareness and recognition of early stroke warning signs). Dispatch (nurses in the triage set are often the first point of contact for someone having a stroke). Delivery (comprehensive assessment of a suspected stroke patient). Door (nurses are often the link between the prehospital and the emergency department teams). Data (in order to determine processes and options). Decision (the goal is to begin stroke treatment within 30 minutes after arrival at the hospital). Drug/Device (treatment with clot dissolving medications, as well as appropriate patient monitoring during administering of the medications). Disposition (nursing interventions during transitions when a patient leaves the emergency department can successfully improve stroke outcomes)7,8.
A wide variety of factors influence stroke prognosis, including age, severity, mechanism, infarct location, previous illness and treatments, clinical findings, and related complications. In addition, interventions such as thrombolysis, mechanical thrombectomy, and stroke unit care, can play a major role in the outcome of ischemic stroke. 90% of acute ischemic strokes can be attributed to modifiable risk factors, and approximately 75% are due to hypertension, lipid metabolism alterations, diabetes, arrhythmias, and behavioral factors such as smoking, poor diet, and poor physical activity. Achieving control of risk factors could prevent more than three-quarters of the global stroke burden9. It is well known that several clinical indicators, such us the National Institute of Health Stroke Scale (NIHSS) strongly predicts outcome after stroke10. Thus, the knowledge of the important factors that affect prognosis is necessary to make a reasonable prediction for individual patients, to provide a rational approach to patient management, and to help the patient and family understand the course of the disease.
Currently, the large amount of information stored in databases about multiple patients who have suffered this pathology can be processed with machine learning techniques in order to automatically detect the most relevant factors and predict the evolution of future patients. Machine learning is a process used to obtain knowledge from large sets of raw data. It involves the application of algorithms to extract patterns from data. These fall into two main categories, supervised and unsupervised learning algorithms. Techniques in the former category are used to induce predictive models and those in the latter to discover similarities or correlations that are hidden in huge volumes of data. There are many applications domains where large databases can be exploited with machine learning algorithms. One of them is the health area since it is a rich data source, including electronic medical records, administrative reports, and medical imaging among others11,12. There are numerous studies in the literature, including from our group13)(14)(15)(16, in which different machine learning algorithms have been used for various purposes such as the automation of medical diagnosis, and prediction of mortality or treatment outcomes.
Discharge to home from rehabilitation marks an important milestone in the life of a stroke survivor. A lot of stroke survivors are being discharged home to cope with stroke-related disabilities. Although numerous studies have looked at predictors of discharge to home, and the factors that influence the functional deficits of stroke patients, in particular the multiple factors that influence the neurological status of the post-stroke patient, no machine learning techniques have been used in this area for this purpose. There is some related work but with a different objective, such as the use of these algorithms to classify the time elapsed since stroke onset based on image features17.
In a previous paper in this journal18 we have highlighted the role of nursing in triage and prediction of the stroke, based upon the scales performed by nurse in the triage area of the hospitals. In the present study we strongly amplify these previous results, with the objective of obtaining a more accurately information about the early prediction of neurological prognosis, in particular the functional independence immediately after discharge from the hospital. Thus, a combined clinical analysis and machine learning techniques of the possible associations in rules of factors that influence the neurological status of the post-stroke patients at the moment of the discharge from the hospital has been carried out.
MATERIAL AND METHODS
A total of 2080 medical records of patients with confirmed acute stroke were analyzed. The area of influence of this study was the Salamanca University Hospital and the 112 emergency service of Salamanca during the years from 2016 to 2019. This area of influence includes not only the entire province of Salamanca but also the neighboring provinces of Ávila and Zamora, which represents a registered population over 664.000 people. We must point out that Salamanca has a very high unregistered population, due to the influence that the University of Salamanca has on this data. In fact, there is an unregistered population of more than 50,000 university students, although, due to their age, the repercussion in a study of stroke, a pathology with a great relationship with age, is of minor importance, but must be considered.
We have only considered ischemic strokes in this study, ruling out hemorrhagic ones. We have used only those cases in which the stroke code was activated. Thus, the final number of medical records analyzed in the four years was 1572:
The information of the patients was obtained from the medical records under the strict control and supervision of the Documentation Service of the University Hospital of Salamanca. Data management was carried out under the rules of the Ethics Committee for the use of the information collected in the patients' medical records.
The total of parameters analyzed of the medical records have been divided into 11 groups:
Gender.
Age (divided into three groups: 0-50, 50-80, > 80).
Cardinal presentation (speech disturbances, facial asymmetry, total or partial loss of strength in the limbs, altered level of consciousness, difficulty walking and falls, balance disturbances, visual disturbances, headache).
Previous diseases (hypertension, arrhythmias - especially atrial fibrillation -, lipid metabolism alterations, diabetes, and heart diseases).
Previous medications (anticoagulants - dicoumarinics and new type generation -, digoxin, diuretics, beta-blockers, angiotensin-converting enzyme inhibitor - ACEIs -, and Angiotensin II receptor blockers - ARBs -)
NIHSS scale (divided into 5 groups: <7. 7-11, 12-15, 16-20, and >20).
MRS scale (1 to 6 points).
Diagnostic imaging (computed tomography: affected artery).
Fibrinolysis (alteplase, recombinant form of tissue plasminogen activator)
Trombectomy (primary and in addition to fibrinolysis).
Neurological status at the moment of hospital discharge. Five groups: minor neurological deficits that allow the activities of daily living to be carried out, major neurological deficits that have a negative impact on the normal development of activities of daily living, recovery ad integrum, exitus, transfer to another reference hospital.
The present work focuses on inducing patterns and rules from the previously described variables in relation to the status at the moment of the hospital discharge. All the data were analyzed in order to:
Prepare a clinical atlas of the patients under study, in which frequencies (descriptive and comparative), percentages and interrelationships between stroke and the parameters were considered.
Carry out a machine learning study of patients with acute ischemic stroke in whom the stroke code was activated in order to know which factors, individually or in combination have a greater influence on the prognosis.
Machine learning methods
Patient data has been processed using both supervised and unsupervised machine learning algorithms. The objective of supervised methods is to create a predictive model of the discharge status, from other variables that are the influencing factors reported above. Although these models can be used for making an automatic diagnosis, we have used them to analyze the patterns they enclose. In contrast, the purpose of unsupervised methods is simply to find patterns in the data that provide knowledge for making a particular decision. The aim in our study is to determine which factor or combination of factors influence the discharge status.
For supervised learning, we have used a simple method since our objective is to analyze the patterns of predictive models and the models provided by other more complex methods are very difficult to interpret. The algorithm applied was the J48 variant of the C4.5 decision tree algorithm19. Within the category of unsupervised learning, we have applied the Apriori algorithm for the induction of association rules of the type "IF X THEN Y", in which X and Y are conditions that are met related to the factors to be analyzed. For example, "IF the patient is older than 80 years, has atrial fibrillation and a high NIHSS, THEN the discharge neurological status is Major Deficits”. We found two important problems that make it difficult to obtain patterns. These are:
Data imbalance: It occurs because there are many more examples (patients) of some classes than of others, the classes being the different values of the target variable, that is, the different status at discharge.
High dimensionality: It derives from the high number of factors or variables collected for each patient, which increases the complexity of the models and the difficulty in obtaining patterns in the data.
To deal with these problems, resampling and feature selection methods were used, respectively.
As mentioned above, in this study, the Apriori predictive algorithm was applied to induce class association rules, that is, rules where conditions in the right side (Y) are only related to the class (discharge status). In addition, the J48 decision tree algorithm was used to find other types of patterns that relate the studied factors to the classes corresponding to the statuses at discharge. Initially, these techniques were applied to the data with all the factors, but no relevant patterns were obtained, so a selection of these factors was made to try to find significant relationships between their values and the classes. The selection was carried out in two different ways:
Automatically using the Correlation-based Feature Subset Selection (CFS) algorithm20.
Manually using our knowledge of the domain.
To address the problem of class imbalance, the SMOTE (Synthetic Minority Over-Sampling Technique)21 resampling strategy was applied. This is an oversampling method proposed to increase the number of instances of the minority class. Instead of replicating the instances of the minority class, new instances are generated by interpolating existing ones. An attempt has been made to detect the influence of the factors on the class, first analyzing all the factors together and then separating, on the one hand, those related to the antecedents and, on the other, those related to the treatment. Support and confidence were the metrics used to evaluate the quality of the rules. A minimum support threshold of 0.1 was established, and the patterns were considered accepTable if the confidence was equal to or greater than 0.80 over 1.
RESULTS
Clinical descriptive data (clinical atlas)
A minor predominance of male patients (871, 55,40%), when compared to females (701, 44.60%) was found in our study. It should be noted that as age increases, the percentage of women with respect to men increases. Thus, in those over 80 years of age, the number of women is greater than that of men (60.50% versus 39.50%). On considering age, was a subdivision into 3 age ranges, was performed. 70 patients (4.46%) were placed in the group under 50 years of age. The group between 50 and 80 years old presented a total of 908 (57.76%), with 594 patients in the range of older than 80 years (37.78%).
Regarding the relationship between variables, gender and age have been selected (Image 1), and their relationship with the state at discharge, as the latter is the most important variable in this study (Images 2and 3).
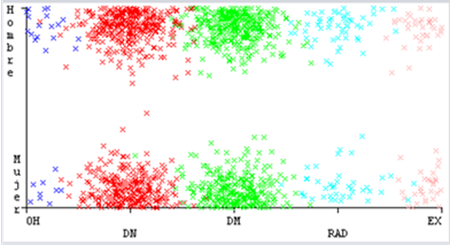
Image 2: Relationship between gender variables and status at discharge (OH: another hospital, DM: major deficits, DN: minor deficits, RAD: recovery ad integrum, EX: death).
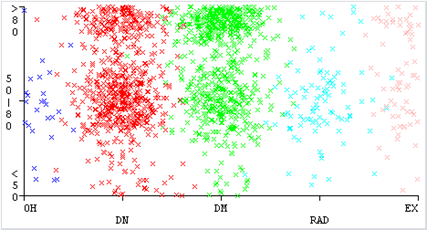
Image 3: Relationship between variables age and status at discharge (OH: another hospital, DM: major deficits, DN: minor deficits, RAD: recovery ad integrum, EX: death).
Analyzing previous diseases (see Image 4), hypertension has been the parameter with the greatest presence prior to the incident, with a total of 583 patients which represents 37.08%. 391 patients had permanent, paroxysmal, or de novo atrial fibrillation at the time of ischemic stroke diagnosis. An additional 128 patients presented some other type of arrhythmia, highlighting atrial flutter and sinus arrhythmia. Thus, the total number of patients with some type of previous arrhythmia was 519, which represents 33.01% of the total. A total of 452 patients presented some type of lipid metabolism alterations, reaching 28.75%.
Diabetes was present in 443 patients. Comparing to groups, type 2 had a higher expression (286, 18.2%) than type 1 (157, 9.91%). Of the total of 1,572 patients analyzed, 223 had non-ischemic heart disease, and 121 had previous ischemic heart disease. Thus, 344 patients had some type of heart disease at the time of diagnosis of ischemic stroke, which represents 21.88% of the total number of patients. Lipid metabolism alterations accounted for a total of 452 patients, which represents 28.75% of the records. All alterations in lipid metabolism have been considered as such, regardless of whether the altered parameters were one or several (cholesterol, high density lipoprotein or low density lipoprotein…).
A very interesting aspect has been the associations of previous pathologies observed in many of the patients who suffered stroke. They have occurred significantly among the five most frequent pathologies. Considered in pairs, the association of hypertension with lipid metabolism alterations has been present in a total of 326 patients, which represents 20.74%. The association of hypertension with arrhythmia is also very frequent, particularly with atrial fibrillation (282. 17.93%) and, to a lesser extent, hypertension with diabetes mellitus (236. 13.47%). An important group of patients presented triplet association, the most frequent being formed by hypertension, alterations of lipid metabolism and arrhythmia, singularly atrial fibrillation (201. 12.79%). Another triplet, particularly frequent, was formed by hypertension, heart disease and diabetes mellitus (172.10.94%).
By analyzing previous treatments (Image 5) 525 patients (33.39%) had prescribed diuretics prior to the stroke, both loop, thiazide, and potassium-sparing. The most frequent of all, far ahead of the rest, was furosemide. A total of 223 patients (14.16%) were receiving treatment with digoxin.
338 patients (21.51%) received treatment with ACEIs. 223 patients (14.18%) were in antihypertensive treatment with ARBs. 208 patients (13.23%) were treated with beta-blockers, the most frequent of all being bisoprolol.
259 patients (16.47%) were receiving treatment with acenocoumarol at the time of the stroke. 207 (13.16%) were taking new-type anticoagulants (rivaroxaban, dabigatran, apixaban, and edoxaban).
377 patients (23.98%) were treated with different statins. The most used were atorvastatin and simvastatin. 208 patients (13.23%) were taking betablockers (most of them bisoprolol).
The two most affected arterial territories in this study were, far above the rest, the middle cerebral arteries (image 6) with a total of 751 patients, which represents 47.77% of all stroke codes. Considered separately, 379 (24.11%) correspond to the right middle cerebral artery and 372 (23.66%) to the left middle cerebral artery. A total of 166 patients (10.56%) presented occlusion of the basilar artery, 88 (5.60%) of the right vertebral artery, 69 (4.39%) of the trunk of the right carotid artery, and 67 (4.26%) of the right posterior cerebral artery.
The rest of the cerebral arteries presented lower and quite similar occlusion Figures. The left anterior cerebral artery accounted for a total of 59 cases (3.75%), as well as tandem lesions. Similar Figures for the left posterior cerebral artery (55, 3.50%), the right anterior cerebral artery (52, 3.31%) and the left carotid trunk (50, 3.18%). The three arteries with the lowest frequency of occlusion were the left vertebral artery with 46 cases (2.93%), the left internal carotid artery with 44 (2.80%), and the right internal carotid artery with 32 (2.04%).
A total of 287 patients (18.26%) received fibrinolysis (alteplase). 330 (20.99%) were proposed for thrombectomy, as they met the requirements for it. However, finally just 255 received the mechanical endovascular treatment (thrombectomy) (Image 7).
Analyzing, the cardinal presentations, the clinical data with the greatest expression was language disorders with a total of 285 patients (18.13%), followed by the group of decreased level of consciousness, with 249 cases (15.84%). Motor problems of the extremities, partial (231, 14.69%) and total (189, 12.02%) ranked fourth and fifth, respectively. Behavioral alterations were present in 7.44% of the total cases (117 patients, Headache, was confirmed as the onset in 56 cases (3.56%). Visual disturbances showed a very low expression with a total of 31 cases (1.97%).
Considering the NIHSS scale, the most important number of patients (474, 30.15%) was situated in the range between 12-15 points. 435 (27,67%) patients were in the range of 16-20 points. 387 patients (24.62%) were situated between 7 and 11 points, while 186 (11.83) were in the area with the highest score above 20 points. With less than 7 points, a total of 36 patients (2.29%) were observed. Analyzing the MRS scale, a total of 808 (51.40%) showed 0 points, indicating that their previous baseline status allowed them to be valid for all possible activities of daily life. The next two groups with the largest number of patients were those that presented a score of 1 (379, 24.11%) or 2 points (11.13%).157 patients (9.99%) presented at the time of stroke diagnosis a total of 3 points, and 38 patients (2.417%) added 4 points. No cases of patients with 5 or 6 points were observed.
A total of 706 patients (44.91%) were discharged with major deficits (Image 8), which seriously hindered their activities of daily living, requiring a permanent caregiver. 674 patients (42.87%) presented minor deficits at the time of discharge. They were valid for most of the activities of daily life, but in some of them, they presented the need for specific help. Of the total of 1572 patients considered in this study, 96 (6.11%) were discharged with recovery to normality, ad integrum, or minimal symptoms that did not impede or hinder, without external help, activities of daily living. 27 patients (1.72%) were transferred to another hospital outside the Autonomous Community of Castilla y León, and a total of 69 patients (4.39%) died before being discharged.
Machine learning analysis
The class analyzed by machine learning was the patient's condition (neurological status) at the time of discharge. Different scenarios with different factors were analyzed in the search for patterns of relationship between all the factors or subsets of these with the neurological situation at discharge. On the other hand, there was a great imbalance in the number of instances or records (each record corresponds to a patient and its corresponding data) for the different values of the class variable. The minor deficits and major deficits values have a much higher number of records than the recovery ad integrum and exitus values.
With the initial factors as a whole, no pattern of association rules was obtained, so we then proceeded to CFS method in order to determine which are the most relevant attributes in relation to the object class of study. In addition, the SMOTE resampling strategy was applied, due to the imbalance in the number of instances of the classes. Instances of the ad integrum recuperation and exitus minority classes were resampled to 500%. Once the preprocessing was done, the Apriori algorithm was applied to obtain the association rules. The method detected four rules of association with a confidence equal to or greater than 95%, from which the following pattern common to all of them can be extracted: Patients in the age range of 50-80 years, the association of a NIHSS between 11 and 15 points (intermediate/low NIHSS), along with thrombectomy, leads to ad integrum recuperation at discharge.
We then proceeded to consider only the factors related to the previous disease. Considering all the antecedents as a whole, the Apriori algorithm did not generate any reliable rules. The CFS and SMOTE algorithms were then applied for feature selection and resampling, respectively. Once the preprocessing was done, the Apriori algorithm yielded two association rules with a confidence above 0.80. It was observed that advanced age and having already suffered at least two of the previous diseases are data that, in association, lead to major neurological deficits at discharge. The J48 decision tree algorithm has also been applied to try to find other different rules than those provided by the Apriori algorithm. The tree model consisted of complex patterns in which many factors are involved. In addition, from this model it was possible to extract information about the most influential factors on the prediction of the class, which are the variables located at the highest levels of the tree. Thus, we observe age, arterial hypertension, and atrial fibrillation, as the most important, together with diabetes mellitus.
We then proceeded to consider only variables from the previous treatment. Considering all these variables, no reliable rule was detected, so the CFS and SMOTE algorithms were used again. In this case, only association rules with confidence below 0.80 were found. In order to obtain decision rules and influential factors, the J48 Algorithm was applied again. Among the most influential variables are previous treatments with acenocoumarol, antiarrhythmics, and antihypertensive treatments.
Based on domain knowledge, we make several targeted attribute selections, relative to the class:
First, patterns with possible significant confidence were sought for attributes, age, NIHSS, and thrombectomy performance. In addition to selecting the attributes, the SMOTE resampling strategy was applied. In this case, 5 rules were obtained with a confidence above 0.85. We were able to observe how, in the age range between 50 and 80 years, the association of not very high NIHSS, fibrinolysis and thrombectomy leads to recovery ad integrum with high confidence.
Relevant patterns were sought for the factors, NIHSS, performance of fibrinolysis and performance of thrombectomy. After feature selection and resampling, 3 rules with a confidence greater than 0.80 were found. Without considering age, these results are totally superimposable to those of the previous section. The association of fibrinolysis, thrombectomy and NIHSS that are not very high are determining factors in achieving good functional status at discharge (minor neurological deficits).
Considering a third group of variables (age, NIHSS and affected artery) and resampling with SMOTE, only 4 rules exceeded the confidence of 0.80. The 100% confidence was reached for two of the rules. The association of high NIHSS, with angiography affecting both anterior and posterior large arterial trunks (carotid and basilar), is related to a very poor prognosis (exitus).
Applying the Apriori algorithm to the factors age, atrial fibrillation, hypertension, diabetes and lipid meabolisms alterations, with the resampled dataset, 20 rules were obtained with a confidence greater than 0.80. When all the selected antecedents are associated, the prognosis at discharge is, once again, very poor.
DISCUSSION
Machine learning, simply explained, is a process used to extract useful information that is hidden in large volumes of data. Association patterns obtained through machine learning can be used to make predictions or simply to find hidden relationships in the clinical information. The data collected in this study are the first processed with machine learning techniques, to the best of our knowledge, on the stroke code worldwide. Our study reveals data association patterns using this approach, some novel and others confirming previously existing patterns with other statistical and data management techniques9,10.
Clinical decision making is essential in stroke, not only at the moment of the triage by the nurse, but also in the previous detection and control of risk factors4. Today, it is well known that the main risk factors for stroke include hypertension, diabetes mellitus, lipid metabolism alterations, arrhythmias (especially atrial fibrillation), and cardiovascular diseases3,9. These five diseases are considered “the five (not four!) horsemen of stroke apocalypse”. The knowledge of them, and the importance of the most dangerous associations of them in the stroke prognosis are a clue instrument for the nurse.
In many application domains, such as medicine, the datasets used to drive classification models often have an uneven distribution of instances of each class. This causes the results being based mainly on the majority class, obtaining few patterns from the minority class and, in prediction problems, a significantly lower precision for this class. Often the minority class is the most interesting in terms of the application domain being studied, so the problem is compounded in these cases. Our own team, in previous works and publications analyzing different pathologies and medical situations, has detected these problems13)(14)(15)(16.
In order to reduce the dimensionality of the data, one of the problems of our analysis, we have used feature selection methods in our study, which has allowed us to obtain association rules with higher support and confidence values. Likewise, as shown in the results section, the application of this type of method has resulted in an increase in the reliability of the prediction models. To deal with the problem of data imbalance, resampling strategies have been used. Specifically, in our study we have applied the SMOTE technique, which is the one that has provided us with the best results, as in previous studies13)(14)(15)(16. With the resampling of the data, we have managed to obtain a greater number of association rules that exceed the support and confidence thresholds. As we have showed in the results, machine learning clearly shows that age, hypertension, atrial fibrillation, and diabetes mellitus are as the most important factors in determining the neurological status at the moment of discharge from the hospital.
Today, it is well known that hypertension is a powerful determinant of ischemic stroke risk, with clear previous data showing that controlling blood pressure levels <140/90 mmHg reduces the risk of stroke. Evidence of benefit is weaker for lower blood pressure targets achieved with aggressive reduction, especially in older patients22,23.
Diabetes mellitus is an important risk factor for stroke24. It causes pathological changes in the blood vessels and can lead to a stroke if the brain vessels are directly affected. In addition, mortality is higher and post-stroke outcomes are poorer in patients with uncontrolled glucose levels. Control of diabetes and other associated risk factors are effective ways to prevent strokes, as well as their recurrence24,25. As it is a disease mainly associated with lifestyle, patients with diabetes mellitus 2 usually have additional risk factors for stroke, such as obesity, hypertension, and dyslipidemia, which multiplies the vascular risk in these patients24. Our results show an association of hypertension, heart disease, and diabetes mellitus in a significant number of patients (172, 10.94%).
There is a complex relationship between normal serum lipid levels and stroke risk. When stroke is subtyped into ischemic and hemorrhagic, contrasting relationships are found between them. High levels of total cholesterol are associated with an increased risk of ischemic stroke, while low levels are associated with an increased risk of intracerebral hemorrhage26. Within ischemic stroke, the subtype of atherothrombotic and occlusive disease of the large arteries has been more closely related to higher levels of total cholesterol. In our study, lipied alterations was one of the pathologies with the greatest presence, reaching a total of 452 patients, confirming it as a predictive clinical history of ischemic stroke, and forming part of the five horsemen of the stroke apocalypse.
Arrhythmias in general, and atrial fibrillation in particular, significantly increase the risk of ischemic stroke. Oral anticoagulation reduces the risk of stroke27,28. It is estimated that about 40 million people have atrial fibrillation in developed countries. During the last decades, we have learned that this dysrhythmia originates from the interaction between genetic predisposition, ectopic electrical activity, and abnormal atrial tissue substrate. Atrial fibrillation and stroke have been associated in rigorous studies, indicating a true association rather than a false finding27,28.
Five rules were obtained with a confidence above 0.85, highlighting the association of an NIHSS in the interval 11-16, and the performance of thrombectomy and fibrinolysis, with a confidence of 0.93, for a high ad integrum recovery. These results would be comparable to previous series with descriptive studies and traditional statistics, in which the NIHSS are not very high, and the performance of revascularization techniques, particularly when they are successful, suppose an evident improvement in the functional status of the patient at discharge. and in the following months. There is therefore no doubt, and it is an irrefuTable fact in 2022, that the main objective of advanced treatment of acute ischemic stroke, and the end of the activation of the stroke code, is the early revascularization of the cerebral ischemic area and the limitation of neuronal damage. Mechanical thrombectomy doubles the odds of a better functional outcome compared to standard therapy alone, with no significant difference in mortality or risk of parenchymal hemorrhage at 90 days. Mechanical endovascular treatment should not be avoided on the basis of age alone, and patients older than 80 years may also benefit from it. Although the neurobiological rationale underlying these subgroups remains to be explored, factors that may contribute to different recovery rates include the amount of perilesional edema, as well as remote effects on distant but structurally and functionally connected brain regions, due to injury3,29.
In our study, a total of 706 patients were discharged with major deficits (44.91%), 674 with minor deficits (42.87%), 96 with ad integrum recovery (6.11%) and 69 (4.39 %) were exitus at some point in the entire process since the stroke code was activated. 27 patients (1.72%), for different reasons, normally return to their reference hospital, were discharged before fulfilling the requirements for it. As we have already commented in the results section, the group with the greatest deficits normally presented the highest NIHSS at the time of diagnosis, together with the fact that it was not possible to apply fibrinolysis or thrombectomy, either one of them, or both techniques. Additionally, and logically from the clinical point of view, discharges with recovery ad integrum used to correspond to low NIHSS together with the application of at least one of the two effective treatments, either fibrinolysis, thrombectomy, or both.
With the SMOTE resampling technique, the 100% confidence reached for the association of high NIHSS (>20.4) and involvement of the carotid and basilar arteries, with a dire prognosis (exitus), was striking. These results could be extrapolated to previous studies with other assessment methods in which high NIHSS are always associated with a worse prognosis1)(9)(10. Along with this, it is known that the medial cerebral are statistically the most affected arteries and with the worst NIHSS, with some evolutions being considered malignant strokes.
In summary, in our present study we confirm with machine learning techniques, for the first time, the importance and influence of the association of some traditional predictors, in the stroke prognosis. The analysis of the rules of association of the predictors found in our study will be of particular interest for clinicians, including nurses30, to predict the neurological status of the post-stroke patient.
CONCLUSIONS
Nurses serve in a critical role during a patients’ stroke management and treatment by providing prognosis, communication and care prior to, during and after medical procedures. As a consequence, nurses are often asked, to predict factors that influence post-stroke outcome. In the light of our results and previous publications, nursing staff can successfully improve stroke outcomes, by adequately managing the stroke predictors, obtained not only by means of pure and traditional clinical data, but also by machine learning methods.
Acknowledgements
The authors would like to thank the Admission and Documentation Service of the Salamanca University Hospital, for their contribution and facilitation of electronic access to the medical records of patients, under the rules of ethics and data protection. Special mention to Arancha García MD.
REFERENCES
1. Powers WJ, Rabinstein AA, Ackerson T, Adeoye OM, Bambakidis NC, Becker K, Biller J, Brown M, Bart M. BM, Hoh B, Jauch EC, Kidwell CS, Leslie-Mazwi TM, Ovbiagele B, Scott PA, Sheth KN, Southerland AM, Summers DV, L. Tirschwell DL, and on behalf of the American Heart Association Stroke Council. 2018 Guidelines for the early management of patients with acute ischemic stroke: A guideline for healthcare professionals from the american heart association/american stroke association. Stroke. 2018. 49: 46-99. Doi: 10.1161/STR.0000000000000158. [ Links ]
2. Green TL, McNair ND, Hinkle JL, Middleton S, Miller ET, Perrin S, Power M, Southerland AM, Summers DV; American Heart Association Stroke Nursing Committee of the Council on Cardiovascular and Stroke Nursing and the Stroke Council. Care of the Patient with Acute Ischemic Stroke (Posthyperacute and Prehospital Discharge): Update to 2009 Comprehensive Nursing Care Scientific Statement: A Scientific Statement From the American Heart Association. Stroke. 2021. 52(5): e179-e197. Doi: 10.1161/STR.0000000000000357. [ Links ]
3. Xu ZH, Deng QW, Zhai Q, Zhang Q, Wang ZJ, Chen WX, Gu MM, Jiang T, Zhou JS, Zhang YD. Clinical significance of stroke nurse in patients with acute ischemic stroke receiving intravenous thrombolysis. BMC Neurol. 2021. 21: 359. Doi: 10.1186/s12883-021-02375-6. [ Links ]
4. Stanfield LM. Clinical decision making in triage: an integrative review. J Emerg Nurs. 2015. 41(5): 396-403. Doi: 10.1016/j.jen.2015.02.003. [ Links ]
5. Xian Y, Xu H, Lytle B, Blevins J, Peterson ED, Hernandez AF, Smith EE, Saver JL, Messe SR, Paulsen M, Suter RE, Reeves MJ, Jauch EC, Schwamm LH, Fonarow GC. Use of strategies to improve door- to-needle times with tissue-type plasminogen activator in acute ischemic stroke in clinical practice: findings from Target: Stroke. Circ Cardiovasc Qual Outcomes. 2017. 10: 003227. Doi: 10.1161/CIRCOUTCOMES.116.003227. [ Links ]
6. Ragoschke A, Walter S. DAWN and DEFUSE-3 trials: is time still important? Radiologe. 2018. 58(1): 20-23. Doi: 10.1007/s00117-018-0406-4. [ Links ]
7. American Heart Association Scientific Statements. Updated guidance confirms crucial role of nurses for patients with acute ischemic stroke. Scientific Statements/Guidelines. 2021. https://newsroom.heart.org/news/updated-guidance-confirms-crucial-role-of-nurses-for-patients-with-acute-ischemic-stroke. [ Links ]
8. Loft MI, Poulsen I, Martinsen B, Mathiesen LL, Iversen HK, Esbensen BA. Strengthening nursing role and functions in stroke rehabilitation 24/7: A mixed-methods study assessing the feasibility and acceptability of an educational intervention programme. Nurs Open. 2018. 19; 6(1): 162-174. Doi: 10.1002/nop2. 202. [ Links ]
9. Libruder C, Ram A, Hershkovitz Y, Karolinsky D, Tanne D, Bornstein NM, Zucker I. The contribution of potentially modifiable risk factors to acute ischemic stroke burden - Comparing young and older adults -. Prev Med. 2022. 155: 106933. Doi: 10.1016/jypmed.2021.106933. [ Links ]
10. Murie-Fernández M, Marzo MM. Predictors of Neurological and Functional Recovery in Patients with Moderate to Severe Ischemic Stroke: The EPICA Study. Stroke Res Treat. 2020. 1419720. Doi:10.1155/2020/1419720. [ Links ]
11. Dash S, Shakyawar SK, Sharma M, Kaushik S. Big data in healthcare: management, analysis and future prospects. J Big Data. 2019. 6: 54. Doi: 10.1186/s40537-019-0217-0. [ Links ]
12. Parisi L, Chandran NR, Manaog ML. Feature-driven machine learning to improve early diagnosis of Parkinson's disease. Expert Syst. Appl. 2018. 110: 182-190. Doi: 10.1016/j.eswa.2018.06.003 [ Links ]
13. González J, Martín, F, Sánchez M, Sánchez F, Moreno MN. "Multiclassifier systems for predicting neurological outcome of patients with severe trauma and polytrauma in intensive care units". J Med Syst. 2017. 41: 136. Doi: 10.1007/s10916-017-0789-1. [ Links ]
14. Martín-González F, González-Robledo J, Sánchez-Hernández F, Moreno-García MN. Success/Failure Prediction of Noninvasive Mechanical Ventilation in Intensive Care Units. Using Multiclassifiers and Feature Selection Methods. Methods Inf Med. 2016. 55(3): 234-41. Doi: 10.3414/ME14-01-0015. [ Links ]
15. Kraiem MS, Sánchez-Hernández F, Moreno-García M. Selecting the suiTable resampling strategy for imbalanced data classification regarding dataset properties. 2021. Models. Appl. Sci. 11, 8546. Doi: 10.3390/app11188546. [ Links ]
16. Sánchez-Hernández F, Ballesteros-Herráez JC, Kraiem MS, Sánchez-Barba M, Moreno García MN. "Predictive Modeling of ICU Healthcare-Associated Infections from Imbalanced Data. Using Ensembles and a Clustering-Based Undersampling Approach". Appl. Sci. 2019. 9(24): 5287. Doi: 10.3390/app9245287. [ Links ]
17. Zhang YQ, Liu AF, Man FY, Zhang YY, Li C, Liu YE, Zhou J, Zhang AP, Zhang YD, Lv J, Jiang WJ. MRI radiomic features-based machine learning approach to classify ischemic stroke onset time. J Neurol. 2022. 269, 350-360. Doi: 10.1007/s00415-021-10638-y. [ Links ]
18. Rodríguez V, Sánchez F. Nursing triage in acute stroke. Enfermería Global. 2021. 64:120-131. Doi: 106018/eglobal.465261. [ Links ]
19. Quinlan JR. C4.5: Programs for machine learning. Morgan Kaufmann. 1993. San Mateo, CA. USA. [ Links ]
20. Hall MA. Correlation-based feature selection for machine learning. Ph.D diss. Dept. of Computer Science. Waikato University. 1998. [ Links ]
21. Chawla NV, Bowyer K, Hall LO, KebelmeyerWP. SMOTE: synthetic minority over sampling technique. J. Artif Intell Res. 2002. 202(16): 321-357. Doi: 10.1613/jair.953. [ Links ]
22. Zonneveld TP, Richard E, Vergouwen MD, Nederkoorn PJ, de Haan R, Roos YB, Kruyt ND. Blood pressure-lowering treatment for preventing recurrent stroke, major vascular events, and dementia in patients with a history of stroke or transient ischaemic attack. Cochrane Database Syst Rev. 2018. 7: CD007858. Doi: 10.1002/14651858.CD007858.pub2. [ Links ]
23. Weiss J, Freeman M, Low A, Fu R, Kerfoot A, Paynter R, Motu'apuaka M, Kondo K, Kansagara D. Benefits and harms of intensive blood pressure treatment in adults aged 60 years or older: a systematic review and meta-analysis. Ann Intern Med. 2017. 166: 419-29. Doi: 10.7326/M16-1754. [ Links ]
24. Tun NN, Arunagirinathan G, Munshi SK, Pappachan JM. Diabetes mellitus and stroke: A clinical update. World J Diabetes. 2017. 8(6): 235-248. Doi: 10.4239/wjd.v8.i6.235. [ Links ]
25. Saeedi P, Salpea P, Karuranga S, Unwin N, Wild SH, Williams R. Mortality attribuTable to diabetes in 20-79 years old adults, 2019 estimates: Results from the International Diabetes Federation Diabetes Atlas, 9th edition. Diab Res Clin Prac. 2020. 162: 108086. Doi: 10.1016/j.diabres.2020.108086. [ Links ]
26. Hackam DG, Hegele RA. Cholesterol Lowering and Prevention of Stroke. An Overview. Stroke. 2019. 50: 537-541. 2019. Doi: 10.1161/STROKEAHA.118.023167 [ Links ]
27. Li YG, Lip GYH. Stroke prevention in atrial fibrillation: State of the art. Int J Cardiol. 2019. 287: 201-209. Doi: 10.1016/j.ijcard.2018.09.057. [ Links ]
28. Katsanos AH, Kamel H, Healey JS, Hart RG. Stroke Prevention in Atrial Fibrillation. Looking Forward. Circulation. 2020. 142: 238. Doi: 10.1161/CIRCULATIONAHA.120.049768. [ Links ]
29. Albers GW, Marks MP, Kemp S, Christensen S, Tsai JP, Ortega-Gutierrez S, McTaggart RA, Torbey MT, Kim-Tenser M, Leslie-Mazwi T, Sarraj A, Kasner SE, Ansari SA, Yeatts SD, Hamilton S, Mlynash M, Heit JJ, Zaharchuk G, Kim S, Carrozzella J, Palesch YY, Demchuk AM, Bammer R, Lavori PW, Broderick JP, Lansberg MG; DEFUSE 3 Investigators. Thrombectomy for stroke at 6 to 16 hours with selection by perfusion imaging. 2018. N Engl J Med. 378(8): 708-718. Doi: 10.1056/NEJMoa1713973. [ Links ]
30. Gong L, Ruan C, Yang X, Lin W. Effects of Predictive Nursing Intervention among Patients with Acute Stroke. Ir J Public Health. 2021. 50(7): 1398-1404. Doi:10.18502/ijph.v50i7.6629. [ Links ]
Received: July 04, 2022; Accepted: October 09, 2022