Types of design in program evaluation
A program can be broadly defined as a series of actions (an intervention) which aims to address an identified problem of some sort (Chacón-Moscoso et al., 2013). The intensity of this intervention can vary enormously depending on the situation, which also determines the procedure that shall be used. These situations can range from the lowest level of intervention (daily routines continue as usual, i.e., program users are not asked to alter their behaviors) to the highest (may be applied in experimental contexts with a low degree of ecological validity, in which the program interventions are akin to the independent variables of a randomized control trial -RCT-).
Given that random assignment enables an unbiased estimation of program effects and is justified according to the theory of significance tests, it could be argued that the different designs can be ranked by their quality (although two epistemologically different approaches are assumed to be the starting point). This ranking would be based on knowledge of the assignment criterion or on the procedures used to avoid correlation between the error term and the parameters to be estimated. Nevertheless, the issue is not merely one of random versus non-random, since, for example, evaluations based on randomized designs must be properly executed and take into account the conditions of application, e.g., they must follow an adequate randomization process, there being no treatment-correlated attrition, differences at pre-test, or partial implementation of treatments (Shadish, 2002). Likewise, non-randomized evaluations may yield results similar to those of randomized ones when, for example, they include the matching of relevant and reliable covariates, with the added bonus of greater external validity.
Instead of rejecting one of the possible designs at the outset (Chacón-Moscoso & Shadish, 2001), the feasibility of each design should be examined in every case. Once the most suitable design has been chosen based on the type of evaluation (process, result, etc.), the practitioner estimates the program component, considering the different characteristics of each design rather than simply an overall methodology (experimental/quasi-experimental vs. observational/qualitative). As it is impossible to list all the possible contingencies, it is also necessary to understand which methodological and substantive factors may contribute to the analysis of outcomes, and to determine the weight of these factors in each unique design type.
Two methodological approaches underlie the different points along of a continuum. If we start from the highest level of intervention and move toward the lowest, the programs evaluation requires the use of an experimental or quasi-experimental (Q-E) design at a high level of intervention (Cook & Campbell, 1979). The analysis that follows, in medium/high intervention programs, is based on the validity framework proposed by Shadish et al. (2002) for generalizing causal inference. Although not all the N-E aim to obtain this causal generalization, all types of validity (statistical conclusion, construct and external validity) except internal validity can be applied to non-experimental (N-E) studies, as much as they can to random experiments (R-E) or Q-E. We will also borrow from the UTOSTi (units, treatment, outcome, setting, and time) system of structural design dimensions (Chacón-Moscoso & Shadish, 2001), which was first introduced by Cronbach (1982) to compare different kind of studies. Finally, with this comparative analysis as a point of reference, we describe the main aspects to consider in each methodology, taking into account the design mutability (Anguera, 2001).
On the other hand, in low-intervention program evaluations, certain situations require an idiosyncratic approach to observational methodology, both direct (information contained basically in images is required, which provides a high level of discernment) (Sánchez-Algarra & Anguera, 2013) or indirect (based mainly on texts) (Anguera et al., 2018). Observational methodology is considered a mixed method, given that it involves three macro-stages: qualitative data are gathered and then transformed into quantitative data, at which point a qualitative interpretation is made (Anguera et al., 2020). Therefore, the starting point and the entire data collection stage will be carried out over the course of the program interventions, which will all be low intensity. This context obliges us to take into account a reliability framework that certain idiosyncratic validity models for qualitative studies partially support, considering that “a qualitative study cannot be assessed for validity” (Onwuegbuzie & Leech, 2007b, p. 238). And “partially” is particularly important here because it is relevant to note that these are not qualitative studies, though their first macro-stage is qualitative, as indicated above.
Anguera (1995) describes the designs that can be proposed in the evaluation of low intervention programs; these are the result of comparing the criteria of users or audiences (vertical axis) and temporality (horizontal axis), obtaining diachronic designs (quadrant I), synchronic designs (quadrant III), and lag-log or mixed designs (quadrant IV), all ongoing. Each of these designs has been widely used in program evaluation in several settings over the last 25 years.
This work presents an innovative proposal to apply methodological complementarity and quality in program evaluation. Different congresses on methodology (organized by the Mixed Methods International Research Association, the Spanish Association of Methodology of Behavioral Sciences, the European Association of Methodology, the Research Network on Methodology for the Analysis of Social Interaction, etc.) have discussed innovations in this regard. A brief report of these advances was published in Chacón-Moscoso et al. (2014). This paper aims to propose a framework for program evaluations from two methodological approaches often contrasted from one another, to make decisions about design, measurement and analysis in unstable intervention conditions based on structural dimensions from a practical methodological quality perspective.
Basic concepts associated with validity types
From the methodological point of view, we continue with the aforementioned differentiation based on the degree of intervention.
Referring to high-intervention program evaluation (Chacón-Moscoso & Shadish, 2001; Shadish et al., 2002), statistical conclusion validity is defined as inferences based on the association treatment/outcome in the sample. Internal validity examines whether this relationship is causal; construct validity considers whether the previous studied relationships can be generalized from the sample and applied to the reference population; and external validity assesses whether the findings from the sample can be generalized to other sub-samples or populations.
In low-intervention programs, based on the Qualitative Legitimation Model by Onwuegbuzie and Leech (2007b), which we adopt as a partial reference, elements of internal and external credibility are integrated, which allow the level or degree of reliability to be modulated, though these lists are by no means exhaustive.
Considering the characteristics of the low-intervention program evaluations, certain features of the Qualitative Legitimation Model correspond to internal credibility and are applicable to the first stage of the process, i.e., data collection. These are a) descriptive validity (Maxwell, 1992), b) structural corroboration (Eisner, 1991), c) theoretical validity (Maxwell, 1992), d) observational bias (Onwuegbuzie, 2003), e) researcher bias (Onwuegbuzie, 2003), f) reactivity (Onwuegbuzie, 2003) and g) effect size (Onwuegbuzie, 2003). Regarding external credibility, the features include a) investigation validity (Kvale, 1995), b) interpretive validity (Maxwell, 1992), c) consensual validity (Eisner, 1991), d) population generalizability/ecological generalizability/temporal generalizability (Onwuegbuzie & Daniel, 2003), e) researcher bias (Onwuegbuzie & Leech, 2007b), f) reactivity (Onwuegbuzie & Leech, 2007b), g) order bias (Onwuegbuzie & Leech, 2007b) and h) effect size (Onwuegbuzie & Leech, 2007a).
Strategies derived from these elements make it possible to improve the legitimacy of low-intervention programs evaluation in the initial stage of the program. Among them, we highlight a) prolonged engagement (Glesne & Peshkin, 1992), which allows diachronic modulation of the data collection time through observational records; b) persistent observation (Onwuegbuzie & Leech, 2007b), which will be materialized in the fineness of the observation instrument, c) an audit trail (Halpern, 1983), collecting direct observation records and different indirect observation materials, and d) testing its representativeness (Onwuegbuzie & Leech, 2007b), which allows the representativeness of the results to be increased by modifying the facets of the study, such as the number of observers, the number of observation sessions, etc.
Structural dimensions in program evaluation
The first step of medium-high intervention program evaluations is to reflect on the elements that should be incorporated in the design to heighten the validity and increase the possibility of generalizing our results. Once an initial design structure that underscores the validity of the process has been drafted, it will be possible to analyze the degree to which our results can be generalized.
UTOSTi constitutes the basic structural dimensions that serve as a reference for evaluation designs. However, it is important to consider the specific limits required for each individual evaluation program. In this regard, various factors (for example, the degree of commitment to the program, drop-outs, the effect of friends and family on users, or the need to reconsider the treatment after a given point) can have a wide range of impacts. Therefore, each design proposal must be adjusted so that the program is suited to the actual setting (Chacón-Moscoso & Shadish, 2001).
Table 1, which sets out the structural elements, shows that each evaluation design should consider a series of aspects that will enhance the ability to generalize the results obtained a priori.
These structural dimensions provide a theoretical framework for evaluation that ensures consistency in the different designs at a conceptual level although, in practical terms, their implementation requires certain adaptations. Indeed, innumerable aspects will have to be dealt with differently depending on how the gathering of certain data is contemplated in the design. Different types of instruments may then have to be implemented in different ways to obtain the data; for example, categorical data proves complex under certain circumstances. The instruments used yield different types of data that will require various techniques for quality control, and in accordance with these techniques, a wide range of analytical procedures may be used, always with the aim of providing a firm basis for the results obtained.
In light of the above, the importance of a strong interaction between the theoretical framework and the empirical implementation cannot be underemphasized, with the aim of ensuring that the empirical work bears the conceptual load as efficiently and feasibly as possible. This paper seeks to link these two broad components and to convey the importance of their interaction by reflecting on the possibilities on applying them.
Differences may arise in terms of the different intervention levels (high, medium, low) because these relate to a specific approach. To some extent, this may be modulated by the program setting, though in essence the intervention levels depend on what design the practitioners choose. Similarities will be observed because the full set of designs can be represented as a continuum; each different design has its place alongside others. By analyzing the relationships between them, common elements can be identified that will facilitate their overall integration (Anguera & Chacón-Moscoso, 1999).
In low-intervention program evaluations, the UTOSTi model is not totally applicable, and adaptations that can be verified by the different methodological decisions will be required.
Structural dimensions of evaluation designs in relation to high and medium-level interventions
The general aim of evaluative designs involving a medium-level intervention is to draw causal inferences about program effects, whereas the main objective of R-E (high-level interventions) is to analyze those variables or factors that produce variations in the study variables of interest (Cook & Campbell, 1986).
Broadly speaking, the necessary conditions for conducting an R-E or a Q-E are having a theoretical model or prior knowledge, ensuring that the program is implemented as planned, and having suitable procedures in place to measure the dependent variable in the intervention context. With respect to the structural design dimensions, this involves the following:
Units (Users)
Criteria for the selection and assignment of users. Research in the form of R-E emphasizes the need for as much control as possible over all those variables that may influence a given study. Therefore, the selection method for the units which the program is designed to impact is rendered explicit, along with how these units have been assigned to the interventions or treatments. Experimental models can be categorized into two broad blocks: R-E (high-level intervention) and Q-E (medium-level intervention). In R-E, the experimental units are strongly advised to be selected at random and have to be assigned in a randomized way to the different study groups/conditions; thus, the procedure for assigning users is entirely known. In Q-E, by contrast, the units may be selected by means of known or unknown criteria, and the users' allocation to groups is rarely random; this is because users normally already belong to pre-existing groups (e.g. cohorts, natural groups, etc.), and also because the assignment to different interventions may depend on criteria related to the particular setting in which the program will be implemented (Chacón-Moscoso & Shadish, 2001).
Generally speaking, the use of R-E has two main objectives. Firstly, it seeks to ensure internal validity through the randomized assignment of units to the different intervention groups, thereby favoring the comparison of groups which are similar to one another prior to program's implementation. Secondly, although not every experimental model necessarily implies randomized selection, the random sampling of units can help making the sample representative and, therefore, enhance the possibility of generalizing the results obtained. In other words, on the basis of random sampling of a well-defined context, it is possible to generalize the results obtained from the study sample, thus establishing construct validity. However, the use of random sampling in social intervention is complex, and it is difficult to choose randomly between a set of interventions, possible outcome variables that could be measured, and different time points for implementing a program, not to mention the fact that even the users and settings associated with the program are usually opportune or incidental (Cook, 1991).
Despite the advantages of achieving validity by basing an evaluation on R-E (Cook & Campbell, 1979), the instability of the real situation in which programs are applied means that practitioners face numerous problems when trying to implement a R-E (Gorard & Cook, 2007). Hence, evaluation designs rarely use a fully randomized experimental model, it being more practical to opt for a Q-E (Cook, 1991). Obviously, the fact that users are not randomly assigned to different conditions means that the different groups in a Q-E do not have the probabilistic equivalence that is characteristic of R-E; for this reason, the aim is to obtain similar groups or measures that may be comparable prior to the program's implementation (Cook et al., 1990). Thus, internal validity is considered more difficult in a Q-E than in an R-E.
When a randomized procedure is not used, a distinction is made between known and unknown assignment rules (Cook et al., 1990). The problem is that in many social and health-related intervention contexts, the groups differ in a multitude of variables, and therefore, in the absence of randomization, it is difficult to apply any intentional adjustment that renders them equivalent (Anguera, 1995).
Comparison groups. Broadly, one or more groups of users may and should be created. In the case of two groups, one is the experimental group, e.g., those participating in the program, and the other is the control or comparison group, which does not receive the treatment aim of study (Shadish et al., 2002). On one hand, an inactive comparison group would not participate in any intervention (e.g., a wait list for treatment); on the other hand, and active comparison group would participate in a different intervention (it could be the usual intervention -called treatment-as-usual-, an alternative intervention, or a placebo). In any case, the comparison group serves as a reference, allowing the effects noted in the experimental group to be compared.
In the event that different groups are formed, it is necessary to define the rule for assigning users (the principal object of internal validity), i.e. whether the process is randomized or not. If assignment is not random, there will be some cases in which the rule is known (regression discontinuity design) and others in which it is not (non-equivalent control group design or cohort design) (Cook et al., 1990).
In the event of non-randomized assignment, in which the process of assigning users is unknown, efforts must be made to create comparable groups (Chacón-Moscoso et al., 2008). For example, techniques such as matching users prior to assigning them to the program conditions may be used; nevertheless, sometimes this is not possible, or could in itself prove problematic by adding potential sources of error to the assignment process. Therefore, cohort controls (comparison to groups that move through an institution in cycles) are thought more recommendable for comparisons than non-equivalent groups, since the degree of similarity among cohort members offers better guarantees than does the use of groups created based on a criterion which may not always be properly applied. Practitioners should also strive to use multiple non-equivalent comparison groups in order to explore more threats to validity and enable the triangulation of data, thereby allowing a more precise estimation of the range of program effects.
Generally speaking, designs without a comparison group may be improved to some extent by the use of certain procedures of constructing contrasts other than with independent control groups including, in descending order of priority, regression extrapolation contrasts, normed comparison contrasts, and secondary source contrasts.
In the case of a single group, the level of the target variable or variables must be specified prior to implementing the program, so that after the intervention, practitioners are able to detect any changes to them.
Treatment/program interventions
Level of intervention. The term “intervention” refers to the level of control over the situation, which is related to the extent to which this situation is a natural or everyday one; in other words, the degree to which the users' contact with the program modifies their natural interactions with the setting (Anguera, 1995).
The concept of intervention should not be considered in dichotomous terms, i.e., whether or not there is an intervention, but as a matter of degree or level. Furthermore, any evaluation the research involves (with recording systems, user instructions, program implementation, etc.) constitutes an intervention. Levels of intervention are, as stated above, regarded as existing along a continuum (Chacón-Moscoso & Shadish, 2001). In most cases, it is possible to determine the level of intervention, with R-E presenting the highest level of intervention and Q-E, medium or low levels (Rubio-Aparicio, Marín-Martínez et al., 2018; Rubio-Aparicio, Sánchez-Meca et al., 2018).
The level of intervention changes. Some programs may initially imply a significant change in the everyday lives of participants, and therefore the design intervention would be regarded as high-medium. However, as the program begins to take effect over time, users may incorporate the intervention as a normal activity. Once could therefore say that the design intervention is now low-medium.
In all events, bearing in mind the existence of the intervention -as opposed to attempting to precisely defined its level- provides additional information about how it can be a source of variation in the data we are gathering, as well as its procedural implications.
Outcomes (results/instruments)
Types of data. The data obtained from the standardized instruments used in R-E are usually of a scalar nature, whereas Q-E normally use standardized or semi-standardized instruments that are constructed a posteriori (Shadish et al., 2005). Hence, data are either scale or ordinal. In some cases, nominal data can be found in R-E and Q-E, similar to the type often used in evaluations of low-intervention designs. The type of data is an important aspect to consider, not least because of the often lax approach toward the study of the data metric and the implications this has for the type of statistical analysis that can be performed (Holgado et al., 2010).
Data quality. When standardized instruments are used, instructions and interpretation are clearly established. Hence, data quality analysis is less relevant, as instruments are assumed to be valid and reliable (Anguera et al., 2008). Nevertheless, when semi-standardized instruments are used, data quality analyses become more necessary, mainly the testing of validity, reliability and corrections of measurement error (Holgado et al., 2009).
In summary, the validity of a measurement instrument is derived from evidence of the instrument's quality.
Rationale for the use of the chosen instruments (Anguera et al., 2008). As medium-high intervention designs refer to evaluation contexts in which the practitioner has a high degree of existing substantive knowledge, and where the level of intervention and control is also higher, standardized (or at least semi-standardized) instruments are usually available to collect data (Cano-García et al., 2017). These instruments can estimate the performance or behavior of the observed subjects on the aspect measured by the instrument (criterion-referenced test) and/or reveal inter-individual differences in the behavior or trait measured by the instrument with respect to a standard population (norm-referenced test). Handbooks or standard procedures are usually published on the use of these instruments. A semi-standardized instrument lacks some of the characteristics of the standardized ones, e.g., normalized scales to interpret scores obtained in different populations.
Types of instruments. The distinction between instruments is based on the stages involved in developing standardized tests (Crocker & Algina, 1986).
Changes in the instruments used. In program evaluations involving a low intervention, it is common to alter the measurement instrument as the program progresses. Obviously, such alterations will be minimal in high-level interventions, but the lower the intervention level, the more the design can change.
Setting (context of implementation): aspects related to context feasibility and modulator variables
The program implementation is related to both the conditions and context of implementation (setting), since implementing the same program in different contexts may yield important variations in terms of how the original design is applied (Shadish et al., 2002).
Time: number of measures and measurement points (Chacón-Moscoso et al., 2008)
As regards the measures used prior to program implementation, there has been evidence that more is better. A minimum of one pre-test measure is always needed. In the event this is not possible, a pre-test measure of independent samples can be performed or retrospective measures can be done.
In terms of post-implementation measures, one is always needed, and ideally multiple will be done to enable a comparison with a pattern of measures previously developed on the basis of substantive theory. The use of non-equivalent dependent variables is based on the same reasoning.
Adaptation of the structural dimensions of evaluation designs in relation to low-level interventions (applying observational methodology)
Given the particular characteristics of low-level intervention programs, the respective sections have been adapted to facilitate their fit with a mixed method: observational methodology.
Participants (users)
Criteria for the selection and assignment of participants. In most cases, participants are subject to a non-random selection process, one in which the idiosyncrasy of the program and the setting both play an important role, though it may very occasionally be possible to select users through a randomized procedure (Onwuegbuzie & Leech, 2007b).
The number of potential users is usually greater than that which can be accommodated given the resources available to the program. As applying a randomization procedure to these potential users would not be fair, criteria must be established to prioritize them.
Individualization of participants. Two broad aspects of low intervention programs are that of users (persons) and response levels (behaviors) (Anguera & Chacón-Moscoso, 1999). Once the number of participants or users in an evaluation study (i.e., the individuals who are the target of intervention), the criterion that prevails is the intensity. In other words, having systematic and detailed records of a few participants is better than data on a great number of participants in just one measurement occasion.
Two particular situations each require a different approach. The first is programs which are applied to groups of users though follow-up is done individually, despite the nomothetic nature of the evaluation; the second is nomothetic programs in which focal sampling is used. In recent years, the analysis of particular cases (case studies) has been gaining traction, in order to check for a common data structure; another option is the existence of a multiple-case study (Stake, 2006).
Program activities
Level of intervention/daily routines. A low level of intervention means that the treatment does not alter daily routines (Castañer et al., 2017; Portell et al., 2015; Santoyo et al., 2017). Generally, the implementation of the program involves a set of actions (perhaps even subtle ones), but these are performed without any substantial changes to the users' lifestyle or everyday context
Everyday activity implies a continuity, one in which various behaviors (both homogeneous and disparate) will emerge. It is akin to a journey through an individual's life history, and it is therefore a highly complex dynamic process (Anguera, 2001). When the aim is to evaluate programs that have been implemented as part of everyday activity, it is important to clearly define the content, that is, the everyday activity (a perceivable behavior) that we wish to evaluate.
The level of intervention changes. It is a known fact that the continuity of a program, i.e., the coherence between the different actions it involves, may require a change from a low to a medium level of intervention, or vice-versa.
Similarly, an intervention, or the logical organization of the actions the program involves, must adhere to a schedule, with specific periods assigned to the implementation of each action; it may also be advisable or even essential to establish a diachronic or synchronous plan of actions using, e.g., milestone plans, Critical Path Methods (CPM), the ROY method or the Program Evaluation and Review Technique -PERT- (Sánchez-Algarra & Anguera, 1993).
Observation instruments and data collection
Types of data. The data obtained from the instruments mentioned below are mostly nominal or categorical (Sanduvete-Chaves et al., 2009). The evaluation of programs based on observational methodology yields categorical data. Bakeman (1978) established the typical types of data of observational records (types I-IV), later adding a fifth.
Data is carried out through different free registration programs designed for the observational methodology. The most used and recommended are GSEQ (http://bakeman.gsucreate.org/), LINCE (Gabin et al., 2012) (http://observesport.com/), HOISAN (Hernández-Mendo et al., 2012) (www.menpas.com), and LINCE PLUS (Soto et al., 2019) (https://observesport.com/).
The registration yields a matrix of categorical data that marks the end of the QUAL stage and is available for the beginning of the QUAN stage, through the data quality control and its subsequent analysis.
If a rating scale is used, ordinal data is obtained (Holgado et al., 2006). In low-level intervention designs, there are very few situations in which the user, -or the practitioner conducting the evaluation- is asked to provide a response.
Data quality. Once the data has been gathered from the program user or users, the practitioner must then check to ensure a certain level of quality. In this regard, the most basic requirement is traditionally referred to as the reliability of observational data.
It is generally assumed that if at least two independent observers agree, an observational system has the consistency it requires to be valid. However, this is not necessarily the case, since the two observers may not have used the system consistently and yet still agree with one another. The same applies to intra-observer agreement (Blanco-Villaseñor, 1997).
An instrument tends to be reliable if it makes few measurement errors, and if it demonstrates stability, consistency and dependency as regards individual scores for the measured traits. Precision is another concept related to the reliability of recordings: a measure is precise if it fully represents the topographic features of the behavior in question. Precision is assessed according to the degree of concordance between an observer and a given reference norm (Blanco-Villaseñor, 1997).
Blanco-Villaseñor (1997) developed three ways of interpreting the reliability of observational data: (a) coefficients of concordance between two observers who, working independently, code behavior using the same observation instrument; (b) coefficients of agreement, calculated on the basis of correlation; and (c) the application of generalizability theory, when the practitioner wishes to integrate different sources of variation (different observers, measurement points, instruments, types of register, etc.) into a global structure.
Table 2 shows the coefficients that are usually used to measure the quality of data obtained from observational registers.
Table 2: The most widely used coefficients for measuring the quality of data obtained from observational records.
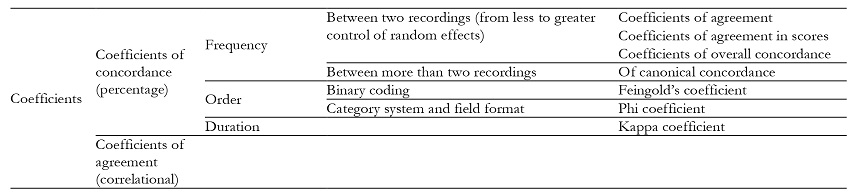
In addition to quantitative data quality controls, the notion of agreed consensus is gaining traction in observational methodology. This implies observers reaching an agreement prior to the observation (rather than measuring it afterwards, as the various quantitative coefficients are), and will always be possible provided that a recording of the behavior is available (audio if only vocal and/or verbal behavior is being evaluated, or video in other cases) and the observers discuss which category to assign to each of the behavioral units.
Rationalization for the use of the instruments. Standardized or semi-standardized instruments are not usually used in a low-level intervention program, given the idiosyncrasy of natural and/or familiar contexts. The instruments used in these situations should not only be able to address their particular features but must also be sensitive to the specific context. Although these non-standardized instruments will not have been validated with respect to a reference population, their development need to be described in great detail.
Types of instruments. Practitioners usually develop ad hoc instruments. We highlight that certain observation instruments, depending on the corresponding design, are multidimensional, such as field format combined with category system, or just field format (Anguera et al., 2008) and, consequently, multi-event sequential data, and time and event sequential data respond to this profile.
Changes in the instruments used. In low-level intervention programs, it is common to introduce changes to the design plan (duration, continuity of the different stages, contextual influencing factors, etc.). This mutability in the design has implications in the program implementation. Sometimes the modification of the instrument suffices and the level of intervention does not need to be changed. Another common situation is when the core instrument is not modified but other new instruments are introduced at different points in the process (Anguera et al., 2008).
Setting (implementation context): aspects related to feasibility and contextual modulator variables
Low-level intervention programs are usually applied in a natural context (Anguera et al., 2008). The setting is particularly relevant in the case of low-level interventions, since by their very nature, these interventions do not alter the context, which is supposed to accompany the treatment in a natural way. Nonetheless, some programs are implemented in a wide range of contexts. Furthermore, many programs may be influenced by the social context, which refers to what people are experiencing in their own lives during the program's implementation.
A diachronic perspective of time: number of measures and measurement points
One of the criteria which may be used to evaluate a program refers to the points at which data were collected. The usual scenario involves understanding how program users behave over an established period of time.
In addition to the temporal perspective on intersessional research, the diachronic perspective is focused on follow-up throughout each session, during the whole session. Many works have relied on this approach, especially Anguera et al. (2021). Here the order or sequence parameter proposed by Bakeman (1978) becomes relevant, laying the groundwork for the quantitative analyses of categorical data during the QUAN stage. Perfectly robust, it proves extremely useful in low-level intervention programs. The main diachronic analyses are the polar coordinate analysis, the sequential analysis of lags, and T-pattern detection.
The temporal aspect of data gathering means distinguishing between just one measurement occasion and monitoring/follow-up. One moment occasion will enable the situation at any given moment to be analyzed, whereas the follow-up requires a number of measurement points during the implementation of the program. Other temporal aspects are the starting point for measurements (prior to, during, or after the intervention) and the gathering period, i.e., throughout the intervention, periodic monitoring up until a certain point, continuous monitoring up until a certain point, etc. The optimal or ideal approach is to begin before the program starts, continue throughout its implementation, and conclude with medium- or long-term follow-up that will enable a rigorous analysis of the program's effects.
The various ways in which these aspects can be combined in all events and the need to adapt to the (generally limited) resources available should form the basis for the decision-making process about the data register.
Conclusions
As seen herein, any evaluation design can be systematically broken down and analyzed in relation to the same parameters. In the real world, however, it is difficult to encounter pure situations in which a single type of evaluation design unquestionably applies (e.g., Sene-Mir et al., 2020). On the contrary, the unstable and changing nature of the contexts in which program evaluation takes place makes hybrid situations frequent, thus requiring a combination of different design dimensions. Furthermore, and as noted above, these situations can and usually do change during the implementation of the program, hence the need to consider the mutability of evaluation designs.
In our view, the main variables that can influence the potential integration of low, medium and high-level intervention designs are precisely why the integration is necessary. The realities of program evaluation in various fields (healthcare, social services, sports, education, etc.), where the criteria upon which each design is based often converge, are further indication of this need.
One possible strategy could be to initially consider the most rigorous methodologies available, i.e., those associated with experimental and Q-E designs, which are rigorous in that they enable the greatest degree of intervention and/or control over the intervention in question. If low-level interventions are used, it should be done with the same level of rigor in their application.
The framework for decision-making on the design, measurement, and analysis in R-E, RCT, Q-E and N-E/observational methodology as presented herein has being laid out in two checklists on methodological quality assessment (Chacón-Moscoso et al., 2016, 2019).