INTRODUCTION
On December 31st, 2019, the People's Republic of China announced the detection of cases of pneumonia secondary to infection due to a new coronavirus, later named SARS-Cov-2. On March 11th, 2020, the World Health Organization (WHO) declared the infection for COVID-19 as a pandemic [1].
Up to July 3rd, 2021, Argentina had reported 4,526,473 confirmed cases of COVID-19, and 95,594 deaths. [2].
According to a national epidemiological study of patients with a confirmed diagnosis of COVID-19, 20.1% of them were hospitalized in general ward, 2.73% required admission to the Intensive Care Unit (ICU), and 5.27% died. [3].
At a national level, the specific mortality rate was of 4.70 COVID-19-deceased persons per 100,000 inhabitants, according to an epidemiological surveillance study [4].
At the onset of the pandemic, multiple papers were published, which tried to describe the natural history of the disease and to identify patients with a higher risk of poor outcomes (PO). Most of these studies originated in China [5, 6, 7, 8, 9, 10, 11]. As COVID-19 spread worldwide and countless outbreaks challenged health systems, identifying these patients became a priority in order to take the necessary medical decisions.
However, to this day, there is no consensus on how to successfully predict which patients are more likely to require mechanical ventilation or die during hospitalization, even though there is an increasing number of studies available proposing multiple algorithms and predictive variables. Most studies only show moderate predictive capacity. Moreover, there is scarce evidence available regarding the reproducibility of these findings in the latin-american population.
Therefore, our objective was to identify clinical and biochemical predictors of PO, in a cohort of patients hospitalized due to COVID-19 in a tertiary care center in Santa Fe province, Argentina.
METHODS
This cohort study was conducted prospectively, from March 3rd, 2020 to February 16th, 2021 in a tertiary care center in Santa Fe, Argentina. We included all patients older than 16 years of age with COVID-19 pneumonia consecutively admitted to the hospital, whether to the General Ward or the Intensive Care Unit (ICU).
Diagnosis of COVID-19 pneumonia required radiological evidence of new infiltrates and a nasopharyngeal swab with positive polymerase chain reaction for SARS-Cov-2. Patients with negative polymerase chain reaction were also included if they presented with typical lung CT findings [12, 13], in the context of community transmission of the virus. In the absence of community transmission, they were included if they belonged to a cluster of COVID-19.
Two epidemiological periods of time of the pandemic were analyzed, a first stage (S1) between the months of March and October 2020, and a second stage (S2) between November 2020 and February 2021.
DATA COLLECTION
Data collection was performed by routinely reviewing patients' medical records during their hospitalization. All clinical data at admission was included: general information, epidemiological history, clinical characteristics, biochemical markers, lung images and treatments administered. Access to medical records was granted by the Hospital Director's Office, and the conducting of the study was approved by the Hospital's Teaching Committee.
Clinically relevant comorbidities were selected based on available literature [14, 15, 16]: hypertension (HT), diabetes (DBT), obesity, Chronic Obstructive Pulmonary Disease (COPD), active cancer, Chronic Kidney Disease (CKD) and Ischemic Heart Disease (IHD).
4C-score of in-hospital mortality was calculated for all patients [17].
OUTCOMES
For the primary analysis, outcomes were assessed at hospital discharge, death or transfer to another facility. The primary outcome of interest was a composite of poor outcomes (PO), comprising all-cause mortality and/or need for invasive mechanical ventilation (IMV).
A secondary analysis was conducted, for exploratory purposes, on the characteristics of the sub-population of patients older than 65 years of age.
STATISTICAL ANALYSIS
Categorical variables were analyzed with Chi2, Fisher or Fisher-Freeman-Halton tests. Post-hoc analysis for multinomial variables was carried out with z-tests with Bonferroni correction.
Distribution of frequencies was analysed and the normality of the variables was determined by the Kolmogorov-Smirnov test. Quantitative variables were expressed as mean ± standard deviation or as median and interquartile range, according to normality. T-test for independent samples and Mann Whitney U-test were performed, as appropriate.
A logistic regression was performed for multivariate analysis, including those clinical and biochemical variables with a p < 0.05 value in the bivariate analysis, prioritizing those variables with the smaller data loss.
A ROC curve was carried out, considering the variables that were statistically significant in the logistic regression.
Statistical analyses were conducted, using the SPSS Statistics v27.0 (IBM) programe and StatCalc.
Figures were generated in Microsoft Excel and GraphPad Prism 8.
RESULTS
421 patients hospitalized due to pneumonia for COVID-19 were included.
Mean age was 56.13 ± 15.05 years. 31.7% of the patients were older than 65 years of age. 57.5% were male. 79.7% presented at least one comorbidity and 47.3% presented two or more. The main clinical characteristics and the results of the bivariate analysis are shown in Table 1.
Table 1. Comparison of clinical characteristics between patients with poor outcomes and those without
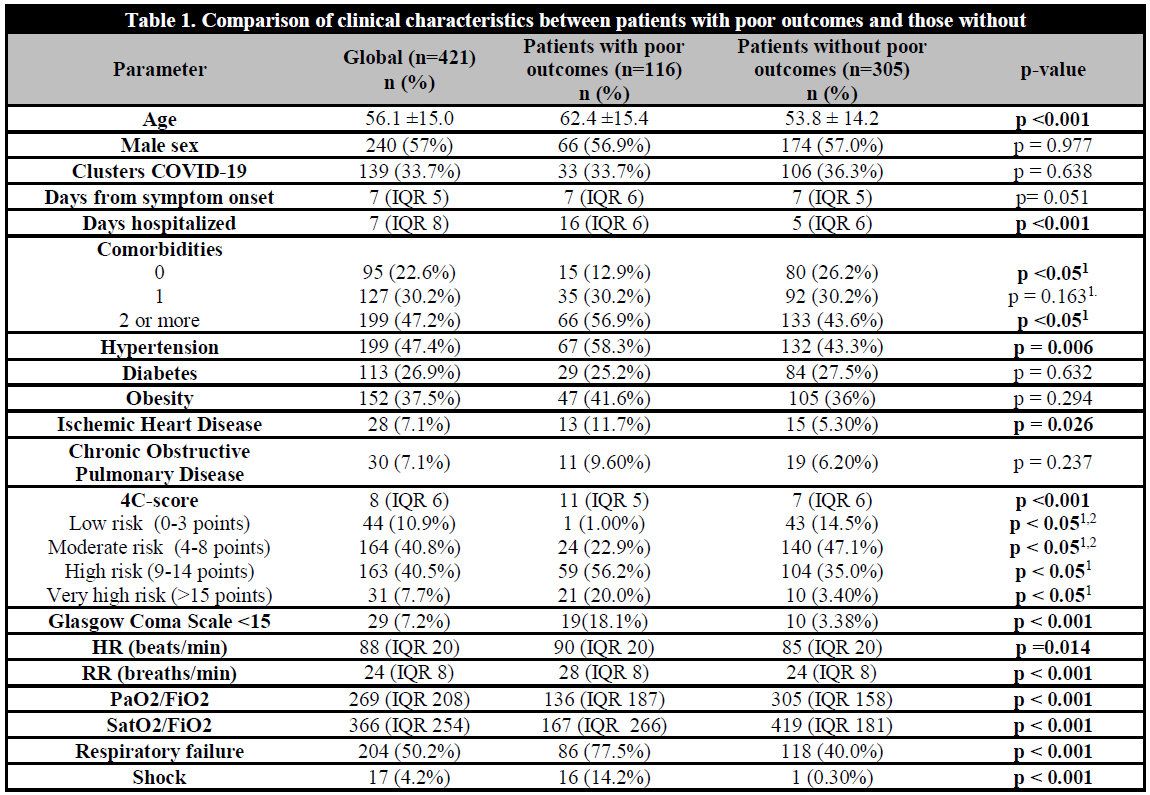
1p Value for post-hoc comparisons. 2In the post-hoc analysis there was no significant difference in the risk of poor outcomes between the groups with 4C-score in the categories of low and moderate risk.
27.7% (n=116) presented PO (all-cause mortality and/or need for IMV). 23.7% (n=99) received IMV. Mortality was of 18.8% (n=79).
29.1% (n=122) were admitted to ICU at any moment during their hospital stay, 81.0% of these received IMV - with a median stay in ICU of 4 days (IQR 15), and a maximum stay of 57 days. Patient mortality in the ICU was of 59.8% (n=68).
23.9% (n=99) of patients were diverted to other medical centers, and in 46.8% (n=46) of the cases, follow-up was lost. Total mortality ascended to 21.4% (n=90) if the outcome of patients diverted to other medical centers was considered.
Taking into account the two stages analyzed, mean age in S1 was lower (54 ±14.8 years vs 59.9 ± 14.7 years, p <0.001) and the frequency of obesity was higher (41.9% vs 29.3%, p <0.009). S1 presented a lower frequency of respiratory failure at admission (42.7% vs 64.3%, p <0.001) and a lower frequency of sepsis (52.4% vs 80.1%, p <0.001), with a numerically lower mortality rate between the groups (17.2% vs 23.7%, p = 0.054).
The mean age of patients with PO was higher (62.4 ± 15.4 vs 53.8 ± 14.2 years, p<0.001). This difference was maintained if each component was analyzed separately.
Table 2 stratifies the risk of PO, IMV and mortality based on the number of comorbidities and the risk category according to 4C-score.
Table 2. Number of comorbidities and categorization of the risk of in-hospital mortality by 4C-socre, in relation to poor outcomes, invasive mechanical ventilation, all cause mortality
Table 3 shows biochemical characteristics of patients and highlights the differences found in patients with PO in the bivariate analysis.
In the multivariate analysis, a higher 4C-score and a higher value of LDH, as well as a lower SatO2/FiO2 were associated with a higher risk of PO. For each point that the 4C-score increased, the risk of PO raised by 28%. Also, for every 100-units increase in LDH or 50-units decrease in SatO2/FiO2 at admission, there was a 20% increased risk of PO (Table 4). In a second analysis, when the 4C-score was excluded from the model, age became statistically significant.
Table 4. Multivatiate analysis of predictor variables of poor outcomes
Predictive capacity of the model: Nagelkerke's R2 0.383.
A ROC analysis was performed using as predictor variables age, 4C-score, PaO2/FiO2, SatO2/FiO2 and LDH. No variable reached an AUC of 0.800. 4C-score presented an AUC numerically higher (0.766 CI 95% 0.715-0.817). It was found that the 4C-score AUC and the SaO2/FiO2 AUC were significantly higher if compared to the age AUC (Table 5, Figure 1).
Table 5. Predictor variables of poor outcomes
Area under the curve (AUC), of ROC (Receiver-Operator Curve). The cut-off points are presented optimized through ROC analysis and the values chosen to increase specificity (aligned to the right). Sensitivity and specificity of cut-off points are also presented.
A 4C-score of 15 or higher presented a specificity of 97.5% for PO. Also, reducing the cut-off point of PaO2/FiO2 and SatO2/FiO2 to 100 allowed for a specificity of 91.3% and 88.9% for PO, respectively.
In the analysis of a subgroup of patients older than 65 years of age, 39.8% presented PO, 31.6% died, 31.5% received IMV and 38.2% were admitted to ICU -among these, mortality ascended to 71.4%. In a post-hoc analysis, the biochemical variables studied behaved differently if compared to patients younger than 65 years of age (Table 6).
DISCUSSION
In this study, we presented a prospective cohort of 421 patients admitted to a tertiary care center with the diagnosis of COVID-19 pneumonia, the majority of which were severe. 27.7% of patients presented PO (18.8% died and 23.7% received IMV during hospital stay).
The primary finding was that, in the multivariate analysis, for each point that the 4C-score increased the risk of PO raised by 28%. Also, for every 100-units increase in LDH or 50-units decrease in SatO2/FiO2 at admission, there was a 20% increased risk of PO.
Although since the onset of the pandemic, multiple papers suggested models for prediction of PO/mortality in patients with COVID-19 [17, 18, 19], the strength of our score lies in the fact that we included patients only after their hospital stay had finished (discharge or death), unlike others that included patients who have not yet reached the final event.
However, contrary to what was expected and to what has been described in developed predictive models [19], lymphopenia was not associated with a higher PO risk in the multivariate analysis.
Moreover, when the model was re-analyzed excluding the 4C-score, age became significant, which showed co-linearity with the 4C-score and the better performance of
the latter due to the inclusion of the other variables assessed by it. Surprisingly, sex, comorbidities and urea did not show a better performance when 4C-score was excluded, which probably reflects a type-2 error, or the small effect of these variables, whose influence is presumably, additive. It highlights the additive value of LDH to 4C-score, in all likelihood as a reflection of the severity of pulmonary involvement [20, 21, 22, 23, 24].
This paper, on its own, cannot clarify whether the lack of predictive value of other variables, in particular, the lymphocyte count, depends on the multicollinearity with other variables, on its role only as a marker of underlying phenomena or on a lesser predictive role that requires a higher inclusion of patients to be detected.
In the context of a pandemic, predictive models have the added value of being necessary for decision-making: early hospitalization, early admission to ICU/early IMV, patients triage in case of the health system being saturated. For all these, a predictive model should be able to identify with high specificity, those patients that will develop the assessed event - since otherwise there would be a risk to implementing more aggressive conducts [17] with patients that would have a favorable outcome. Similarly, the models destined to define early discharges should also be highly specific. This need to prioritize sensitivity or specificity originates in the fact that there are no optimal models in the literature and the better designed scores present an AUC/ROC under 0.800 [17, 18, 25, 26].
In the ROC analysis, individually, age, 4C-score, PaO2/FiO2 and SatO2/FiO2, and LDH presented a predictive capacity for PO. Age was the variable with the worst diagnostic performance.
It is worth noting that a 4C-score of 14 or more and a PaO2/FiO2 or SatO2/FiO2 lower than 100 presented high specificity for PO.
We observed a positive relationship between age and risk of PO and mortality, in accordance with other series [18, 25, 26, 27]. However, in a post-hoc analysis and contrary to reports from a large series of ICU patients, age was not related to a higher risk of mortality in this subgroup [28, 29].
Different hypotheses have been put forward to explain the increasing risk of developing PO with older age. Among them, we can mention: the influence of pulmonary physiological changes [30] with different response and tolerance to noxas [31] and immunosenescence [32]. The presence of crossed CD4+ immunity seen in younger persons, in the absence of CD8+ and humoral responses, is suggested as another tentative explanation for the influence of age in the risk of PO [33], even when evidence is contradictory [20, 34, 35, 36].
Contrary to other series [18, 28, 37] and multinational epidemiological analyses [38], no association was found between sex and PO or in-hospital mortality. It should be highlighted that even though the aforementioned meta-analysis found a higher mortality in males, these were a group that presented a higher prevalence of comorbidities, a fact that did not replicate in this series and could explain the lack of association with PO.
This cohort presented a high rate of comorbidities, the same as other series of patients hospitalized due to COVID-19 at a national [3] and international [39] level. In the bivariate analysis, to present at least one comorbidity was statistically associated with a higher risk of PO, need for IMV and all-cause mortality. Among them, HT was the most frequent comorbidity found (47.4%) and it was statistically associated with PO, coinciding with reports from other series [19, 27, 40]. Diabetes was not associated with PO in this cohort, while the antecedent of ischemic heart disease - which has been included in prognostic scores of COVID-19 [18] was an infrequent finding however associated with PO in the bivariate analysis.
We highlight the fact that no comorbidity, isolated or in group, was predictive of PO in the multivariate analysis. This is opposed to previous studies [41] and could be due to a type-2 error or to particularities in the population. On the other hand, the fact that diabetes was not related to PO neither in the bivariate nor in the multivariate analysis, in spite of them being adequately represented, is unlikely to have been caused by the size of the sample. As regards hypertension, it is considered a debated risk factor - sometimes thought of as an epiphenomenon- which in some studies did not present association with PO nor mortality after adjustment by other factors [42].
In most patients, the diagnosis of obesity was clinical and was due to the fact that the clinical condition of the patients made it difficult to measure the BMI. There was no association with PO, probably because the lack of registry of BMI limited the possibility of analysis of the variable, since this variable was considered categorically.
Among the multiple predictive models developed for COVID-19, 4C-score - which was derived from a large British series of 57,824 patients hospitalized with the objective of predicting in-hospital mortality [39] - was evaluated given that its predictive capacity is higher than other models´. In our cohort, 4C-score was associated with PO. This association increased throughout the risk categories defined by the score. It is noteworthy that the median age in this large British cohort is 16 years older than the one found in our series, even though the difference in life expectancy between the two countries is of only 5 years [43, 44]. This observation limits its extrapolation. Up to this date, this is the first paper to describe the behavior of this score over Argentinean population.
In the bivariate analysis, patients with PO presented an hemogram related to the activation of the immune system in the context of severe viral infections (leukocytosis, lymphopenia, higher leukocyte-lymphocyte and platelet-lymphocyte ratios). Patients with PO also showed elevated urea values with no changes in serum creatinine levels, which allows us to suspect that these changes do not depend on kidney function. Other markers like LDH, CK, D-dimer, procalcitonin, troponin T and ferritin also were significantly higher in patients with PO.
The association of these variables with PO and mortality in the bivariate analysis is in agreement with what was found in most series of COVID-19 in the rest of the world [45, 46].
The neutrophil-lymphocyte ratio, in particular, was found associated with a higher mortality in other series and has been used for the creation of predictive models [45].
In a post-hoc analysis, patients older than 65 years of age in our series presented higher levels of hs-CRP and a lower lymphocyte count - which constitute the most consistent findings in different series - but did not present higher LDH values if compared with younger patients [47]. As it was described in the literature, the lymphocyte count did not present changes if compared to younger patients, even when they showed a higher leukocyte-lymphocyte and platelet-lymphocyte ratio.
Levels of troponin T, D-dimer and procalcitonin were found higher than those found in patients younger than 65 years of age, a fact that was not found in previous publications.
Of note, two epidemiological stages were described, S1, period in which there was a lower number of cases in the city and patients were hospitalized for epidemiological blockade purposes; and S2, when the number of cases increased exponentially and our medical centre only admitted patients with moderate to severe COVID-19 disease. Patients presenting mild cases of the disease were derived to less complex medical facilities. During S1, admitted patients were younger but with a higher number of comorbidities, probably due to the different criteria for hospitalization used. These patients showed lower incidence of respiratory failure and sepsis.
CONCLUSIONS
In this study, we found that each point that the 4C-score increased, the risk of PO raised by 28%. Also, every 100-units increase in LDH or 50-units decrease in SatO2/FiO2 at admission, there was a 20% increased risk of PO.
Even when arterial gasometry is not widely available, pulse oximetry - which allows for a reliable approximation - indicates rather than predicts the development of PO. Therefore, other biochemical parameters need to be identified to act as early markers of PO risk. Here we showed that LDH independently predicts PO
However, similar to the finding of other studies, there is neither clinical characteristic nor biochemical test that allows for an ideal classification of patients. In this context, the inclusion of a high number of variables in models in order to increase the diagnostic performance, not only generates loss of patients but also limits its utilization in clinical practice. In our case, the lack of resources limited the amount of biochemical analyses conducted, which forced us to exclude some variables from the multivariate model in spite of its statistical significance in the bivariate analysis.
This cohort of patients hospitalized due to COVID-19 comes forward for being one of the few ones that assessed PO predictors in Argentina.
Future studies need to assess the impact of strategies based on early laboratory screening for clinical decision making and to clarify whether these results are reproducible in other medical centers in Argentina and Latin-America.