Alcohol and other substance use disorders are frequently associated with harmful outcomes, including increased risk of injury and suicide (Edwards et al., 2020; Rehm et al., 2017; Roerecke & Rehm, 2014; Weil et al., 2018), communicable diseases (Rehm, 2011; Rehm et al., 2017), social disadvantage (Doran et al., 2018; Duke & Searby, 2019; Fazel et al., 2008), and mortality (Holst et al., 2017; Rehm et al., 2013). Moreover, substance use disorders are associated with the loss of up to 5.1% of daily adjusted life years (DALY) globally (World Health Organization [WHO], 2018), as well as significant costs including health care, productivity loss, and criminal justice systems (Manning & Mazerolle, 2013; Peacock et al., 2018). Subsequently, there have been substantial efforts to understand and address the mechanisms underpinning higher-risk alcohol use.
From a cognitive perspective, alcohol and other substance use disorders are underpinned by alterations in inhibitory control (disinhibition), the ability to inhibit rapid and context-inappropriate responses (Bari & Robbins, 2013; Nigg, 2017). Inhibitory control has been conceptualised as a multifaceted construct, consisting of at least three different mechanisms: attentional control, information gathering, shifting and monitoring of feedback (Sharma et al., 2014; Verdejo-Garcia et al., 2021). Deficits in these mechanisms have been observed in individuals with higher-risk alcohol use, with increased levels of severity and associated harm generally relating to greater impairment. For example, those with alcohol use disorder display significantly poorer attentional control relative to healthy controls (Kopera et al., 2012), with higher levels of alcohol use negatively associated with attentional control impairment (Ames et al., 2014; Claus et al., 2013). Similarly, greater levels of alcohol use severity are associated with decreased efficiency of shifting and monitoring of feedback capabilities (Houston et al., 2014; Loeber et al., 2009). Similar results have been observed in people with stimulant and opioid use disorders (Fernandez-Serrano et al., 2010). While individuals with alcohol use disorder tend to gather information more slowly than healthy counterparts (Mandali et al., 2019), it is not yet clear whether this deficit is modulated by the severity of alcohol use. However, emerging research suggests that poorer information gathering in early adulthood may be a salient predictor of future alcohol and substance use severity (Weigard et al., 2021). Overall, alcohol use problems are associated with a profile of cognitive disinhibition that is distinct from healthy individuals and appears to worsen with higher-risk alcohol use.
Previous research attempts to profile disinhibition by levels of alcohol and drug use are associated with potential limitations. Samples recruited from treatment services often present with more severe clinical presentations and are not representative of the broader treatment-seeking population with alcohol use related problems (Subbaraman et al., 2015). Further, research is often constrained by small samples sizes, as well as the use of strict inclusion criteria to control for comorbidities (Moberg & Humphreys, 2017; Strickland & Stoops, 2019). This can negatively impact statistical power and generalisability of research findings (Button et al., 2013). In contrast, recruitment through online support services can provide an invaluable method of yielding larger samples and reaching participants under-represented in mainstream treatment services (Chebli et al., 2016; Miller & Sønderlund, 2010), while providing cognitive task data that is comparable to in-person assessment in terms of reliability and validity (Chandler & Shapiro, 2016; Mortensen & Hughes, 2018; Stewart et al., 2017). Importantly, online support services may also minimise practical issues related to recruitment, including geography, financial costs, and concerns around privacy, which are key barriers to traditional face to face addiction research (Ekström & Johansson, 2019; Gainsbury & Blaszczynski, 2011; Kiluk et al., 2018).
Further limitations to the measurement of disinhibition among people who use alcohol and other substances include the lack of comprehensive and reliable measures of distinct cognitive processes underpinning disinhibition (Eisenberg et al., 2019; Enkavi et al., 2019), as well as poor criterion validity between self-report and cognitive measures (Dang et al., 2020; Enkavi & Poldrack, 2021). To address these issues, we recently developed a novel online gamified measure of disinhibition and its cognitive components (the Cognitive Impulsivity Suite or CIS; Verdejo-Garcia et al., 2021). The CIS measures disinhibition across three empirically validated components (attentional control, information gathering, and feedback monitoring/shifting), and has the advantage of assessing these separate cognitive processes via individual tasks with similar motor and perceptual demands. Moreover, the online mode of delivery makes the CIS suitable for testing participants who are less likely to use traditional treatment services, such as populations facing increased stigma and scarcity of services catering to their needs (e.g., women), as well as those residing in remote locations.
This study aimed to examine the association between the severity of alcohol and drug use and different mechanisms of cognitive disinhibition measured with the CIS among people who engage with online addiction treatment support. We hypothesized that higher-risk alcohol and drug use groups, identified through latent class analysis of the Alcohol Use Disorders Identification Test (AUDIT) and the Drug Use Disorders Identification Test (DUDIT) responses, would be associated with poorer performance in the CIS across tasks measuring attentional control, information gathering, and feedback monitoring/shifting.
Method
Participants
Participants were recruited from Counselling Online (https://www.counsellingonline.org.au/ ), an Australian national online alcohol and other drug support service, between 12 August 2018 and 10 October 2019. The study was situated under a ‘self-assessment' link on the website and participants could choose to assess their impulsivity by playing the CIS games and receiving feedback on their performance, as well as general recommendations to help regulate impulsive behaviors (e.g., encouraging goal-focussed decisions and relaxation strategies). Eligibility to participate in the study was limited to individuals aged 18 years and over, with the absence of vision impairments (including color-blindness), a history of head trauma, or neurological illness. Completion and genuine response criteria, based on previously proposed recommendations for online cognitive testing (Kaplan et al., 2017; Strickland & Stoops, 2019) were included to minimize a pattern of false responding and detect participants who deliberately skipped sections of the study. The survey was hosted on Qualtrics. Participants received $10AUD for completing the study.
Instruments
The Cognitive Impulsivity Suite (CIS)
The CIS is a set of three gamified tasks assessing key cognitive drivers of disinhibition, including difficulties with attentional control, lack of information gathering, and poor feedback monitoring/shifting (Verdejo-Garcia et al., 2021). The three CIS mechanisms were conceptualized from meta-analytic factor-findings and biological brain lesion-based findings that have identified separate brain areas and processes involved in disinhibition (Sharma et al., 2014; Stuss & Alexander, 2007). Each task has a common theme and layout and is similar in visual design, 4-block-structure (40 to 60 trials each), and binary choice response requirements (see Figure 1). The CIS takes approximately 35 minutes to complete and the task order is randomized.
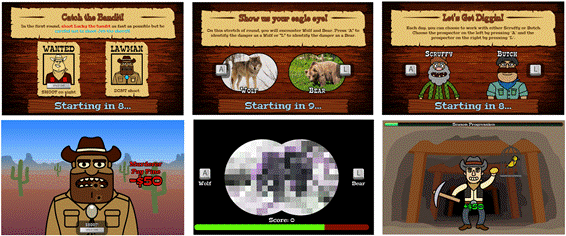
Note. The images from left to right reflect Bounty Hunter, Caravan Spotter, and Prospector's Gamble tasks.
Figure 1. CIS Task Images
Bounty Hunter. This task captures attentional control. Participants are presented with one of two stimuli in each trial, a bandit or a sheriff character. They are tasked to shoot the bandits (“go”), but not the sheriffs (“no-go”) by making quick and accurate “go” (press spacebar) or “no-go” (withhold from pressing spacebar) responses. Stimulus onset asynchronies (SOAs; the interval between the cue and the stimulus) are manipulated with shorter (50ms, 200ms) or longer (1500ms, 3000ms) SOAs, to challenge recruitment and maintenance of attentional resources, respectively. The data from the Bounty Hunter task were analyzed using the principles of Signal Detection Theory (Stanislaw & Todorov, 1999), which are superior to analyses on overall accuracies as they separate the distinct components of response bias (Criterion, C) and discriminability (D Prime; d'; Macmillan & Creelman, 2005). Higher Criterion C scores indicate more conservative responding with a lower proportion of false alarms and correct (“hit”) responses. Higher D Prime scores reflect a greater ability to discriminate between correct and incorrect stimuli.
Caravan Spotter. This task captures information gathering. Participants are presented with an ambiguous pixelated image that gradually disambiguates during the trial. Images initially appear 50% pixelated, linearly transitioning to 100% unpixellated across a 2000ms response window. Participants must decide whether the image reflects category A or category B (e.g., a horse or a wagon). The primary outcomes were mean parameters from drift diffusion modelling (DDM); a framework describing information accumulation that is suited to speeded binary decisions (Ratcliff & Tuerlinckx, 2002). The starting point bias (z), describing whether an evidence accumulation advantage to the correct response, was modelled as constant (0) across participants. DDM permits the calculation of different components of the decision process, including the speed at which information accumulation approaches one of the decision boundaries (i.e., the drift rate; v) and the distance between the two boundaries (upper threshold; a). Higher drift rate reflects a tendency to absorb more information per time unit before making a decision (i.e., reflecting perceptual sensitivity) and a higher upper threshold reflects a tendency to accumulate more evidence before triggering the selected choice (i.e., conservative rather than liberal response style; Voss et al., 2004).
Prospectors Gamble. This task captures the ability to monitor feedback and shift behavior accordingly. Participants are presented with two characters (gold prospectors); the goal of the task is to select the prospector who can earn the greater amount of gold. Participants are informed that one of the prospectors will always be luckier than the other one, but also that their luck may change. A time pressure element was included, requiring participants to make selections in a short (1000ms) interval. The key outcome was the proportion of total perseverative errors (i.e., selecting the previously correct stimulus following stimulus change). Higher errors reflect reduced feedback monitoring and shifting.
Alcohol Use Disorders Identification Test (AUDIT)
The AUDIT (Babor et al., 2001) is a 10-item self-report measure of alcohol consumption and alcohol-related problems over the past 12 months. Items are rated on a 5-point Likert-type scale. Total scores range between 0-41 with higher scores reflecting greater alcohol use. A raw score of 8 or more indicates harmful patterns of alcohol use (Reinert & Allen, 2007). Validity and reliability for the AUDIT are well established in both community and clinical (i.e., substance use) adult samples (de Meneses-Gaya et al., 2009).
Drug Use Disorders Identification Test (DUDIT)
The DUDIT is an 11-item self-report measure of illicit drug use and related consequences over the past 12 months (Berman, et al., 2005). Each item is rated on a 5-point Likert type scale. Total scores can range from 0-48 with higher scores reflecting greater drug use. Drug-related problems are indicated by a raw score of 6 or more in men and a raw score of 2 or more in women (Berman et al., 2005). The DUDIT has demonstrated excellent reliability in men and women seeking web-based addiction assessment and services (α = .97 (Sinadinovic et al., 2010), and is a valid indicator of drug use related problems (Hildebrand, 2015).
Procedure
The study was approved by Monash University Human Research Ethics Committee (MUHREC project number 7536). Participants who clicked on the ‘self-assessment' and ‘impulsivity' tabs were informed they would be asked to play three mini-games to test three different thinking styles that can lead to impulsive actions and decisions. Participants completed a short eligibility survey, accessed a digital explanatory statement and provided informed consent. They were then redirected to complete the CIS, followed by a survey consisting of demographic questions (e.g., age, sex, education) and the AUDIT and DUDIT questionnaires. The study took approximately 50 minutes.
Data Analysis
A total of 184 participants completed the entire study. CIS data were evaluated according to pre-determined criteria for each task based on Eisenberg et al. (2019) recommendations for cognitive tests in online studies; detailed in the Additional Information file. If performance did not meet these criteria, participants' responses on that task were not included in the analysis. Only participants with valid data across two of the three CIS tasks were included. Two participants were missing feedback monitoring/shifting data and four participants were missing attentional control data.
Participants with a total score of 0 on both the AUDIT and DUDIT were removed from the analysis as they were not reflective of the target help-seeking sample. The final sample included 137 participants. Years of education values were very low for two participants (< 5 years), representing extreme outliers. As these appeared to reflect confusion over the question, (i.e., less than primary school education) the data were replaced using multiple imputation. One participant was missing one value on the AUDIT. Multiple imputation was also performed to replace this value, which was Missing Completely at Random. The D Prime outcome was negatively skewed; this outcome was reflected, and log transformed to approximately normal distribution.
Latent class analyses of the AUDIT responses were conducted to identify subgroups with distinctive patterns of alcohol use. Given the low number of participants reporting drug use (n = 66) and the limited variability in their DUDIT scores (see Additional Figure 1) the relationship between DUDIT severity and CIS performance was not analyzed. We performed separate hierarchical multiple regression analyses for each cognitive disinhibition outcome (i.e., the CIS task outcomes). As increased age in adulthood has been associated with poorer performance in cognitive inhibition tasks (Argyriou et al., 2018; MacKillop et al., 2016), we controlled for this variable in each of the analyses. See Additional Table 1 for correlations between key demographic variables and disinhibition outcomes. Variables were entered in blocks as follows: (1) age, (2) alcohol use group, and (3) interaction product of age and alcohol use group. Variables were centred prior to calculating the interaction.
Table 1. Demographic Information, AUDIT, and DUDIT Scores (N = 137)
M | SD | Range | n (%) | |
---|---|---|---|---|
Age (years) | 33.10 | 10.64 | 18.00 - 64.00 | 134 |
Education (Years) | 16.59 | 3.11 | 6.00 - 29.00 | 135 |
Sex (% women) | 80% | 109 | ||
Ethnicity | 137 | |||
Caucasian | 110 (80%) | |||
Asian | 15 (11%) | |||
Indigenous Australian | 2 (1%) | |||
Other | 10 (7%) | |||
Employment | 133 | |||
Professional | 59 (43%) | |||
Student | 17 (12%) | |||
Manager | 9 (7%) | |||
Administrative | 8 (6%) | |||
Community work | 5 (4%) | |||
Not employed* | 10 (7%) | |||
Home duties/ carer | 6 (4%) | |||
Other | 19 (14%) | |||
Living situation | 137 | |||
Renting | 58 (42%) | |||
Owned home | 47 (34%) | |||
Living with family | 28 (20%) | |||
Community housing | 2 (1%) | |||
Other | 2 (1%) | |||
Geographic location | 123 | |||
Metro | 100 (81%) | |||
Rural | 23 (19%) | |||
Socioeconomic status^ | 123 | |||
High | 17 (14%) | |||
High-middle | 35 (28%) | |||
Middle | 36 (29%) | |||
Low middle | 19 (15%) | |||
Low | 16 (13%) | |||
AUDIT (total) | 7.51 | 7.03 | 0.00 - 29.00 | 137 |
Class 1 (LRAU) | 3.82 | 2.56 | 0.00 - 13.00 | 99 |
Class 2 (HRAU) | 17.13 | 5.71 | 9.00 - 29.00 | 38 |
DUDIT (total) | 4.96 | 9.25 | 0.00 - 42.00 | 137 |
Note: M = mean, SD = standard deviation.
LRAU = Lower-Risk Alcohol Use; HRAU = Higher-Risk Alcohol Use
* = Unemployed, retired, disabled, or unable to work at present.
^ = Socioeconomic status categories derived from Australian Bureau of Statistics (2018). Five SES categories are indicated, ranging from 1 (most disadvantaged; renamed “Low”) to 5 (most advantaged; renamed “High”).
Latent Class Analysis (LCA) was performed in Mplus 8.3 (Muthén & Muthén, 2017). Procedures for class enumeration were consistent with recommendations, using a combination of fit statistics, including − 2*log-likelihood (− 2*LL), entropy (E), Bayesian Information Criterion (BIC), the Lo-Mendell-Rubin (LMR) adjusted Likelihood Ratio Test (LRT), and Bootstrapped Likelihood Ratio Test (BLRT), as well as considering substantive interpretability and utility (Asparouhov & Muthén, 2012; Nylund-Gibson & Choi, 2018; Nylund et al., 2007). See Additional Information file for further details.
Results
Participant Characteristics
The sample is characterized in Table 1. Participants were aged between 18 and 64, and 80% of the sample were women. As participant responses were included if they scored above zero on either the AUDIT or DUDIT, some participants endorsed alcohol use but not drug use, and vice versa. Table 2 presents participant characteristics between latent class derived alcohol groups. Participants with high severity of alcohol use and related problems (higher-risk) were significantly older than those with lower use and related problems (lower-risk), providing further support to control for age in all regression analyses.
Table 2. Participant Characteristics and Comparisons by Alcohol Group
AUDIT LRAU | AUDIT HRAU | Test Statistic and p value | |
---|---|---|---|
Age (Mdn) | 31 (24, 37.5) | 34 (27, 49) | U = 1364, p = .032 |
Education, Mean (SD) | 16.76 (3.27) | 16.16 (2.66) | t(133) = .99, p = .33 |
Sex (% women) | 80 | 79 | φ = -.03, p = .74 |
DUDIT total (Mdn) | 0 (0, 3) | 2.5 (0,13.25) | U = 1587, p = .13 |
Endorsed illicit drug use (%) | 46 | 53 |
Note. U = Mann Whitney U Test, t = T test; φ = Phi coefficient
LRAU = Lower -Risk Alcohol Use; HRAU = Higher-Risk Alcohol Use
Latent Class Analysis
The results of LCA conducted on the AUDIT are summarized in Table 3. The LCA models did not converge on admissible solutions for the DUDIT.
Table 3. Results of Exploratory Latent Class Analysis of the Alcohol-Use Disorders Identification Test
Classes | LL 1 | Entropy | LMR2 | LMR p | -2*ΔLL | BLRT p 3 | BIC | Pr (H i | D) |
---|---|---|---|---|---|---|---|---|
1 | -1319.547 | 2801.453 | <.001 | |||||
2 | -1066.191 | .975 | 503.701 | < .001 | 506.712 | < .001 | 2462.020 | >.999 |
3* | -1001.116 | .941 | 129.352 | .017 | 130.148 | < .001 | 2499.151 | <.001 |
4* | -964.704 | .943 | 75.982 | .396 | 72.392 | .330 | 2593.606 | <.001 |
Note. LL = log likelihood; LMR = Lo-Mendell-Rubin adjusted Likelihood Ratio Test when comparing the k to k - 1 class model; LMR p = probability value for the Lo-M endell-Rubin adjusted Likelihood Ratio Test. -2* ΔLL = Negative two times the log likelihood difference between k and k - 1 models for the bootstrapped likelihood ratio test. BLRT p = probability value for the bootstrapped likelihood ratio test. BIC = Bayesian Information Criterion; Pr (H i | D) = Bayesian conditional posterior probability of k model compared to all other estimated models. N = 137. Bold typeface denotes preferred model based on converging evidence across fit statistics and substantive interpretability. See Additional Table 2 for more detailed latent class analysis notes.
Latent Class Analysis of the AUDIT
LCA indicated that a 2-class model provided the most parsimonious representation of the latent organization of the AUDIT data into groups. Class 1 (n = 99) was characterized by a high probability of selecting ‘never' for most items (see Additional Figure 2 for item probability plots). This group representing an absence of alcohol use problems and are classed ‘Lower-Risk Alcohol Use' (LRAU). Class 2 (n = 38) was characterized by a more even distribution of probabilities for endorsing AUDIT items across the response categories. The lowest score in Class 2 was 8, which is consistent with clinically significant alcohol use problems (Reinert & Allen, 2007). This group is herein classed ‘Higher-Risk Alcohol Use' (HRAU).
Relationships Between Disinhibition Mechanisms and Alcohol Use Groups
Attentional Control
Hierarchical regression analyses were performed to compare the performance indices of the attentional control task (i.e., D Prime and Criterion C) across LRAU and HRAU groups. For the model with D Prime scores as the outcome (see Additional Table 3), age was entered as a control variable in the model (Block 1). The inclusion of alcohol use group significantly improved the model, ∆F (1, 127) = 5.71, adjusted ΔR2 = .03, p = .018, with the predictive value of the model (Block 2) p = .052. Alcohol use group was the only statistically significant predictor of D Prime scores; HRAU predicted poorer attentional discrimination between target and distractor stimuli.
For the model with Criterion C as the outcome, age was entered as a control variable and did not significantly predict Criterion (C) scores (Block 1), F(1,128) = .10, p = .748, Adjusted R 2 = -.01. Alcohol use group significantly improved the model, ∆F (1, 127) = 6.18, adjusted ΔR2 = .03, p = .014. Although the full model was statistically significant (Block 3), the inclusion of the interaction term did not significantly improve the model; see Table 4. Alcohol use group was the only significant predictor of Criterion (C) scores; specifically, HRAU predicted a more conservative pattern of responding.
Information Gathering
For the upper threshold A outcome, age was entered as a control variable in the model (Block 1). The inclusion of alcohol use group (Block 2) did not significantly improve the model, although including the interaction between age and alcohol use group (Block 3) did significantly improve the predictive value of the model, ∆F (1, 130) = 5.62, adjusted ΔR 2 = .17, p = .019. HRAU was more likely to predict higher upper threshold (a) scores, in older individuals, reflecting a more conservative pattern of responding. Additional Table 4 includes the results and Additional Figure 3 depicts the interaction.
Table 4. Hierarchical Regression Analysis Predicting Attentional Control Criterion (C)
Model | B | SE B | ß | p | 95% CI | Adj. R 2 |
---|---|---|---|---|---|---|
Block 1 | .748 | -.01 | ||||
Age | -.00 | .00 | -.03 | .732 | -.00, .00 | |
Block 2 | .046 | .03 | ||||
Age | -.00 | .00 | -.08 | .390 | -.01, .00 | |
Alcohol (LRAU/HRAU) | .12 | .06 | .22 | .042 | .01, .25 | |
Block 3 | .033 | .04 | ||||
Age | -.00 | .00 | -.04 | .612 | -.01, .00 | |
Alcohol (LRAU/HRAU) | .13 | .06 | .24 | .040 | .02, .27 | |
Alcohol (LRAU/HRAU)*Age | -.01 | .01 | -.15 | .162 | -.02, .00 |
Note. Alcohol Use is dummy coded as 0 = LRAU, 1 = HRAU. Interaction term based on centred variables. CI = confidence interval; CIs and standard errors are based on 1000 bootstrap samples.
For the drift rate outcome, age was entered as a control variable (Block 1). The inclusion of alcohol use group (Block 2) and the interaction term between alcohol use and age (Block 3) did not significantly improve the predictive value of the model. Age was the only significant predictor; older age was associated with a higher drift rate, and a tendency to acquire a greater amount of information prior to making a decision. See Additional Table 5 for results.
Feedback Monitoring/Shifting
For the hierarchical model with feedback monitoring/shifting perseverative errors as the outcome, age was first entered as a control variable (Block 1). The inclusion of alcohol use group significantly improved the model, ∆F (1, 129) = 6.00, adjusted ΔR2 = .04, p = .016, with the predictive value of model (Block 2) p = .050. The inclusion of the interaction between age and alcohol group (Block 3) did not significantly improve the predictive value of the model. Alcohol use group was the only significant predictor of perseverative errors; HRAU was associated with a greater number of perseverative errors. See Additional Table 6 for results.
Exploratory Analyses
As our sample was unique in including a high proportion of women, exploratory correlation analyses between CIS indices and alcohol use were conducted by sex. There was a significant correlation between Criterion (C) scores and alcohol use group in men but not women, r pb (24) = .57, 95% CI [.22, .79], p = .002, where men with HRAU had a more conservative response bias on the attentional control task than those with LRAU. Conversely, there was a significant relationship between upper threshold scores and alcohol use in women but not men on the information gathering task, r pb (107) = .26, 95% CI [.07, .43], p = .007. Women with HRAU responded more conservatively on the information gathering task than women with LRAU. There was a significant relationship between proportion of perseverative errors and alcohol use in men but not women, r pb (24) = .46, 95% CI [.07, .73], p = .017. Men with HRAU had greater perseveration errors than those with LRAU, but this relationship was not seen in women.
Discussion
We investigated associations between severity of alcohol use and disinhibition mechanisms (i.e., attentional control, information gathering, feedback monitoring/shifting) among an online help-seeking sample recruited via a web-based national online support service. Our recruitment and testing platform facilitated a broader geographical reach and participation from consumers who are underrepresented in traditional addiction treatment services, including women and people engaging in lower risk use. As predicted, HRAU was associated with poorer performance on CIS tasks of attentional control and feedback monitoring/shifting. Unexpectedly, HRAU was associated with a more conservative, rather than impulsive, response style on an information gathering task, but only in older participants. We discuss our findings below, with consideration of the proportion of women included in key studies due to the high representation of women in this study.
Our finding that HRAU was associated with significantly poorer discriminability on the measure of attentional control is consistent with previous research in men and women on a similar Go No-Go task (with 30% - 52% women in each study; Ames et al., 2014; Claus et al., 2013). People with HRAU showed low sensitivity to differentiating signals from distractors in the task, suggesting that they find it difficult to direct attention towards relevant stimuli. Surprisingly, those with HRAU displayed a more conservative response style, with higher Criterion scores (reflecting a more conservative approach to response triggering) compared to LRAU. Generally, lower Criterion scores are indicative of a more impulsive response style (Ratcliff & McKoon, 2008). However, in our sample of people engaging in HRAU, reduced discriminability may have driven a compensatory deferral of response. In an inpatient detoxification sample (20% women), heavier patterns of alcohol use were associated with longer decision latency (Czapla et al., 2016). Furthermore, men and women with alcohol use disorder display elevated activity in the prefrontal portions of the salience network during prolonged decision-making time, consistent with a compensatory response (Canessa et al., 2021; Stavro et al., 2013).
HRAU was also associated with a significantly higher number of perseverative errors on the measure of feedback monitoring/shifting, reflecting difficulty in monitoring the consequences from previous decisions and updating of future responses accordingly (Sharma et al., 2014). Both initial learning and subsequent monitoring of response-outcome relationships are modulated by dopaminergic transmission in fronto-striatal circuits (Volkow & Li, 2004). Higher-risk alcohol use has been associated with significant downregulation of dopamine transmission in the ventral striatum in both men and women (Soder et al., 2019; Volkow et al., 2007), resulting in a diminished sensitivity to errors and re-enactment of non-planned disadvantageous responses (Poulton & Hester, 2020). This mechanism underpins motivational aspects of addiction, namely the difficulty to utilize feedback from previous actions and choices to prevent recurrent impulsive responses, which can be linked to both positive (e.g., during the binge / intoxication phase) and negative reinforcement mechanisms (e.g., during the withdrawal and craving phases; Kalhan et al., 2021; Zorrilla & Koob, 2019).
Although previous research has compared information gathering abilities in men and women with and without alcohol use disorders (Banca et al., 2016; Mandali et al., 2019; Townshend et al., 2014), to the best of our knowledge we are the first to examine the latent cognitive mechanisms underpinning information gathering by varying levels of alcohol severity and related problems. Our findings highlight a unique age-dependent relationship, with younger participants within the HRAU class gathering less information before acting, while older participants showed the reversed pattern. Our findings for younger participants extend previous research (including 43% - 53% women) highlighting poorer reflection impulsivity in young adults who binge drink, compared with healthy controls or people engaging in lower-level binge drinking (Banca et al., 2016; Townshend et al., 2014). Increased gathering of information in older participants likely reflects age-dependent changes, where older adults place a greater emphasis on response accuracy than reward rate (Dully et al., 2018; Theisen et al., 2021). However, people engaging in higher-risk alcohol use may experience relatively poorer efficiency in sampling information due to cognitive damage attributable to long-term alcohol use (Kim et al., 2012). Taken together, these findings suggest the need for further research on inter-individual differences in information gathering among people with alcohol use problems.
There were a number of strengths in our study. Our study included 19% of consumers (n = 23) living in rural areas. Rural-based consumers are often missed from traditional clinical research, which frequently recruits from metropolitan addiction services or university settings (e.g., Ames et al., 2014; Kopera et al., 2012; Rodriguez-Cintas et al., 2016). Limited available addiction services in these areas is also a key barrier to seeking help (Ashford et al., 2018; Australian Institute of Health and Welfare, AIHW, 2019). Online addiction support services (e.g., Counselling Online) are ideally suited to overcoming barriers to accessing support, helping to generate research findings that are relevant to a wider population.
We also engaged a much larger proportion of women (80%) than those recruited in traditional treatment seeking settings in Australia (36%; AIHW, 2021), supporting the potential utility of online treatment to reduce barriers for women (e.g., childcare commitments, social stigma; Taylor, 2010). Similarly, we reached a broader age group than targeted recruitment typically permits and did not impose a maximum eligible age (frequently imposed in cognitive research), allowing greater insight into the interaction between age and alcohol use and how these relate to inhibition processes. Both represent important contributions, as women and older adults are currently underrepresented in this area of research and the disproportionately high percentage of young men in treatment and research studies is not reflective of real-world alcohol and substance use patterns. Further, we recruited a larger number of treatment-seeking individuals relative to comparable in-person studies (Hagen et al., 2019; Ridley et al., 2017). Our online recruitment and testing are robust to COVID-19-related changes in research (e.g., social distancing and remote-work requirements) and are therefore well-suited to continuing otherwise prohibitive research. Finally, our novel and highly engaging impulsivity task is well suited for online administration (Verdejo-Garcia et al., 2021) and providing a deeper insight into the mechanisms relating to real-world impulsive behaviors (i.e., patterns of alcohol use).
Notwithstanding the advantages to online recruitment and testing, it does prohibit biological verification of alcohol use levels and relies on honest and attentive self-reported responses. Additional attentional checks throughout the study may have enhanced confidence in the self-reported responses (Strickland & Stoops, 2019). Although our sample (including a uniquely high proportion of women) captured a broader audience than those engaged in traditional face-to-face services, results may not generalise to people with substance use disorders who are currently not seeking treatment. Our self-selected sample comprises a large proportion of participants with lower-risk alcohol use as indicated by AUDIT scores, and although we intended to examine the link between severity of drug use and related problems and disinhibition, the degree of self-reported drug use did not allow meaningful analyses. Further screening questions to identify a minimum level of alcohol / substance use could help to capture more severe substance use patterns. Additionally, screening questions gathering information about current treatment engagement (e.g., medication or behavioral interventions), and patterns of alcohol use (e.g., in the past three months) may have indicated whether other treatment approaches were predictive of disinhibition processes and whether disinhibition processes were less pronounced in those who were recently abstinent (Bartsch et al., 2007; Pitel et al., 2009; Schmidt et al., 2016). Finally, 48% of participants in our sample endorsed using drugs as well as alcohol. As alcohol and different drug classes (e.g., opiates, amphetamines, barbiturates) exhibit different patterns of cognitive impairment (Bruijnen et al., 2019), the impairments we observed may not be strictly attributable to alcohol use alone. However, comorbid substance use disorder is common in those with alcohol use related problems (Moss et al., 2010), and therefore the deficits we observe may be of relevance to people seeking help for polysubstance use, although with alcohol use as a primary concern.
Gaining a deeper understanding of how cognitive inhibition processes are disrupted across varying levels of at-risk alcohol use may help treatment providers to improve individualized predictions of treatment outcomes and select interventions that specifically target the disrupted mechanism (Verdejo-Garcia et al., 2019). For example, older adults with higher-risk alcohol use may require more time to process concepts carefully in behavioral treatment. Additionally, adults engaging in higher-risk alcohol use may benefit from building metacognition and to practice identifying and evaluating positive and negative consequences of thoughts and behaviors, to encourage more flexible on the spot problem solving. Our web-based approach also provides substance use related information services with novel and more individualized ways of reaching out to those in the earlier stages of help-seeking, those with barriers to accessing services, and those who are hesitant to engage in treatment. Consumers may respond more actively to information that reflects their disinhibition profile, which may ultimately help to improve the reach of public health strategies by encouraging uptake of support services.
Overall, our findings provide novel insights into the relationship between alcohol use severity and disinhibition mechanisms in individuals seeking online support. We found that people with HRAU, compared to those with LRAU, had poorer attentional control and monitoring of feedback from past behaviors. They may attempt to compensate for weakened attentional control with more conservative deliberation; this was particularly true for older adults with HRAU who gathered more information before responding. Leveraging a help-seeking web portal for online recruitment has permitted us to reach a sample with distinctive characteristics to those recruited through traditional face-to-face treatment services. Further research is needed to replicate our findings and to extend the clinical utility of assessing cognitive disinhibition processes across the broader help-seeking community.